Predicting Political Frames Across Policy Issues and Contexts
semanticscholar
摘要
Politically-contested issues are often discussed with different emphases by different people. This emphasis is called a frame. In this paper, we examine the performance of classifiers trained using the media frames Corpus (MFC) (Card et al., 2015); a collection of US news labelled with fifteen different frame categories. Specifically, we compare pre-trained language models (XLNet, Bert, and Roberta), fine-tuned using MFC, against results from the literature and simpler models in their ability to predict frames from text. We also test these models on a new corpus that we have derived from Australian parliamentary speeches. Our experimental results first show that the fine-tuned models significantly outperform the current best methods on MFC. We also show that the model fine-tuned on US news articles can be convincingly applied to predict policy frames in Australian parliamentary speeches, though the accuracy is significantly reduced, suggesting potential discrepancy in framing strategies and/or text usage between US News and Australian Parliamentary Speeches.
更多查看译文
AI 理解论文
溯源树
样例
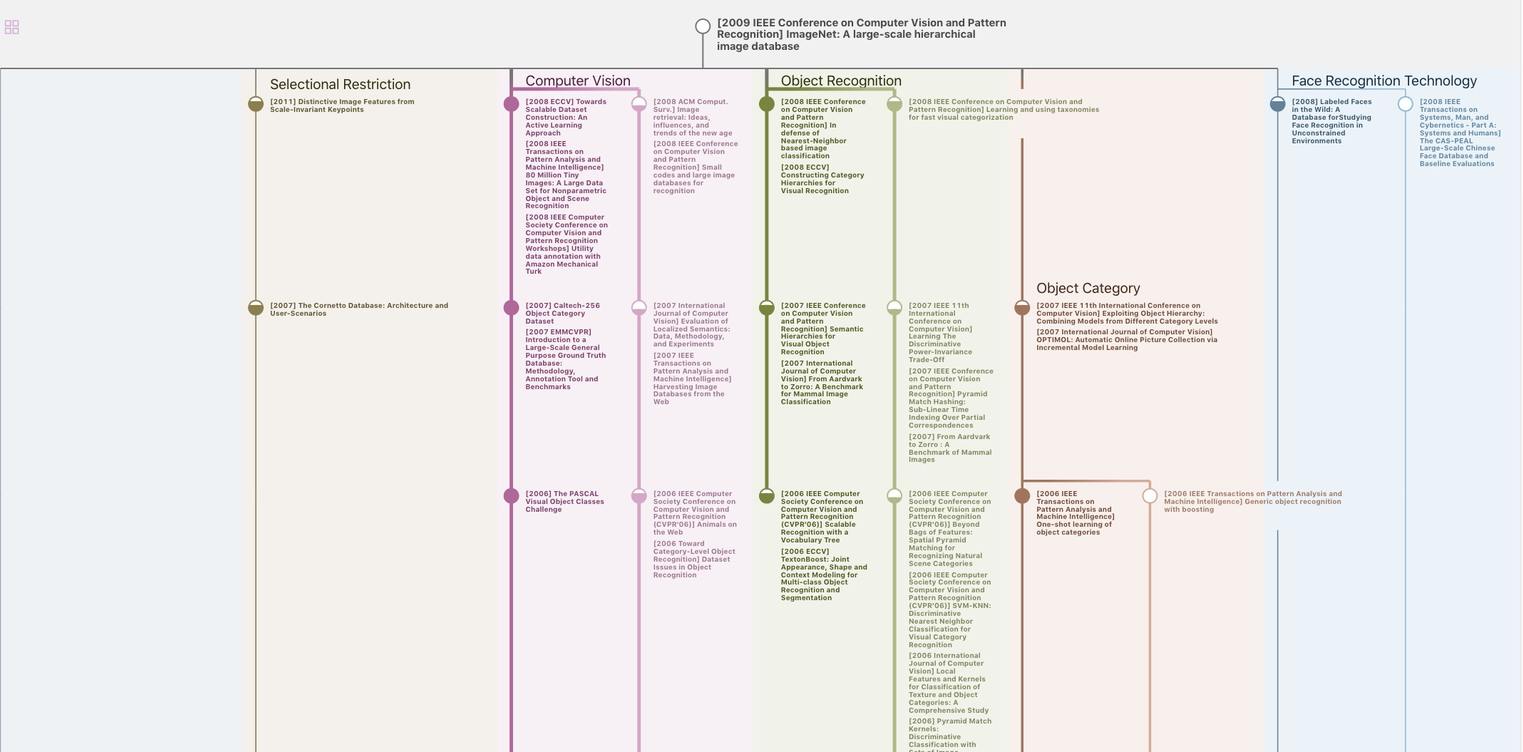
生成溯源树,研究论文发展脉络
Chat Paper
正在生成论文摘要