Informed Truthfulness in MultiTask Peer Prediction ( Working paper )
semanticscholar(2016)
摘要
The problem of peer prediction is to elicit information from agents in settings without any objective ground truth against which to score reports. Peer prediction mechanisms seek to exploit correlations between signals to align incentives with truthful reports. A long-standing concern has been the possibility of uninformative equilibria. For binary signals, a multi-task output agreement (OA) mechanism [Dasgupta and Ghosh 2013] achieves strong truthfulness, so that the truthful equilibrium strictly maximizes payoff. In this paper, we first characterize conditions on the signal distribution for which the OA mechanism remains strongly-truthful with non-binary signals. Our analysis also yields a greatly simplified proof of the earlier, binary-signal strong truthfulness result. We then introduce the multi-task 01 mechanism, which extends the OA mechanism to multiple signals and provides informed truthfulness: no strategy provides more payoff in equilibrium than truthful reporting, and truthful reporting is strictly better than any uninformed strategy (where an agent avoids the effort of obtaining a signal). The 01 mechanism is also maximally strong truthful, in that no mechanism in a broader class of mechanisms is strong truthful on more signal distributions. We then present a detail-free version of the 01 mechanism that works without any knowledge requirements on the designer while retaining -informed truthfulness.
更多查看译文
AI 理解论文
溯源树
样例
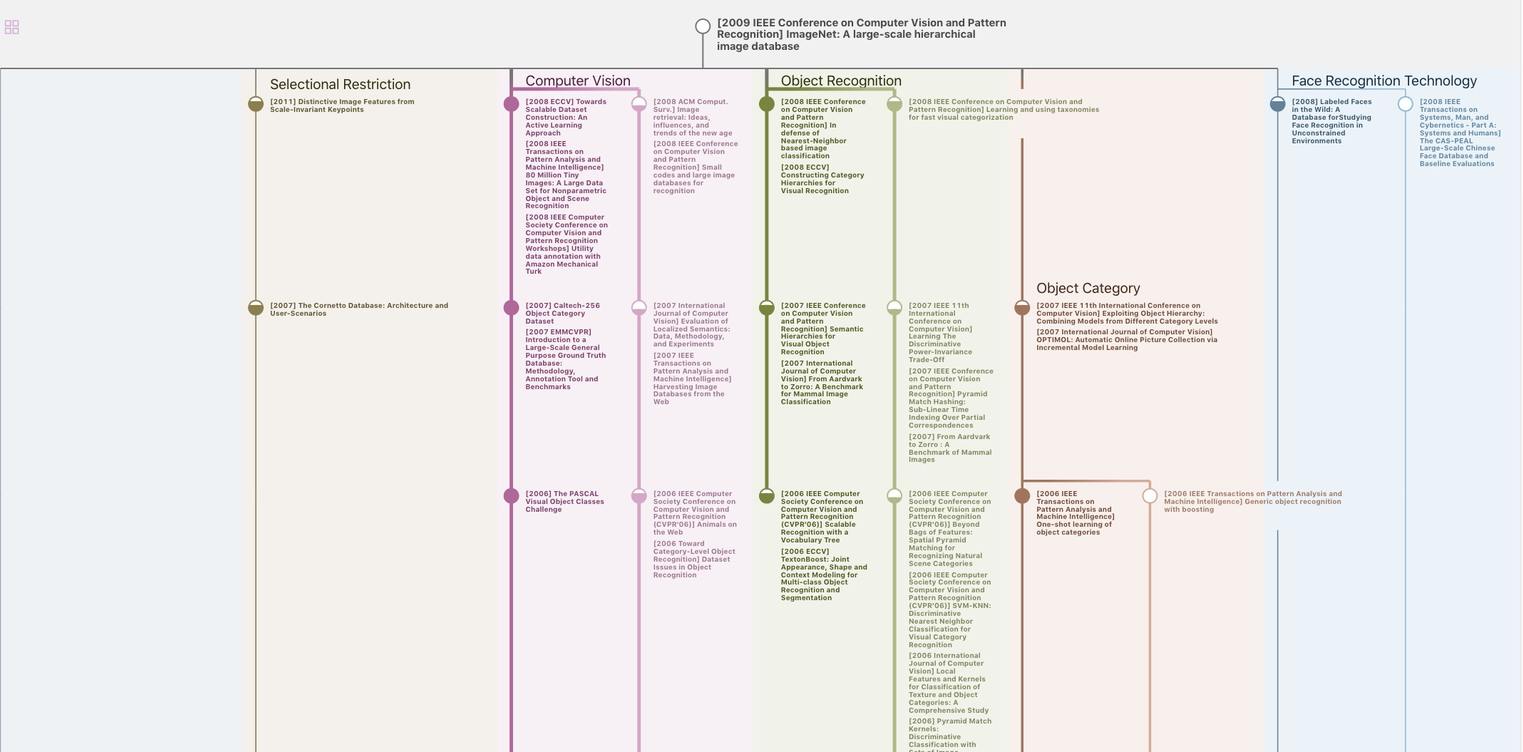
生成溯源树,研究论文发展脉络
Chat Paper
正在生成论文摘要