Training Data Voids: Novel Attacks Against NLP Content Moderation
semanticscholar(2019)
摘要
Machine learning-based content moderation systems make classification decisions by leveraging patterns learned from training data. However, patterns that are under or unrepresented in a system’s training data —which we call training data voids— cannot be learned and may be exploited by adversarial users to confuse the system. Specifically, adversarial users may creatively construct harmful content that differ from known training examples, leading to uncertain classification. Here, we call this type of attack against machine learning classifiers a novelty attack and distinguish it from a more widely known class of attacks (i.e., adversarial attacks). Additionally, we contribute a study design for exploring the extent to which novel harmful content can be constructed and for characterizing the effects of novelty on classification results in several text-based content moderation Permission to make digital or hard copies of all or part of this work for personal or classroom use is granted without fee provided that copies are not made or distributed for profit or commercial advantage and that copies bear this notice and the full citation on the first page. Copyrights for components of this work owned by others than ACM must be honored. Abstracting with credit is permitted. To copy otherwise, or republish, to post on servers or to redistribute to lists, requires prior specific permission and/or a fee. Request permissions from permissions@acm.org. CSCW ’19, Nov. 09–13, 2019, Austin, TX © 2019 Association for Computing Machinery. ACM ISBN 978-1-4503-9999-9/18/06. . . $15.00 https://doi.org/10.1145/1122445.1122456 Training Data Voids: Novel Attacks Against NLP Content Moderation CSCW ’19, Nov. 09–13, 2019, Austin, TX domains. The findings of this study are important for highlighting a potential vulnerability of machine learning-based content moderation systems and may suggest that such systems will remain limited in the near future. We propose that including human intelligence in content moderation systems may be an effective approach for mitigating potential exploitation.
更多查看译文
AI 理解论文
溯源树
样例
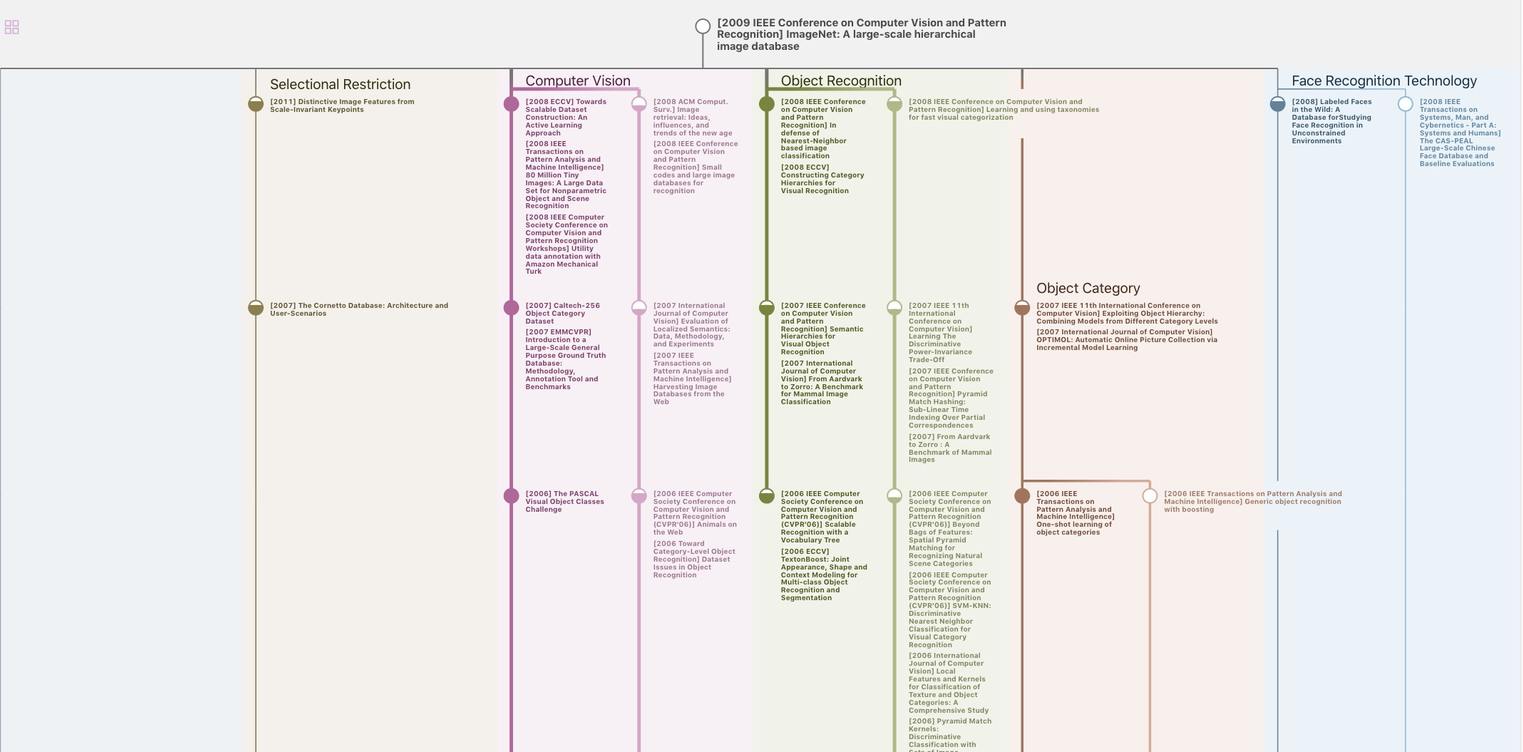
生成溯源树,研究论文发展脉络
Chat Paper
正在生成论文摘要