Multi-objective genetic algorithm
semanticscholar(2011)
摘要
Real world problems often present multiple, frequently conflicting, objectives. The research for optimal solutions of multi-objective problems can be achieved through means of genetic algorithms, which are inspired by the natural process of evolution: an initial population of solutions is randomly generated, then pairs of solutions are selected and combined in order to create new solutions slightly different from the initial solutions. The fittest solutions are kept in the population and are used to generate new solutions. Generations after generations, the initially random population converge towards optimal solutions. The genetic algorithms have the advantage of being easily implemented in any multi-objective problem, and are used in numerous area such as safety systems and electrical network. In this report, key concepts related to multi-objective optimization problems have been presented, such as the notion of decision variables (the actionable characteristics of the problem), objective functions (the relations between the actionable and the observable characteristics) and Pareto optimality (which formally describe how to compare solutions in a multi-objective problem). The mechanisms of genetic algorithms have been described, including the following: the crossover and the mutation which constitute the mechanism of reproduction, the method used for ranking the solutions in a population and the mechanism of selection. A genetic algorithm was implemented to solve a two-objective problem consisting in expanding a power transmission network while optimizing the cost and the reliability of the network. A flaw of the genetic algorithm was illustrated by this example: in spite of a clear convergence of the algorithm towards optimal solutions, the genetic algorithm did not cover uniformly the search space, and the most interesting part (the solutions with low cost) was not explored. Three methods were proposed to guide the convergence of the genetic algorithm towards desired solutions: biasing the generation of the initial population in order to favour the exploration of a certain region of the search space, attributing different weights to the objectives and comparing the solutions according to the weighted sum of the objectives (WPMOGA), and including minimum and maximum trades-off in the comparison of solutions (G-MOGA). The three methods were tested on the network expansion problem in order to find solutions that imply adding
更多查看译文
AI 理解论文
溯源树
样例
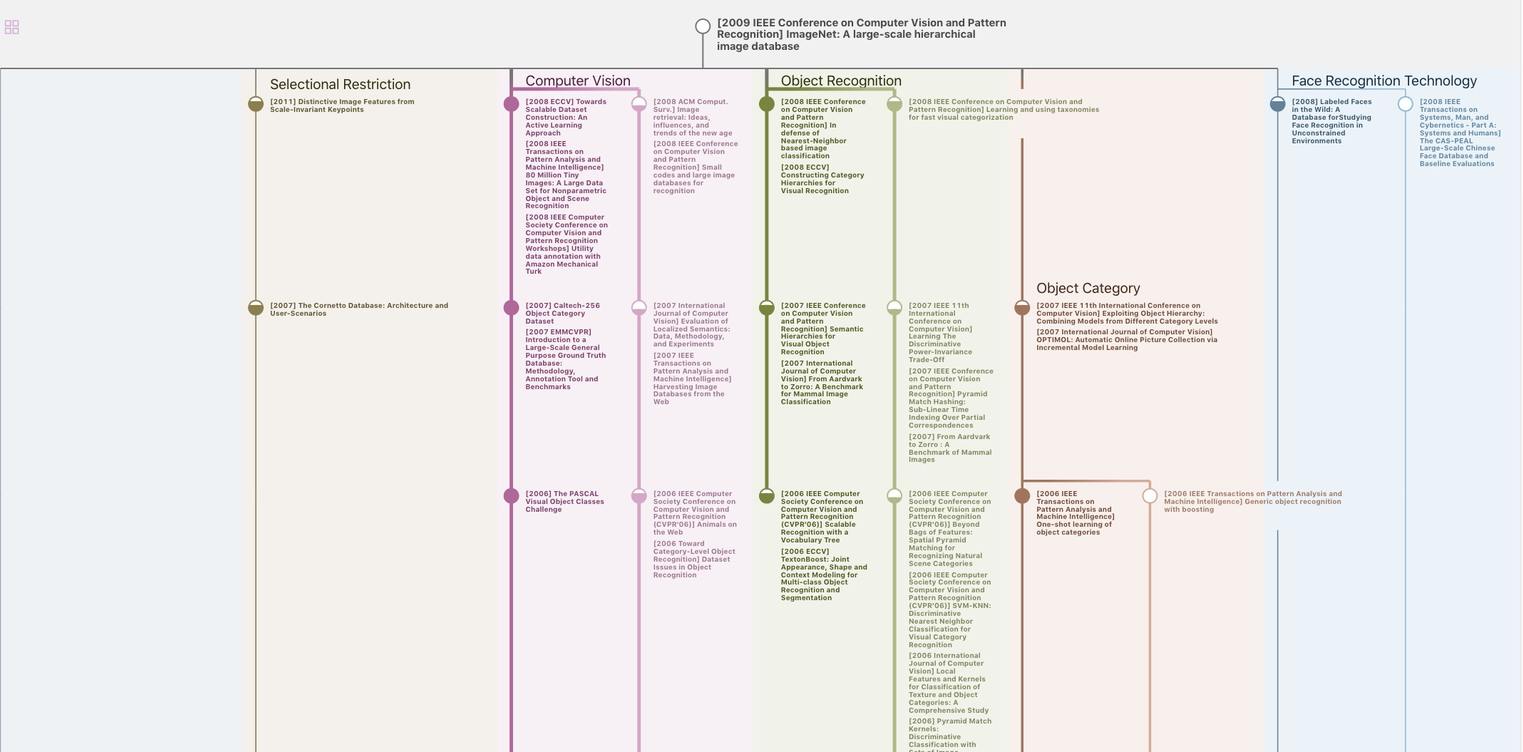
生成溯源树,研究论文发展脉络
Chat Paper
正在生成论文摘要