Waveform Level Synthesis
semanticscholar(2017)
摘要
This thesis investigates waveform-level synthesis models, which directly generate audio waveforms. In contrast, traditional feature-level synthesis models generate vocoder feature sequences, which are then converted to waveforms. This type of synthesis is limited by several factors, including the quality of the vocoder, the fixed-length analysis window and the lack of expressiveness. Waveform-level synthesis models is not limited by these factors, as vocoder is not used to generate waveforms. In this thesis, both unconditional synthesis and conditional synthesis are investigated. For unconditional waveform-level synthesis, the major challenge is to model a long history. Two models are investigated: Hierarchical Recurrent Neural Network (HRNN) and Dilated Convolutional Neural Network (DCNN). HRNN models a long history with a stack of RNNs, each operating at a different time scale, while DCNN uses stack of CNNs, each dilated to a different extent. Experiments are performed with HRNN, using both music and speech data. It is found that the model performs well in both cases, and that the structure of the network should be designed according to the time scales of different tiers. For conditional waveform-level synthesis, the major challenge is to incorporate extra information into the unconditional models. The analysis focuses on adding text information, which is more complicated and more general than other information such as music style. Two approaches are investigated: using standard text labels and using text labels generated by a neural network with attention mechanism. A new conditional synthesis model is developed, combining HRNN and standard standard text labels. Experiments are performed with both the new model and a feature-level synthesis model. The results are analyzed in both timedomain and feature-domain. It is found that the waveform-level synthesis model achieves performance comparable to the feature-level synthesis model, even with very limited tuning, and that having multiple tiers is essential to good performance.
更多查看译文
AI 理解论文
溯源树
样例
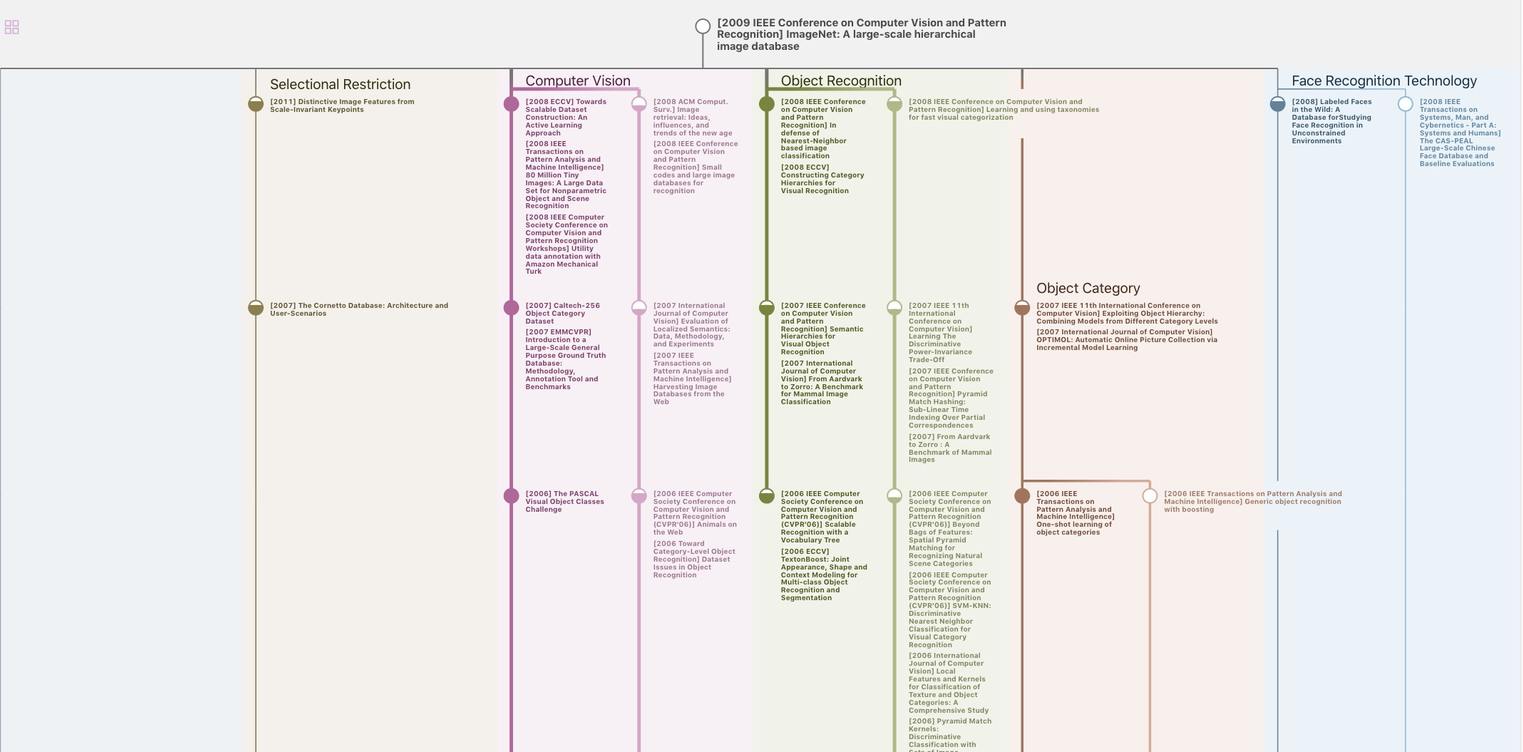
生成溯源树,研究论文发展脉络
Chat Paper
正在生成论文摘要