KOSNet : A Unified Keypoint , Orientation and Scale Network for Probabilistic 6 D Pose Estimation
semanticscholar(2019)
摘要
We propose a novel method using a Convolutional Neural Network (CNN) for probabilistic 6D object pose estimation from color images. Unlike other methods that compute only one data point as the output, our network returns the information necessary to estimate the full probability distributions of 6D object poses. This not only captures the ambiguity of object appearance in the image in a principled manner, but also enables the results to be fused with other sensing modalities using well-established probabilistic inference techniques. One of the main challenges is to provide probabilistic ground truth labels for training the network. To this end, we introduce a way to approximate uncertainties of object poses related to rotational symmetry, occlusion, and how distinct an object is from the background. We demonstrate the unique capability of our network on both fully and partially rotationally symmetric objects while achieving comparable performance with a stateof-the-art method on publicly available datasets.
更多查看译文
AI 理解论文
溯源树
样例
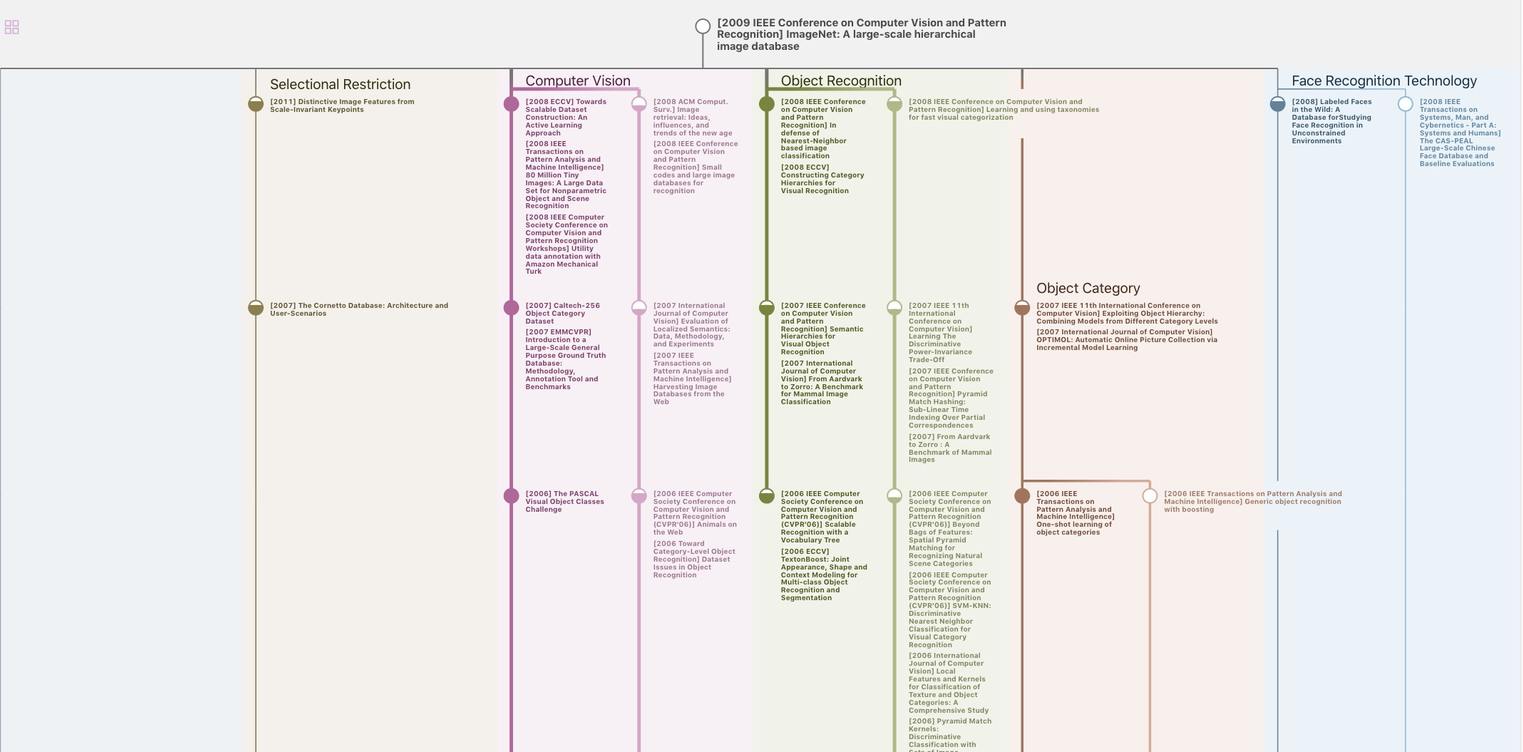
生成溯源树,研究论文发展脉络
Chat Paper
正在生成论文摘要