Müller Intrusion Detection in Unlabeled Data with Quarter-sphere Support Vector Machines
semanticscholar(2007)
摘要
The anomaly detection methods are receiving growing attention in the intrusion detection community. The two main reasons for this are their ability to handle large volumes of unlabeled data and to detect previously unknown attacks. In this contribution we investigate the application of a modern machine learning technique – one-class Support Vector Machines (SVM) – for anomaly detection in unlabeled data. We propose a novel formulation of this technique which is particularly suited for the data typical for intrusion detection systems. Our evaluation on the well-known KDDCup dataset demonstrates a significant improvement over previous formulations of the one-class SVM.
更多查看译文
AI 理解论文
溯源树
样例
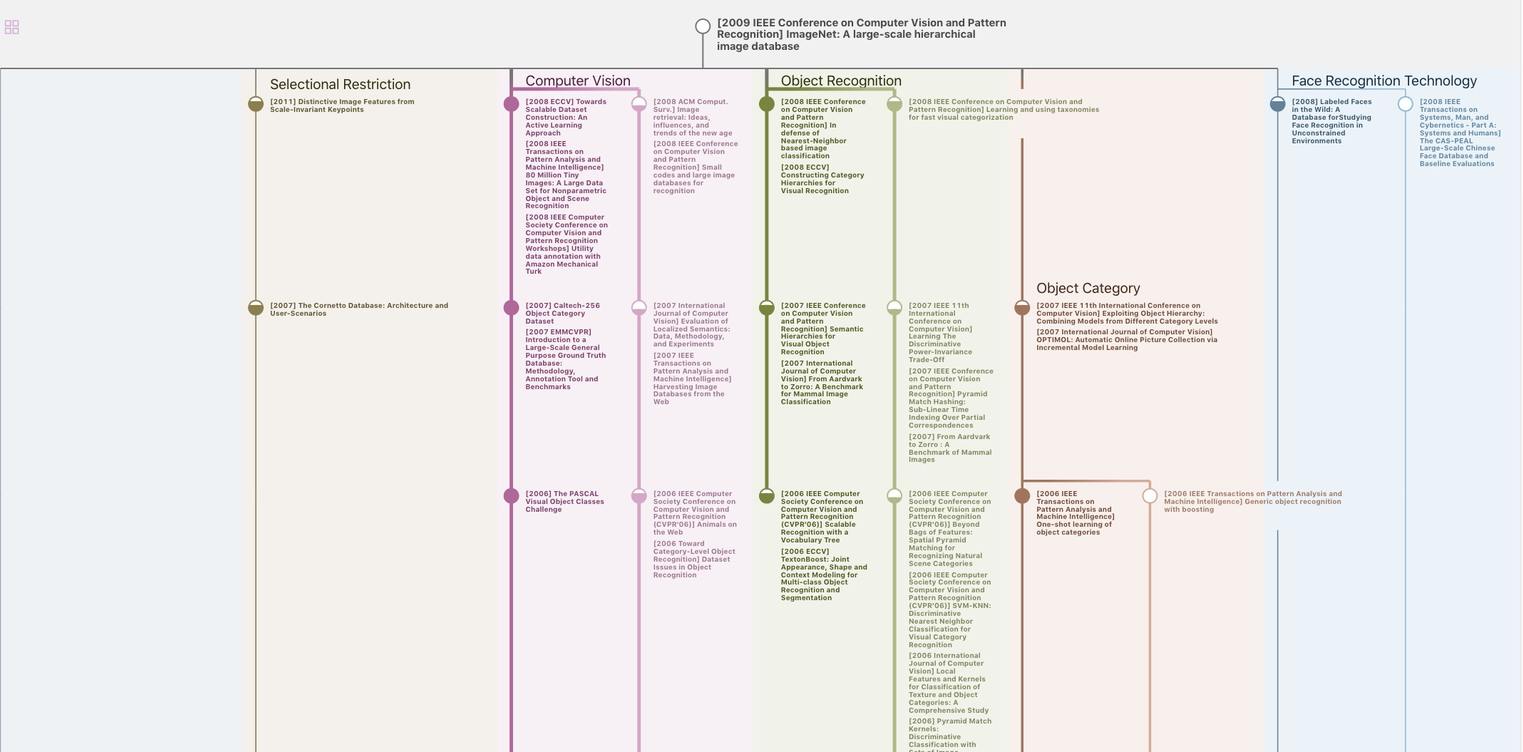
生成溯源树,研究论文发展脉络
Chat Paper
正在生成论文摘要