Simulation-Based Grasp Affordances for the PISA / IIT Softhand
semanticscholar(2014)
摘要
Recently, some research groups came out with a new generation of robotic hands that can be grouped under the name Soft Hands, as suggested in [1]. One of the main characteristic of such prototypes is to afford capabilities that are comparable to human grasping. Indeed, studying human grasping, researchers realized that a correct use and combination of compliance and underactuation can be profitably exploited for designing new robotic hands, both robust to environment interactions, and simple to control. Moreover, the adaptability of these prototypes can be profitably used to increase the range of possible executable task. For example, using soft hands, both the interaction with the object and with the environment, can be in turn used to functionally shape the hand, going beyond its nominal kinematic limits by exploiting structural softness. For this reason, in the field of grasp planning with Soft Hands, they refer to the object to grasp and to the environmental constraints with the collective name of enabling constraints [1]. Soft hands bring to a new challenge in grasp planning. Since the fact that, the low degrees of actuation is compensated exploiting the deformation of the hand through the interactions with objects and the environment, a grasp planner for Soft Hands has now to find the relative hand/object configuration able to achieve the grasp. The adaptive hand behavior, entangled with the complex dynamical interactions between the hand phalanxes and the objects – the object are not fixed during the grasp acquisition – simply cannot be modeled using conventional physics simulators like GraspIt, Open-Rave and OpenGRASP, as the complex routing of a single tendon through all the limbs is a fundamental feature which is, to be best of our knowledge, out of the above physics engines’ capabilities. For this purpose, we modeled the Pisa/IIT SoftHand [2] in ADAMS [3]. Figure 1: Comparison between (a) real and (b) simulated PISA/IIT SoftHand
更多查看译文
AI 理解论文
溯源树
样例
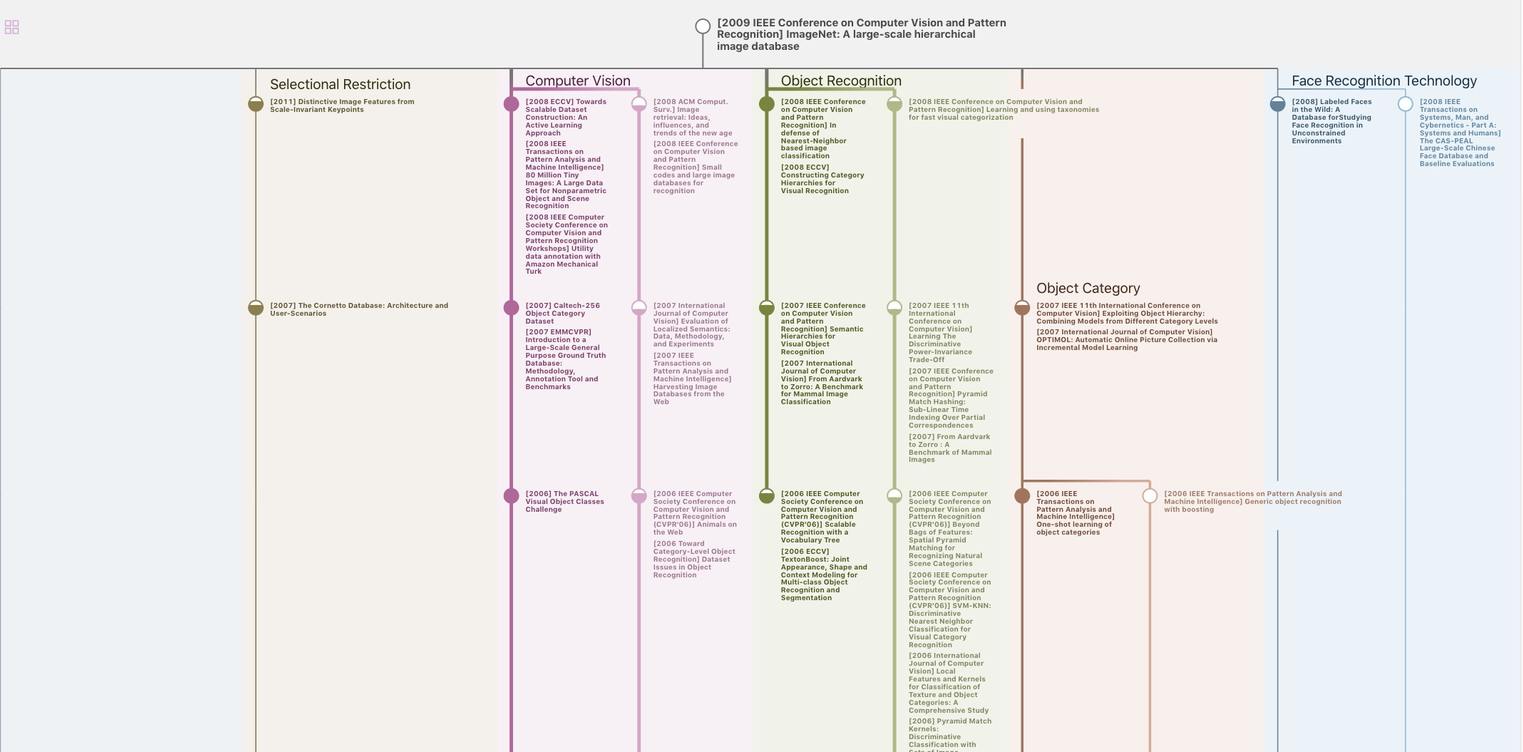
生成溯源树,研究论文发展脉络
Chat Paper
正在生成论文摘要