Xploiting model capacity by constraining within-batch features to be orthogonal
semanticscholar(2018)
摘要
Deep networks have been shown to greatly benefit from large model capacity when trained using various recent deep learning techniques. But at the same time, features in such large capacity networks have a potential to be redundant. In this work, we propose a new regularization method to exploit the given network capacity effectively. By minimizing the redundancy among in-layer filters and the correlation between in-batch features at the same time, we are able to achieve better performance with the same network architecture. Experiments with CIFAR-10/100 show that simultaneously constraining both the in-layer filters to be orthonormal and the in-batch features to be orthogonal is beneficial in efficiently utilizing the model capacity.
更多查看译文
AI 理解论文
溯源树
样例
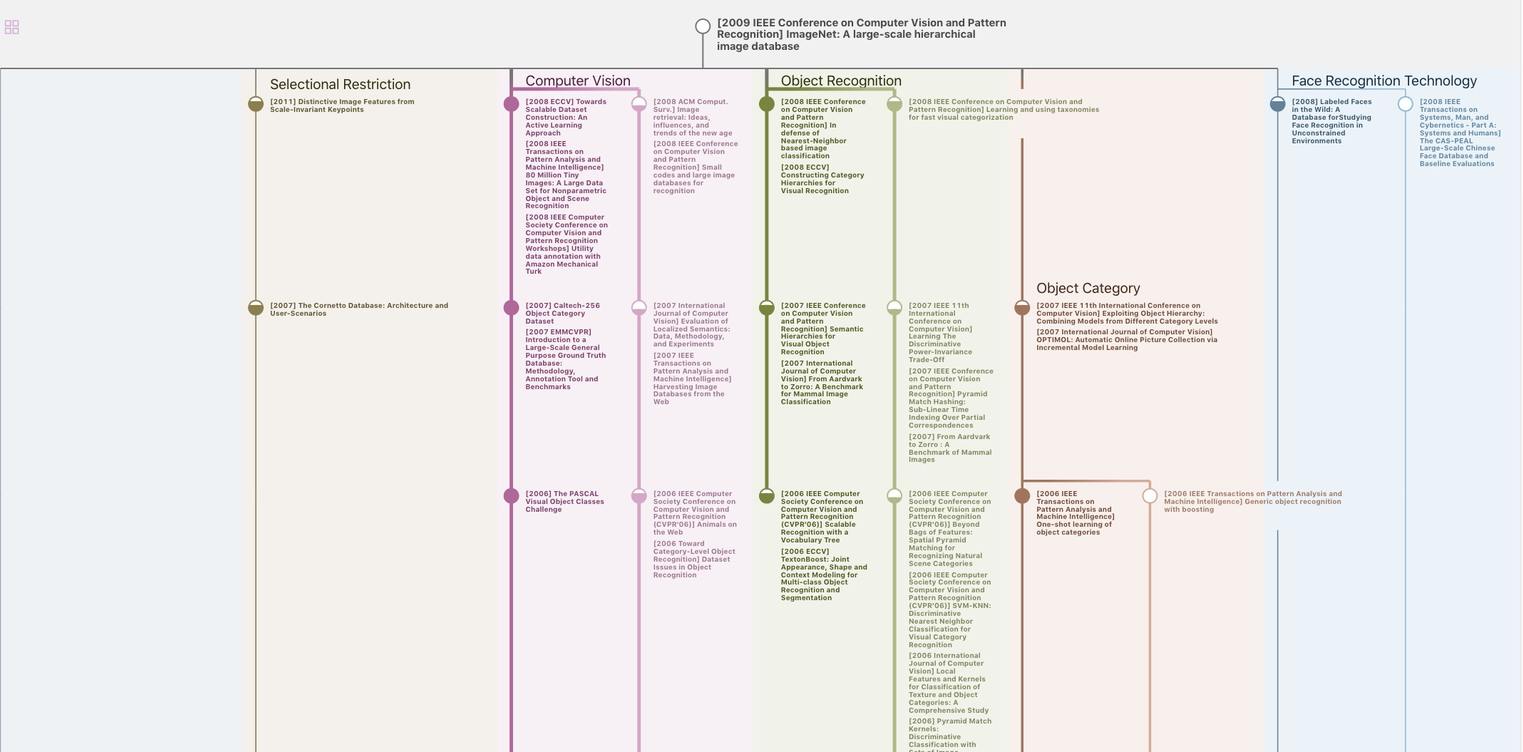
生成溯源树,研究论文发展脉络
Chat Paper
正在生成论文摘要