Learning to Predict the Presence of Nodes in Anonymized Graphs
semanticscholar(2014)
摘要
Introduction We address the problem of learning presence models of nodes in anonymized graphs. Specifically given two graphs, a “known” graph G1 and an anonymized graph G2, we want to learn a classification model that predicts the presence of nodes in G2. Solving this problem does not require solving the nodecorrespondence problem across graphs. All that it requires is the computation of the probability: P(v ∈ G2 | v ∈ G1), ∀v ∈ G1. This problem is a weaker form of the re-identification (a.k.a. de-anonymization) problem [1][2][3]. However, if we can accurately solve this problem, then inferences can be made that could reveal too much information about the anonymized network. This problem has a wide range of applications from network surveillance, product promotion and marketing, healthcare and related services to name a few. For example, consider a known co-authorship graph, which includes Albert-László Barabási, Mark Newman, and Bernardo Huberman; and an anonymized graph where based on a classification model the probabilities of their presences is high, then one can infer that the anonymized graph is about physicists interested in network science and complex systems.
更多查看译文
AI 理解论文
溯源树
样例
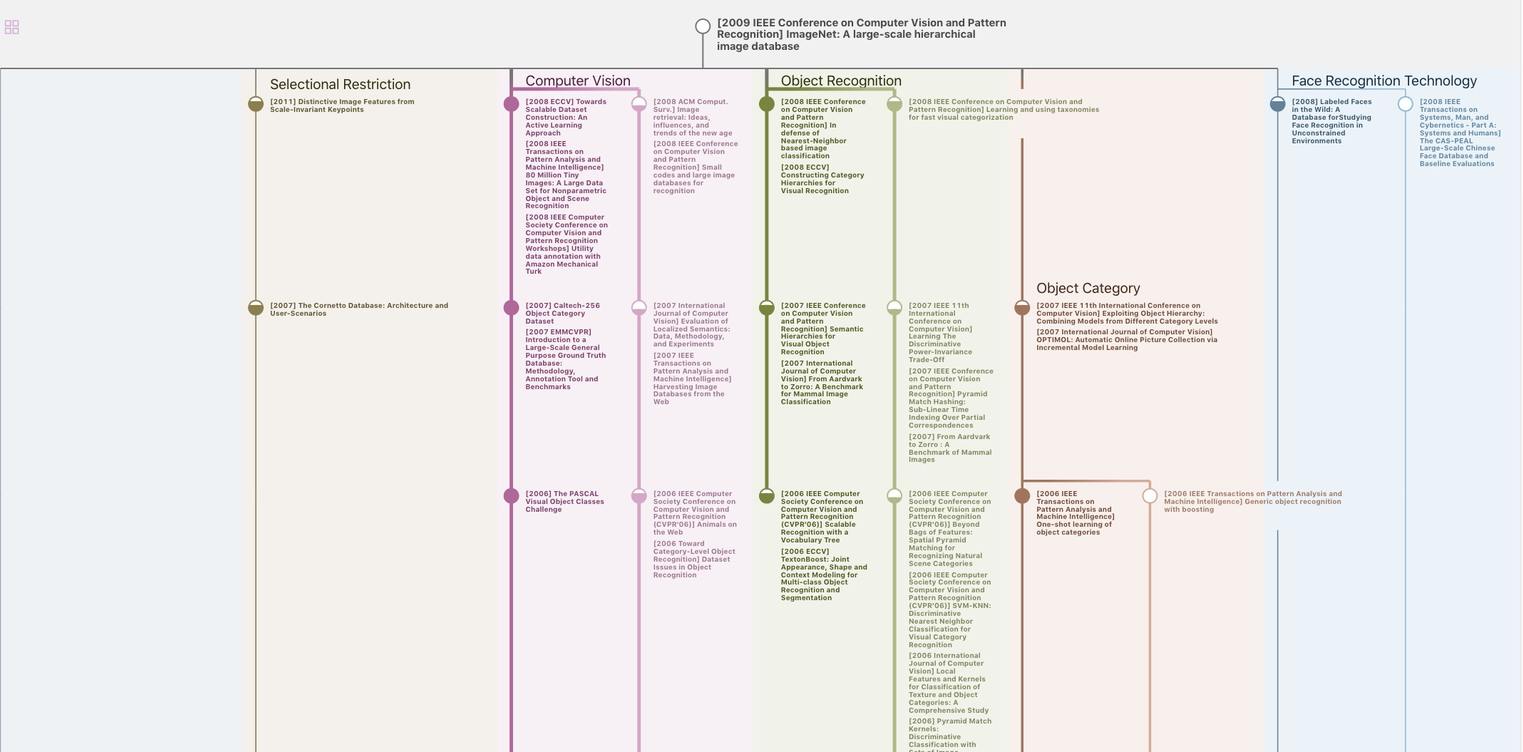
生成溯源树,研究论文发展脉络
Chat Paper
正在生成论文摘要