Digital Image Correlation as an Improved Technique for Adhesive Shear Strain Measurement in the ASTM D 5656 Test
semanticscholar(2016)
摘要
The purpose of this study was to develop and validate an improved technique for measuring adhesive shear deformation during the Thick Adherend Shear Test. The ASTM standard defining this test (D5656) requires the use of a unique extensometer system (KGR-1) that is expensive, tedious, and time consuming to calibrate and operate, and is susceptible to several experimental errors. This work focused on development of two different measurement procedures, both based on two-dimensional Digital Image Correlation (2D DIC) as potential alternatives to the KGR-1. In the first, adhesive shear data was generated from the extraction of point data from the DIC results from the region of the adherend in a manner similar to the KGR-1 (i.e. 3 points on the adherend surface). In the second, adhesive shear strains were directly extracted from the DIC data set from a defined area in the bond line. All work was performed with specimens prepared and loaded in accordance with ASTM D5656. Half the specimens were tested using the KGR-1 system, while the others were tested using DIC to obtain surface displacements and strains. The two different processing methods for the DIC data were applied during post-test analysis . The results showed both DIC processing methods to be in very good agreement with the KGR-1. Detailed analysis of the results found both DIC techniques provided significantly reduced variation in most properties compared to the KGR-1, with the DIC adherend displacement technique providing the more reliable results as well as the best comparison to the KGR-1 data. These tests also found both DIC techniques offer significant potential for time savings during adhesive shear properties testing as compared to the standard KGR-1 method. This study demonstrates the potential for the use of 2D-DIC as a more precise, and time-saving alternative to the KGR-1 extensometer method.
更多查看译文
AI 理解论文
溯源树
样例
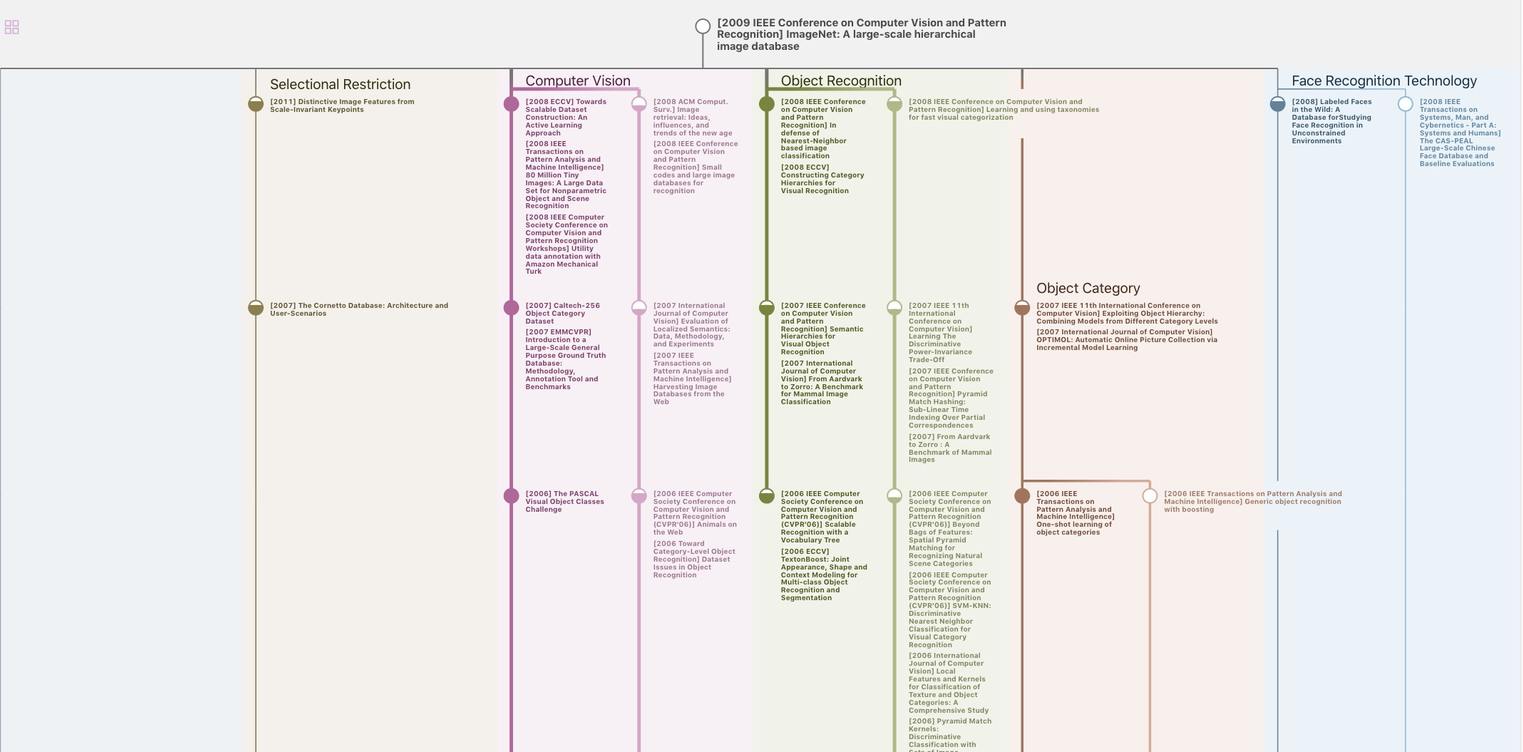
生成溯源树,研究论文发展脉络
Chat Paper
正在生成论文摘要