Artificial Intelligence Applications and Innovations
IFIP Advances in Information and Communication Technology(2019)
摘要
s of Invited Talks Learning from Electronic Health Records: From Temporal Abstractions to Time Series Interpretability Panagiotis Papapetrou Department of Computer and Systems Sciences, Stockholm University panagiotis@dsv.su.se Abstract. The first part of the talk will focus on data mining methods for learning from Electronic Health Records (EHRs), which are typically perceived as big and complex patient data sources. On them, scientists strive to perform predictions on patients’ progress, to understand and predict response to therapy, to detect adverse drug effects, and many other learning tasks. Medical researchers are also interested in learning from cohorts of population-based studies and of experiments. Learning tasks include the identification of disease predictors that can lead to new diagnostic tests and the acquisition of insights on interventions. The talk will elaborate on data sources, methods, and case studies in medical mining. The second part of the talk will tackle the issue of interpretability and explainability of opaque machine learning models, with focus on time series classification. Time series classification has received great attention over the past decade with a wide range of methods focusing on predictive performance by exploiting various types of temporal features. Nonetheless, little emphasis has been placed on interpretability and explainability. This talk will formulate the novel problem of explainable time series tweaking, where, given a time series and an opaque classifier that provides a particular classification decision for the time series, the objective is to find the minimum number of changes to be performed to the given time series so that the classifier changes its decision to another class. Moreover, it will be shown that the problem is NP-hard. Two instantiations of the problem will be presented. The classifier under investigation will be the random shapelet forest classifier. Moreover, two algorithmic solutions for the two problem instantiations will be presented along with simple optimizations, as well as a baseline solution using the nearest neighbor classifier. The first part of the talk will focus on data mining methods for learning from Electronic Health Records (EHRs), which are typically perceived as big and complex patient data sources. On them, scientists strive to perform predictions on patients’ progress, to understand and predict response to therapy, to detect adverse drug effects, and many other learning tasks. Medical researchers are also interested in learning from cohorts of population-based studies and of experiments. Learning tasks include the identification of disease predictors that can lead to new diagnostic tests and the acquisition of insights on interventions. The talk will elaborate on data sources, methods, and case studies in medical mining. The second part of the talk will tackle the issue of interpretability and explainability of opaque machine learning models, with focus on time series classification. Time series classification has received great attention over the past decade with a wide range of methods focusing on predictive performance by exploiting various types of temporal features. Nonetheless, little emphasis has been placed on interpretability and explainability. This talk will formulate the novel problem of explainable time series tweaking, where, given a time series and an opaque classifier that provides a particular classification decision for the time series, the objective is to find the minimum number of changes to be performed to the given time series so that the classifier changes its decision to another class. Moreover, it will be shown that the problem is NP-hard. Two instantiations of the problem will be presented. The classifier under investigation will be the random shapelet forest classifier. Moreover, two algorithmic solutions for the two problem instantiations will be presented along with simple optimizations, as well as a baseline solution using the nearest neighbor classifier. Empirical Approach: How to Get Fast, Interpretable Deep Learning
更多查看译文
AI 理解论文
溯源树
样例
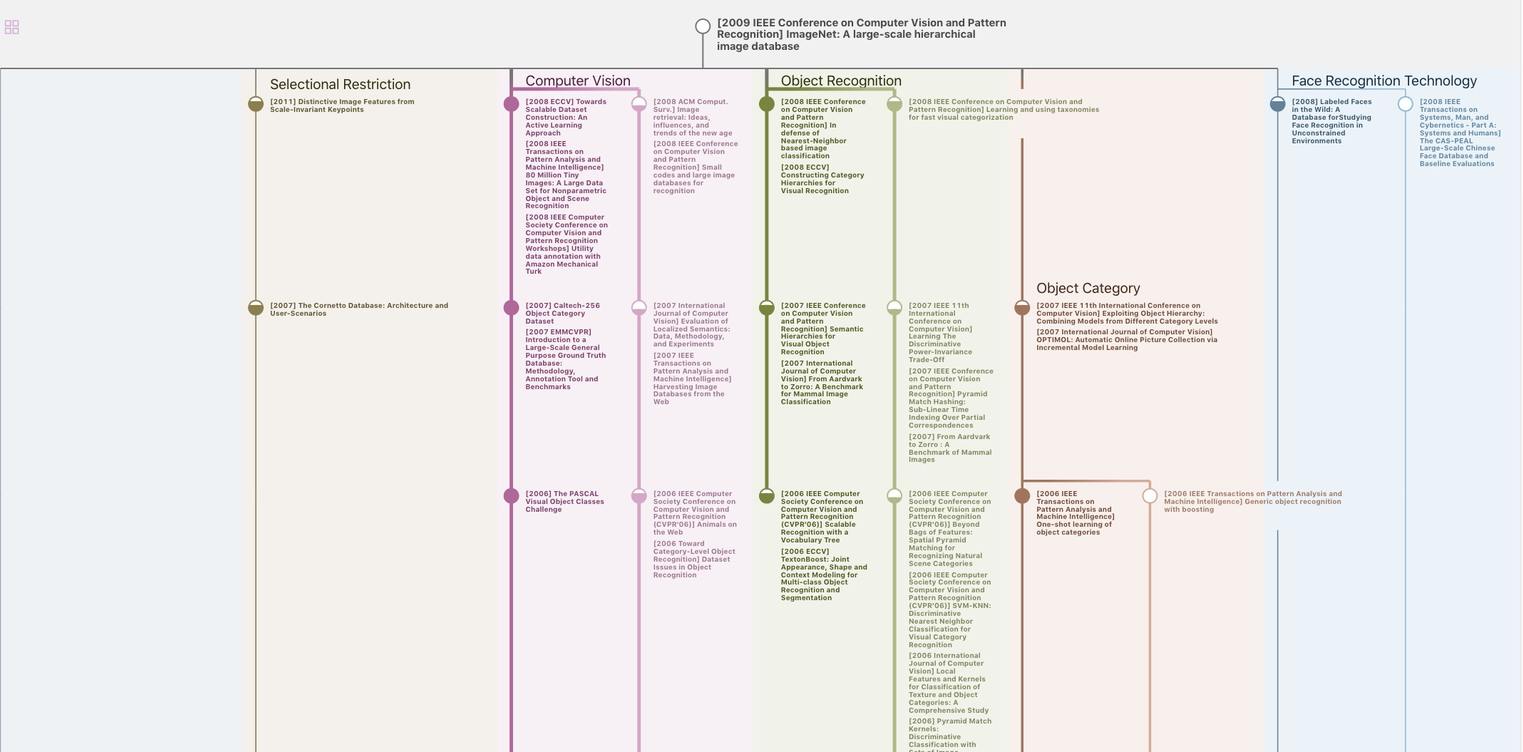
生成溯源树,研究论文发展脉络
Chat Paper
正在生成论文摘要