The Sparse + Low-Rank Trick for Matrix Factorization-Based Graph Algorithms
semanticscholar(2019)
摘要
Matrix factorization is a central block in many graph algorithms for embedding, clustering and a number of other tasks. This block is usually the computational bottleneck of these algorithms and a naive implementation can prevent them from scaling to large datasets. However, the matrices to factorize often have a closedform "sparse + low-rank" structure. In this paper, we show how to adapt state-of-the-art matrix factorization techniques to this class of matrices. We demonstrate that the method is highly competitive with respect to a naive implementation and that it comes at a very small extra cost as compared to the decomposition of the sparse component alone.
更多查看译文
AI 理解论文
溯源树
样例
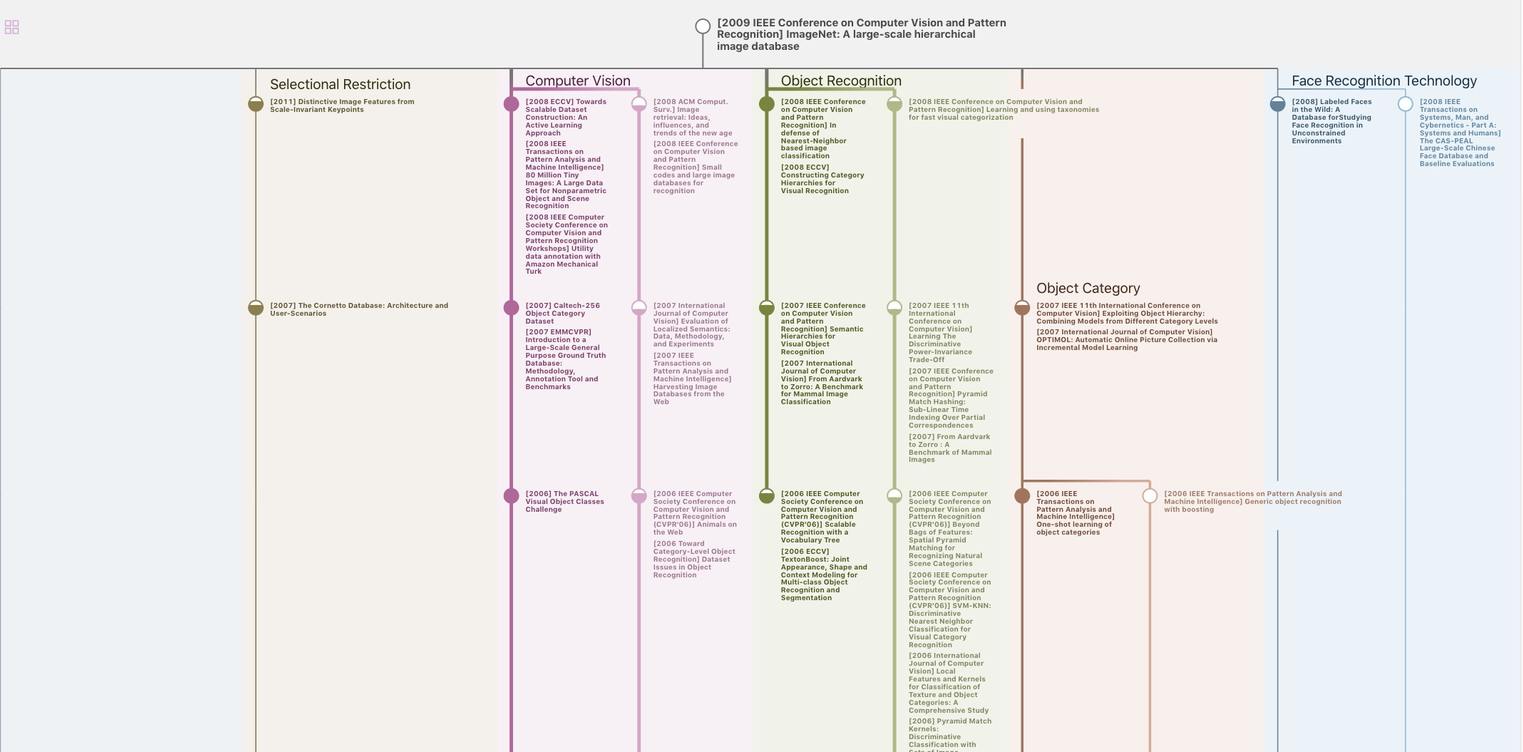
生成溯源树,研究论文发展脉络
Chat Paper
正在生成论文摘要