Project Report: Gendered Pronoun Coreference Resolution
semanticscholar(2019)
摘要
Coreference resolution is the task of identifying all mentions of entities and events in text and placing them into equivalence classes. It is a challenging and unsolved problem which is useful for numerous downstream tasks such as question answering, document summarization, and information retrieval. The goal of our project was two-fold. First, we wanted to obtain theoretical and practical knowledge of the state-of-the-art coreference resolution approaches. Second, we wanted to build our own coreference resolution system which is gender fair. Recently it has been shown (Webster et al., 2018; Zhao et al., 2018; Rudinger et al., 2018) that current state-of-the-art coreference resolution systems are biased by gender, which may impair their applicability to downstream tasks. The community has only just begun to address gender imbalance in coreference resolution accuracy, leaving significant room for improvement. However, (Webster et al., 2018) found that simple syntax-based baselines perform reasonably well on their newly introduced Gendered Ambiguous Pronouns (GAP) dataset. These syntactically motivated baselines also show improved gender balance. This indicated that neural models that incorporate syntax may hold promise. In addition, previous work found that attention-based models implicitly learn to do anaphora resolution (Vaswani et al., 2017; Voita et al., 2018), which suggests that these approaches could yield positive results in debiased coreference resolution. We explored these methods in our project, in addition to the baselines from GAP and neural baselines. We found that a reasonably simple task specific architecture added to BERT was able to outperform the baselines by a large margin. There is still room for improvement, especially in cases involving complicated narrative roles, domain-specific knowledge, or complicated syntactic constructions.
更多查看译文
AI 理解论文
溯源树
样例
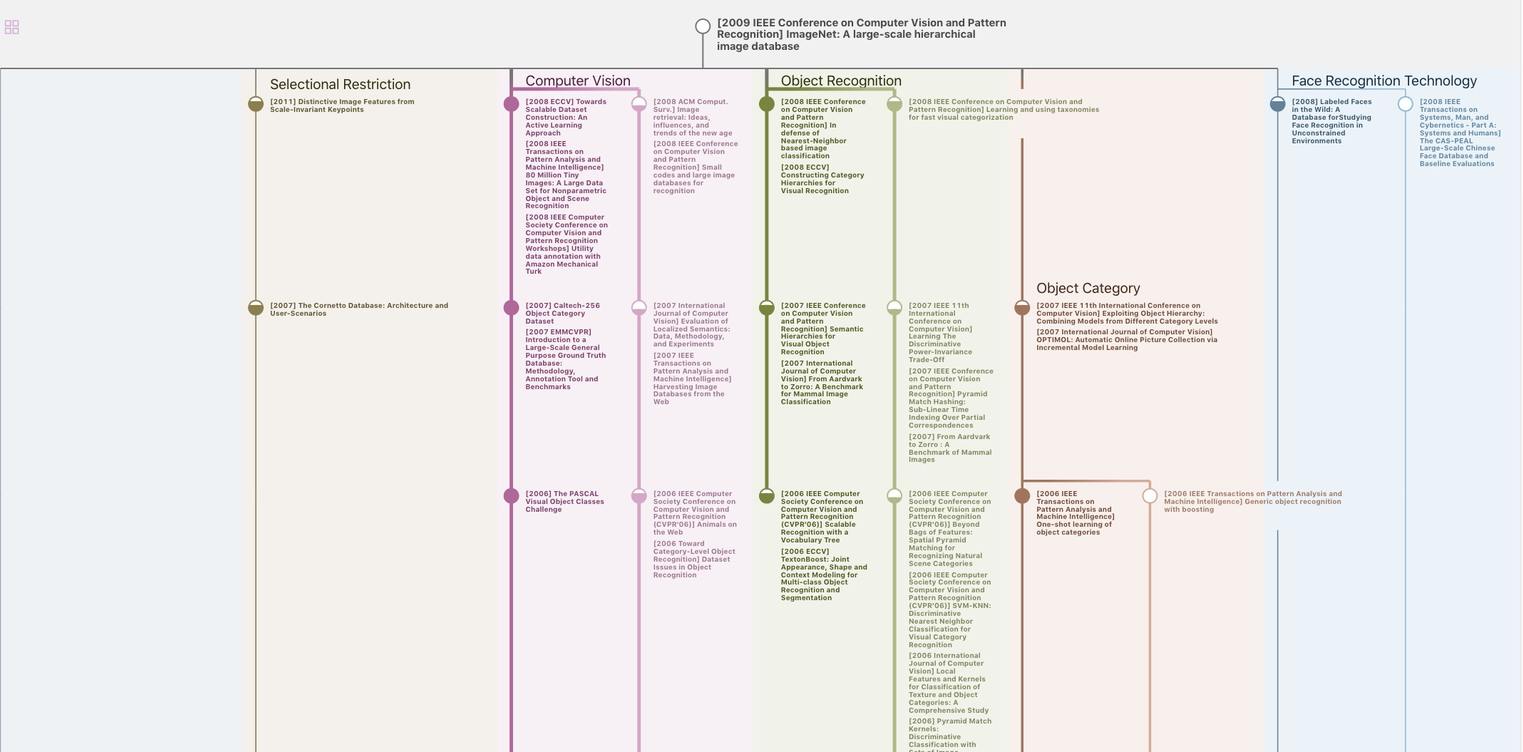
生成溯源树,研究论文发展脉络
Chat Paper
正在生成论文摘要