Decoupled Meta-Learning with Structured Latents
semanticscholar(2019)
摘要
A key aspect of human and animal intelligence is leveraging a rich variety of past experience in order to adapt quickly to acquire new skills. Meta reinforcement learning (RL) has shown promising results in enabling agents to rapidly adapt to new tasks by using prior experience and can, in principle, learn across broad distribution of tasks. However, in practice, most current methods can only meta-train on very limited task distributions. This is often due to the exploration and optimization challenges that arise when trying to quickly adapt to perform very diverse behaviors, challenges that have also plagued multi-task RL methods [8, 11, 13].
更多查看译文
AI 理解论文
溯源树
样例
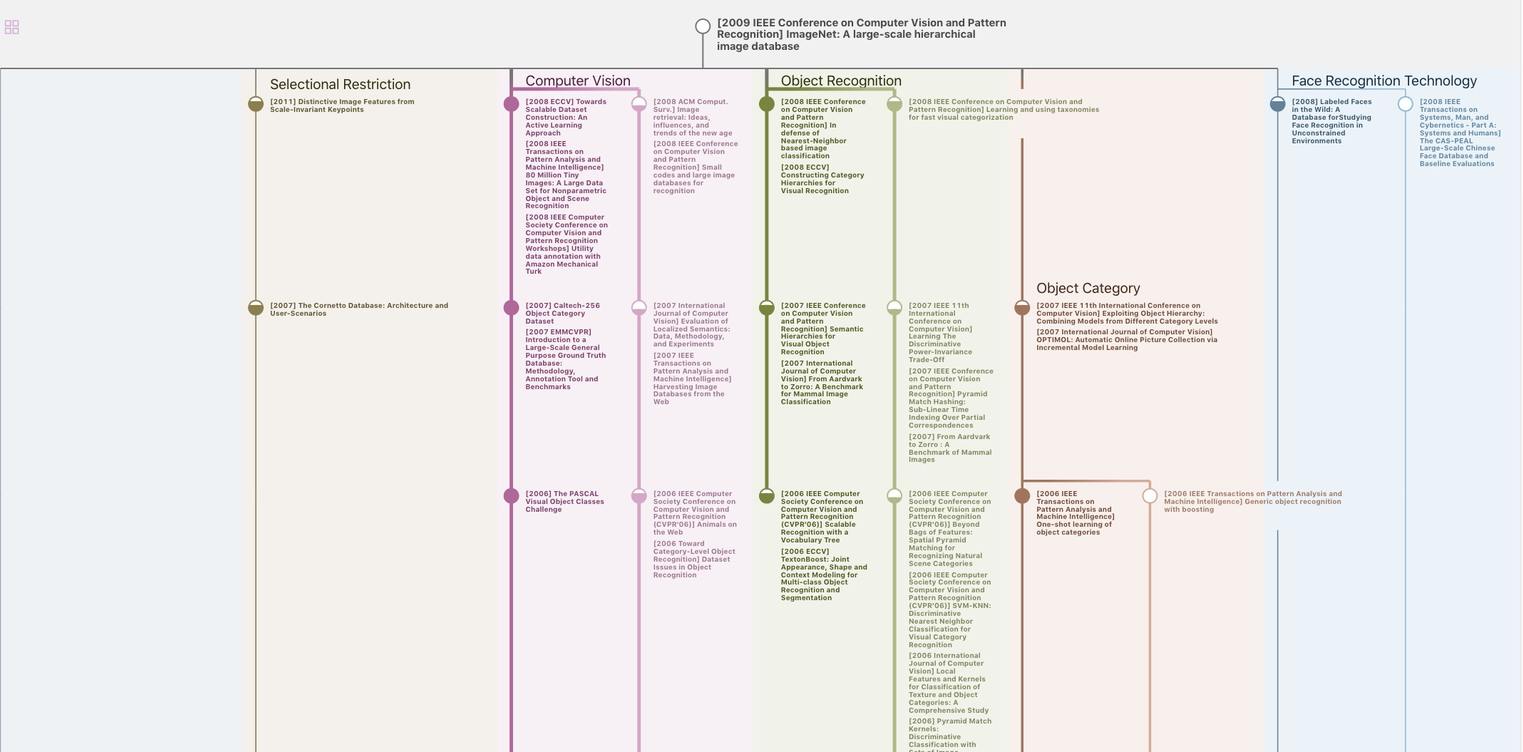
生成溯源树,研究论文发展脉络
Chat Paper
正在生成论文摘要