Classify contaminated blood tests: a neural approach
semanticscholar(2018)
摘要
Automated laboratories analyze thousands of blood tests every day. Some samples can become contaminated by improper collection, producing incorrect results. Identification of such samples is critical and requires a labor intensive expert review. In our previous work, we demonstrated a useful machine learning approach to automated detection, but a severe class imbalance in the dataset led to unstable results. Using a modified data augmentation strategy and an attentional neural architecture, we show significant improvement over previously published methods in all binary classification metrics, with an absolute F1-score improvement of 13%. This work represents the state-of-the-art in automated contamination detection and demonstrates that a machine learning pipeline based on our neural architecture can perform on par with human experts.
更多查看译文
AI 理解论文
溯源树
样例
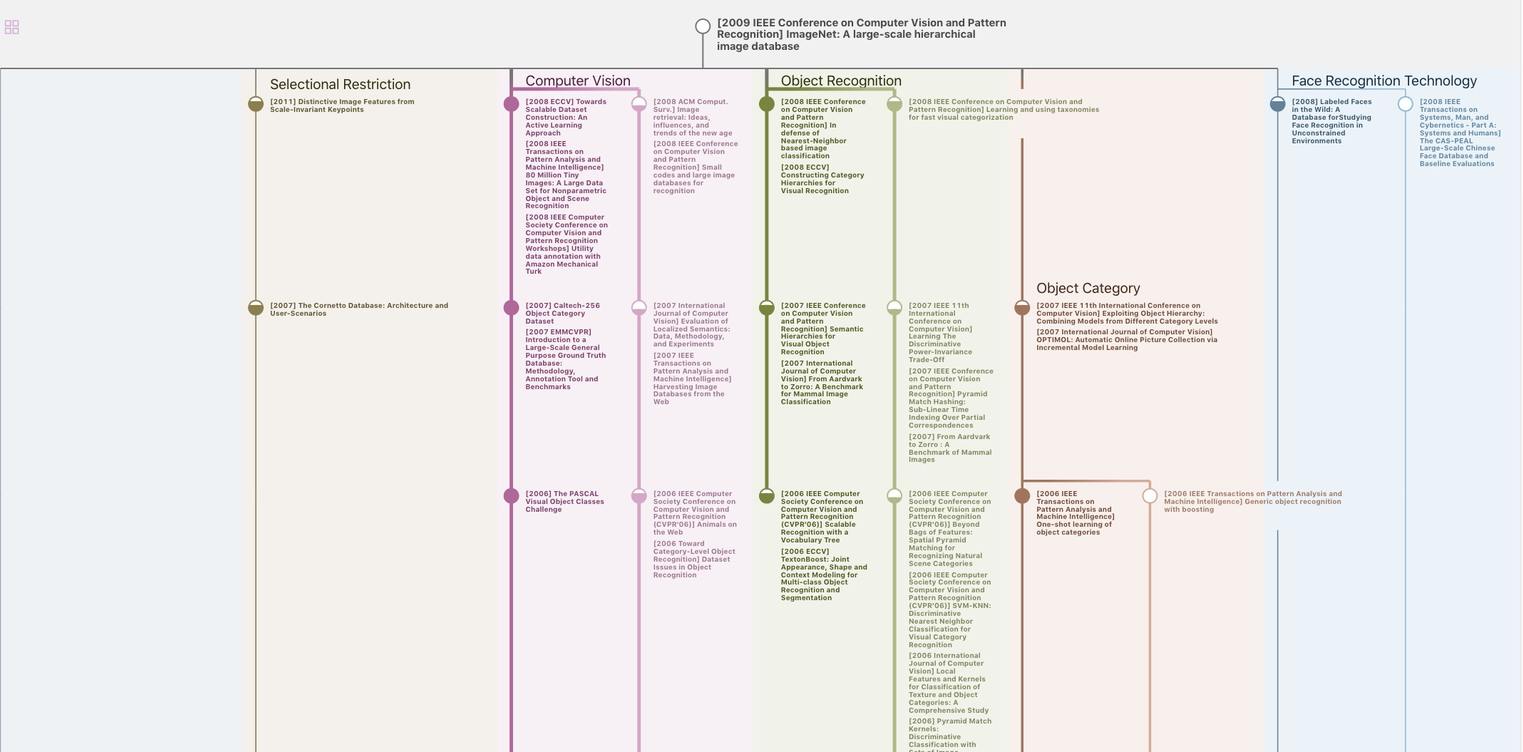
生成溯源树,研究论文发展脉络
Chat Paper
正在生成论文摘要