Hidden Markov Experts for Time Series Prediction
semanticscholar(2004)
摘要
⎯ A modularised model, based on the Mixture of Experts (ME) algorithm for time series prediction, is introduced. A set of connectionist models learn to be local experts over some phases of the trajectory in the state space, so the trajectory-regression task is divided into some simple ones and precise approximation is expected. The dynamics for tracking the local experts is Markovian, where the phases of the trajectory are regarded as the states of a HMM. The state transition on the Markov chain is the process of the underlying system evolving from one phase to another on the trajectory, it is also the process of activating a different local expert or activating some of them simultaneously by different probabilities. The transition probabilities of the hidden Markov model (HMM) are designed to be time-varying and a modified Baum–Welch algorithm is introduced for the learning of the “time-line” HMM. Two versions of the model, based on Multilayer Perceptron (MLP) and Radial Basis Function (RBF) structured experts, have been constructed and demonstrated with two chaotic time series on one-step-ahead prediction. Experiments show this time-varying approach achieves better generalization performance than the corresponding global models. Keywords⎯Time Series prediction, mixture of experts, hidden Markov model, expectation and maximisation
更多查看译文
AI 理解论文
溯源树
样例
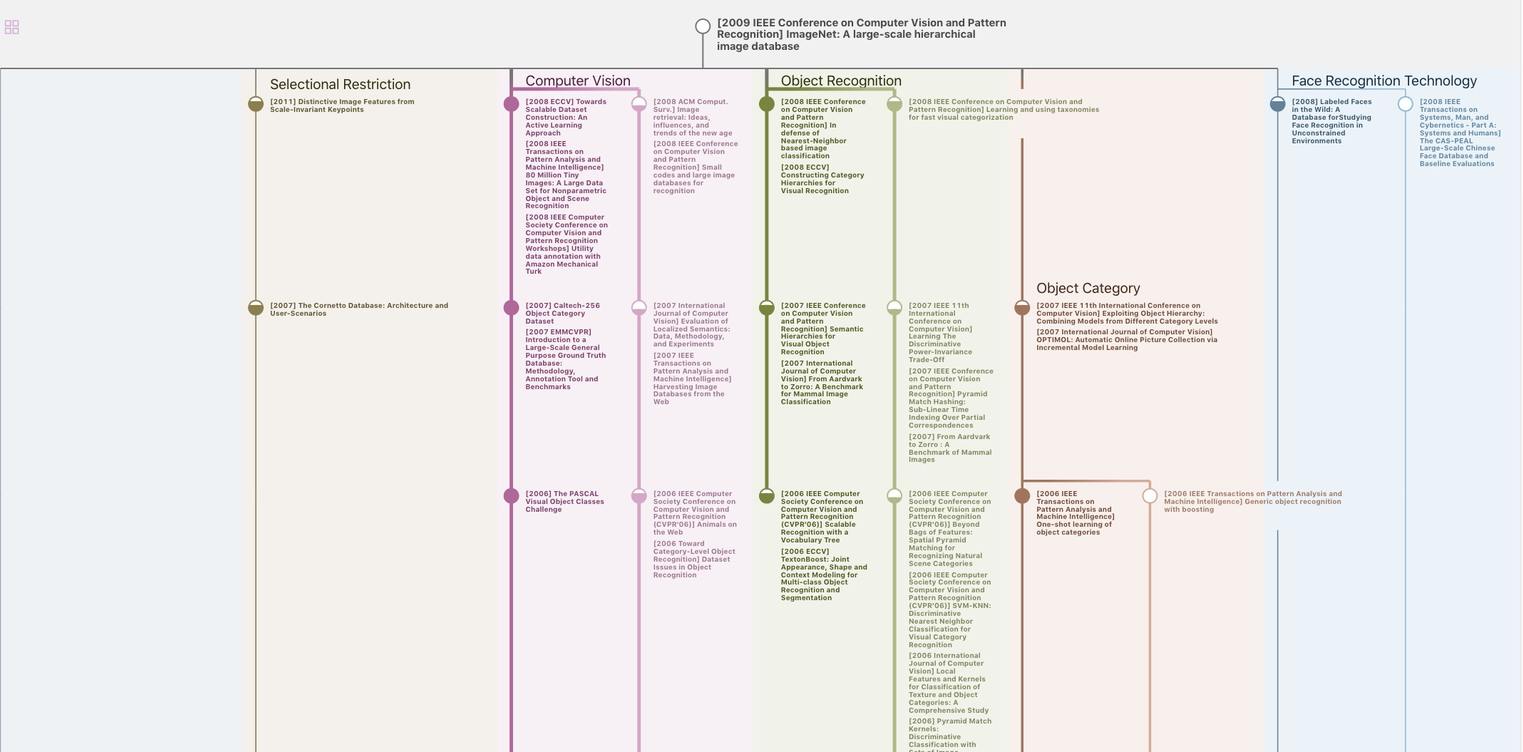
生成溯源树,研究论文发展脉络
Chat Paper
正在生成论文摘要