Analyzing and modeling groups
user-5efd71244c775ed682ed8a03(2015)
摘要
Web search engines utilize behavioral signals to develop search experiences tailored to individual users. To be effective, such personalization depends on access to sufficient information about each user’s interests and intentions. For new users or queries, profile information may be sparse or non-existent. To handle these cases, and perhaps also improve personalization for the others, search engines can employ signals from those similar to the current user along one or more dimensions, ie, those in the same cohort. In this chapter we describe a characterization and evaluation of the use of such cohort modeling to enhance search personalization. We experiment with three predefined cohorts-topic, location, and top-level domain preference independently and in combination, and also evaluate methods to learn cohorts dynamically. We show via extensive experimentation with large-scale logs from a commercial search engine that by leveraging cohort behavior we can attain significant relevance improvements when combined with a production ranker that uses similar classes of personalization signal but at the individual searcher level. Additional experiments show that our gains can be extended when we dynamically learn cohorts and target classes of ambiguous or unseen queries.
更多查看译文
AI 理解论文
溯源树
样例
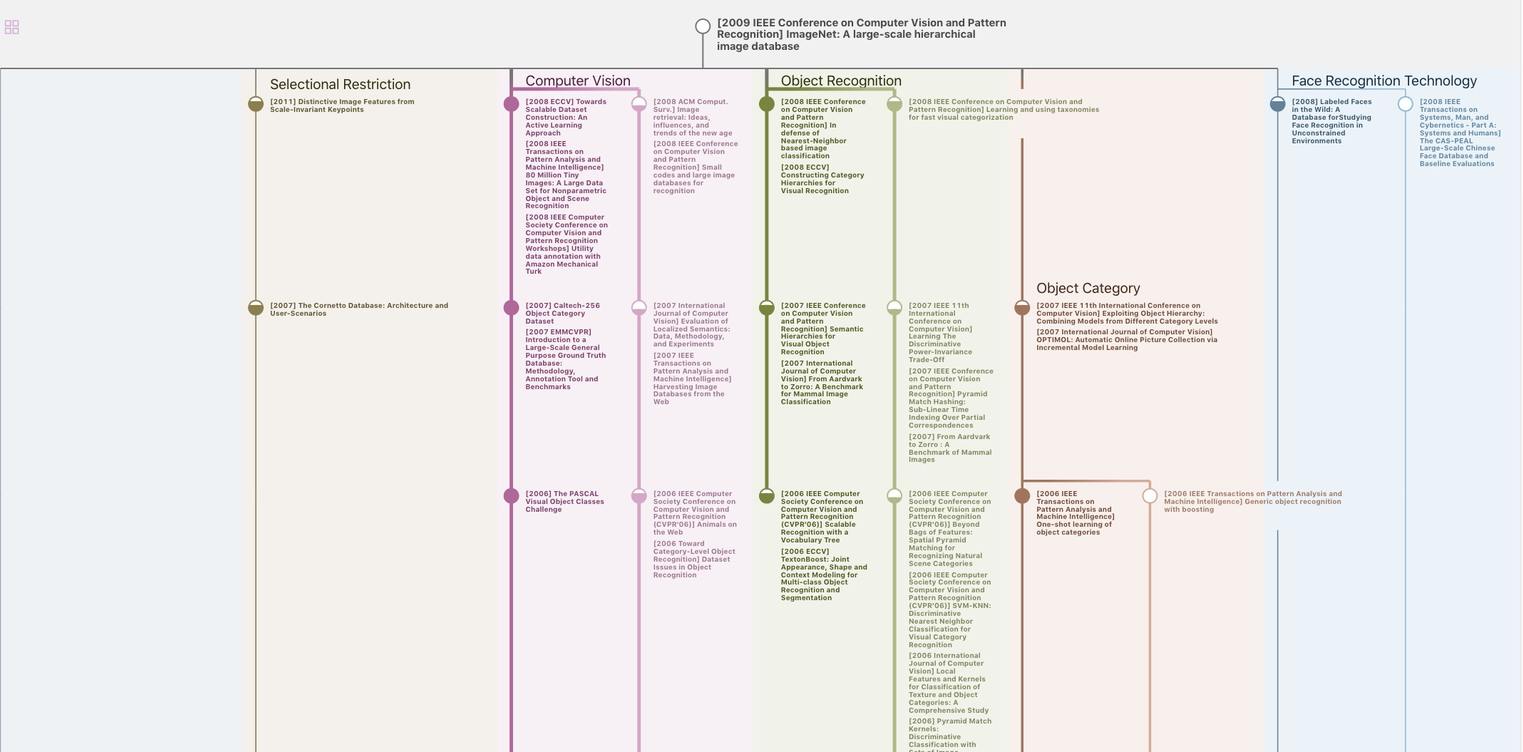
生成溯源树,研究论文发展脉络
Chat Paper
正在生成论文摘要