City Scale Next Place Prediction from Sparse Data through Similar Strangers
semanticscholar(2017)
摘要
Various methods predicting next locations of people have shown to be very successful under certain data conditions. Among other similarly sparse datasets, the nature of Call Detail Records (CDRs) limits the potentials of some approaches due to sparsity. Location information of users is mostly unavailable except when users initiate or receive phone activity. In this paper, we show that when information of travelers and their contacts is obtained from CDRs, alternative approaches become more effective. We tackle the problem of data sparsity in time stamped location data with the goal of improving accuracy of next location prediction in the case of CDRs. We propose a probabilistic framework for human mobility prediction in sparse datasets and introduce data of multiple phones for a richer dataset leading to better performance. The proposed method observes an increase in accuracy of the prediction by coupling with the data of most similar strangers, stranger in a sense that a social link doesn’t necessarily exist in the social graph. The dataset used in this study has 300 thousand users with a duration of a month. ACM Reference format: Fahad Alhasoun, May Alhazzani, Faisal Aleissa, Riyadh Alnasser, and Marta González. 2017. City Scale Next Place Prediction from Sparse Data through Similar Strangers. In Proceedings of ACM KDD Workshop, Halifax, Canada, August 14, 2017 (UrbComp’17), 8 pages. https://doi.org/
更多查看译文
AI 理解论文
溯源树
样例
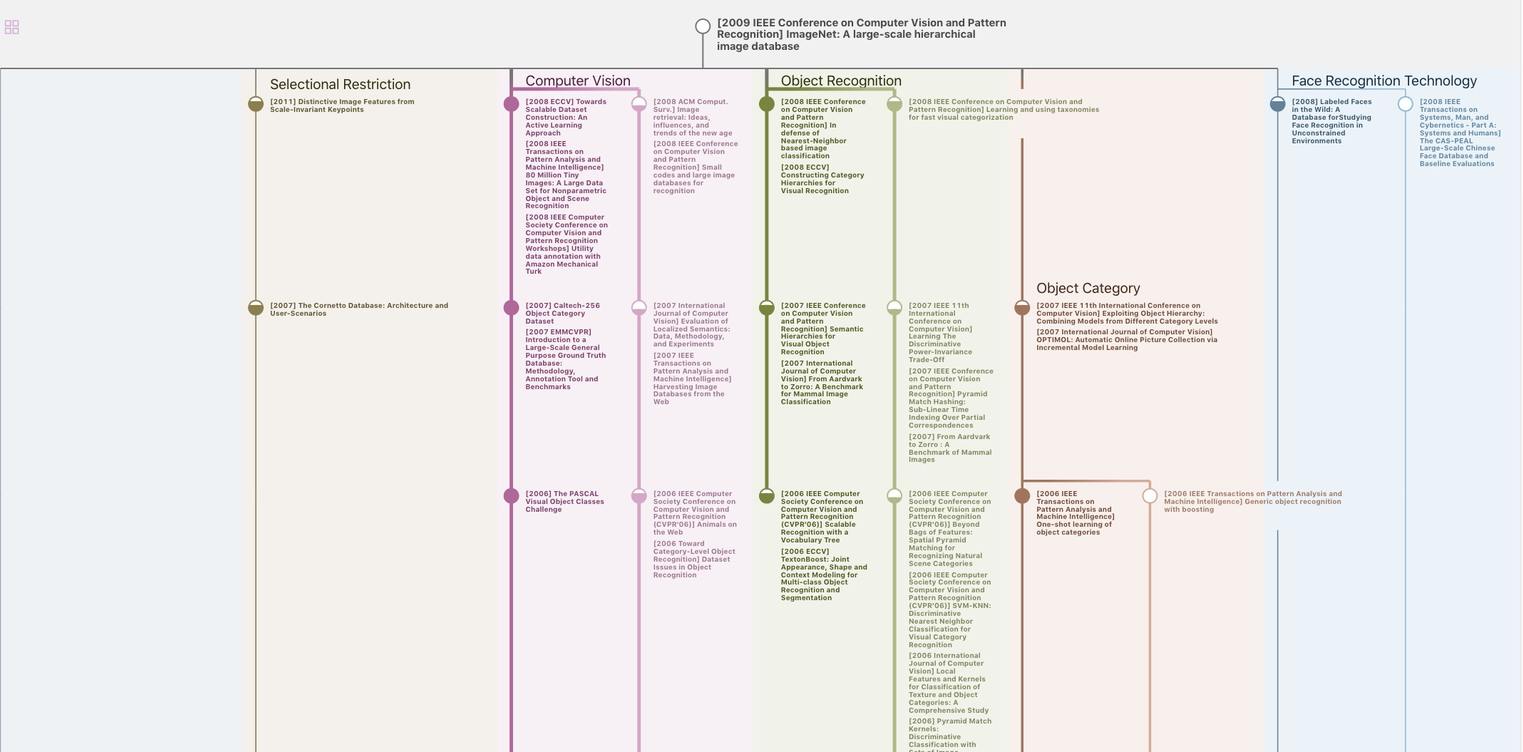
生成溯源树,研究论文发展脉络
Chat Paper
正在生成论文摘要