When Bayesian Model Selection meets the Scientific Method
semanticscholar(2018)
摘要
The scientific method includes the process of testing theories with data in order to reject incorrect ones. I was asked by a PhD student for my opinion of a recent paper which attempted to evaluate the adequacy of competing pharmacokinetic models for the analysis of MR data [2]. Based upon papers I have read over the last three decades, I believe that its description of Bayesian Model Selection could well be a representative example of the methodology from the literature. However, for reasons explained below, I do not believe this approach to be either quantitatively valid or capable of usefully addresing such an issue. As on several previous occasisons, a verbal explanation to the student simply could not do my numerous comments justice, so this time I decided to write them down. The general approach taken is based upon, and consistent with, other documents found on our web pages (see for example [3]). The conclusions which follow may appear quite contentious, even inconvenient, but I don’t expect anyone to simply take my word on this. It should be possible for any mathematically literate reader to independently confirm these criticisms with a little thought. I will modify this document subject to any constructive feedback. Conventional Approach For a set of mutually exclusive data generators mi and associated parameters ai we can define the joint probability of the data d and the model parameters using Bayes Theorem as p(mi, ai|d, I) = p(mi, ai|I)p(d|mi, ai, I) p(d|I) where I is all prior information including the co-hort used to define set of example models (but excluding the current data d). In practical use p(d|mi, ai, I) is taken to be the Likelihood for the data d given the model parameters ai. This expression is sometimes optimised as a process called MAP estimation, and similar forms (a prior multiplying a Likelihood), motivated for model selection. However, this “probability” is not directly suitable for this, or indeed for model selection, as it is really a density and depends upon our specific choice of parametric description (ai). In order to compute the probability of the model independent of the parameter choice we must integrate over a meaningful interval of the parameters. In [2] the common argument is made to use all possible values of the parameter. Then p(mi|d, I) ∝ p(mi|I) ∫ p(ai|mi, I)p(d|mi, ai, I) dai (1) where we have discarded the normalisation and used the result p(mi, ai|I) = p(mi|I)p(ai|mi, I) Where p(mi|I) is a scale factor which is assumed in advance (prior) and p(ai|mi, I) is the distribution of model parameters sampled from data in the specified sample cohort. This theory is very general, no specification is given for particular distributions, but what is known is the definitions of what we mean by p (Kolmogorov’s axioms) and that terms in the expression must be a function of the parameters (and only those parameters) specified. We will also require for science that our probabilities reflect measurable distributions [3]. Where the method requires modification in a way that these requirements are not met, we will say below that it contradicts the theory. You can of course seek to extend the theory to incorporate additional terms or definitions, but can no longer expect to be able to appeal to the original “mathematically rigorous” derivation as justification for the modified approach. Quantitative Application We can imagine, and people often develop, all sorts of ways to construct the terms in equation (1) for real world problems. However, making an analysis scientifically useful requires a quantitative approach. Using equation (1) quantitatively requires us to attend to several issues. Firstly the Likelihood function needs to be appropriate to the data measurement. Secondly (and also third), the prior distributions p(a|mi, I) and the scalings p(mi|I) need
更多查看译文
AI 理解论文
溯源树
样例
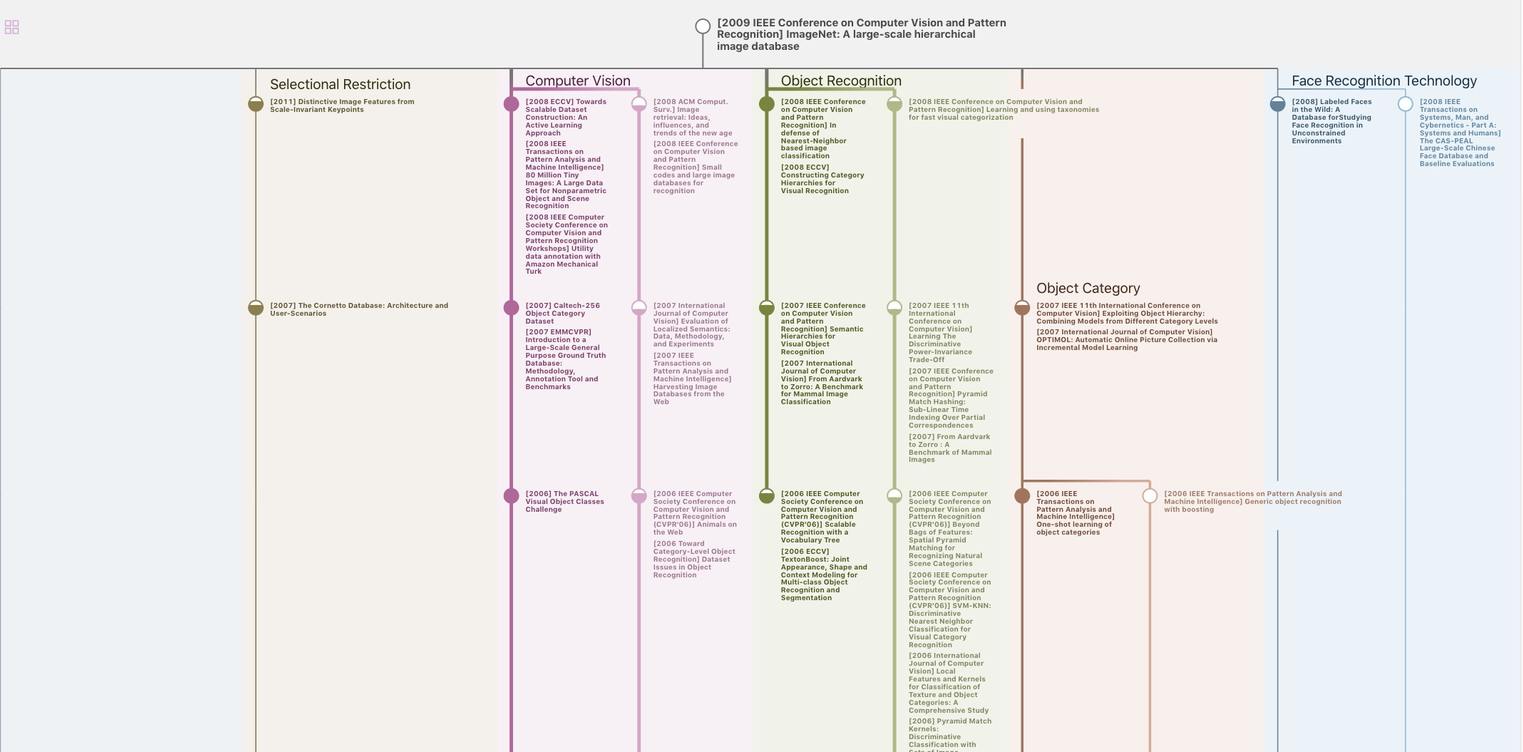
生成溯源树,研究论文发展脉络
Chat Paper
正在生成论文摘要