Interpretable Deep Learning Framework for Predicting all-cause 30-day ICU Readmissions
semanticscholar(2017)
摘要
ICU readmissions are costly and most of the early ICU readmissions in the United States are potentially avoidable. After the US Govts push towards reducing avoidable readmissions, there has been a surge in research and analyses for reducing the readmission rates. Widespread adoption of Electronic Health Records(EHRs) has made large amount of clinical data available for analysis. It has provided new opportunities to discover meaningful data-driven characteristics and implement machine learning algorithms. Sequential characteristics present in EHR data can be harnessed using state-of-the-art deep learning algorithms. While there has been rapid adoption of deep models in many domains, in Healthcare sector however, their adoption has been slow owing to lack of interpretability of these black-box models. Hence, many clinical applications still prefer simple but interpretable machine learning models. In this project, we have implemented a Knowledge-Distillation approach called Interpretable Mimic Learning for predicting 30-day ICU readmissions. Using this approach, the knowledge of deep models can be transferred to simple and interpretable models and we can combine accuracy and sequential learning of deep models with interpretability of simple models. Keywords—ICU Readmissions, Deep Learning model, Interpretability, RNN, LSTM.
更多查看译文
AI 理解论文
溯源树
样例
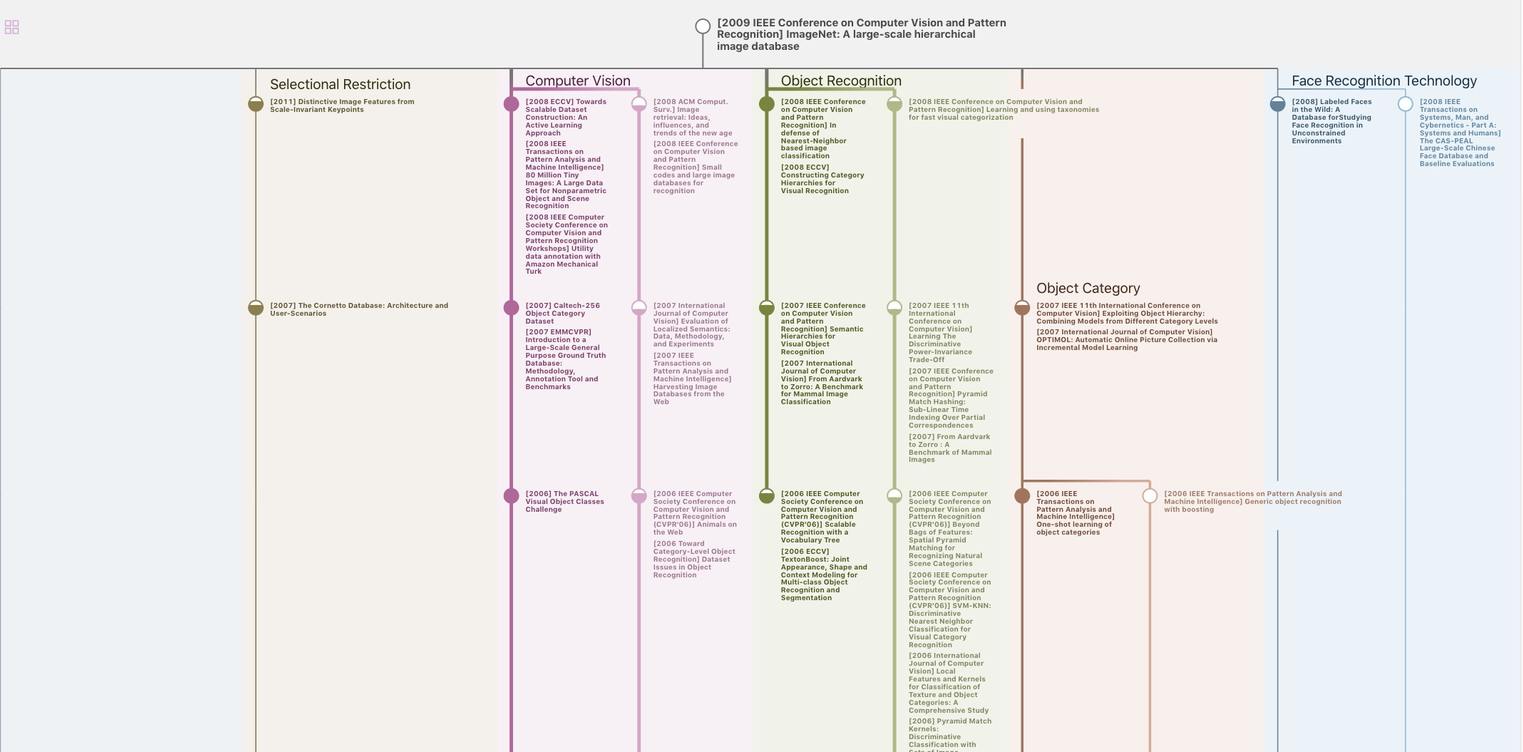
生成溯源树,研究论文发展脉络
Chat Paper
正在生成论文摘要