Physics-informed generative learning to emulate unresolved physics in climate models
AGUFM(2018)
摘要
Simulating Earth's climate often involves solving nonlinear coupled PDEs with multi-scale physics that cannot be fully resolved and requires parameterizations for sub-grid scale phenomena. Therefore, reliable and accurate models to parameterize unresolved and under-resolved physics, such as atmospheric convection, remain an important requirement for simulating climate systems. Recently, Machine Learning has proven to be successful in many data-driven tasks, including in mimicking distributions of processes in complex systems using a flavor of deep neural networks called generative adversarial networks (GANs). GANs have also been designed to generate solutions of PDEs governing complex systems without having to numerically solve these PDEs, by using examples from hi-fidelity simulations or experimental data as training data.
更多查看译文
AI 理解论文
溯源树
样例
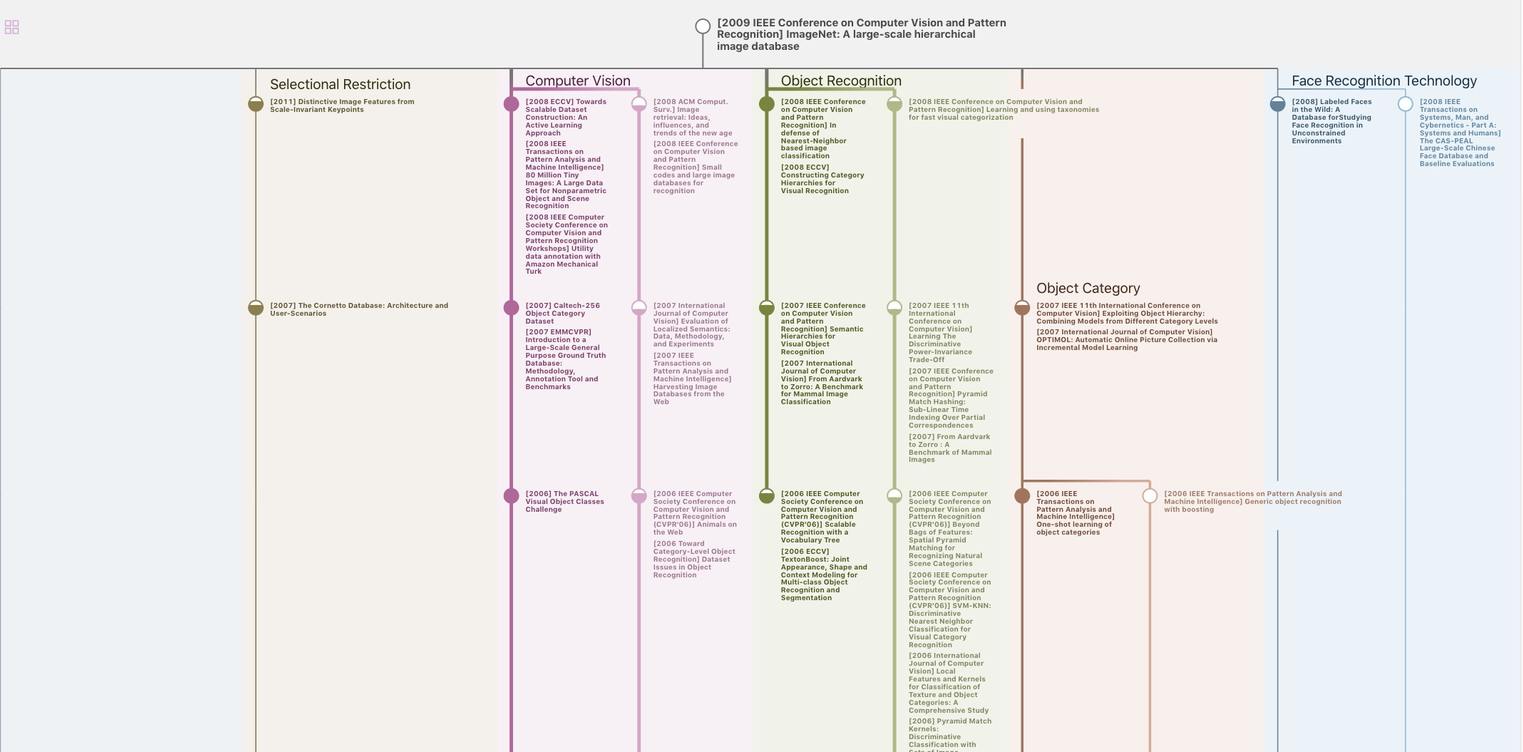
生成溯源树,研究论文发展脉络
Chat Paper
正在生成论文摘要