Fairness and Diversity in Social-Based Recommender Systems
UMAP '20: 28th ACM Conference on User Modeling, Adaptation and Personalization Genoa Italy July, 2020(2020)
摘要
In social networks, the phenomena of homophily and influence explain the fact that friends tend to be similar. Social-based recommenders exploit this observation by incorporating the social structure in collaborative filtering techniques. In practice, these recommenders tend to make friends appear more similar compared to non-socially aware techniques. Various proposals have demonstrated the benefit of incorporating social connections. But at what cost? In this work, we show that there exist users that are mistreated in social recommenders. Specifically, their individual preferences are suppressed more compared to other users in their social circle. We seek to identify who they are and develop techniques that protect them, without severely affecting the effectiveness of the recommender.
更多查看译文
AI 理解论文
溯源树
样例
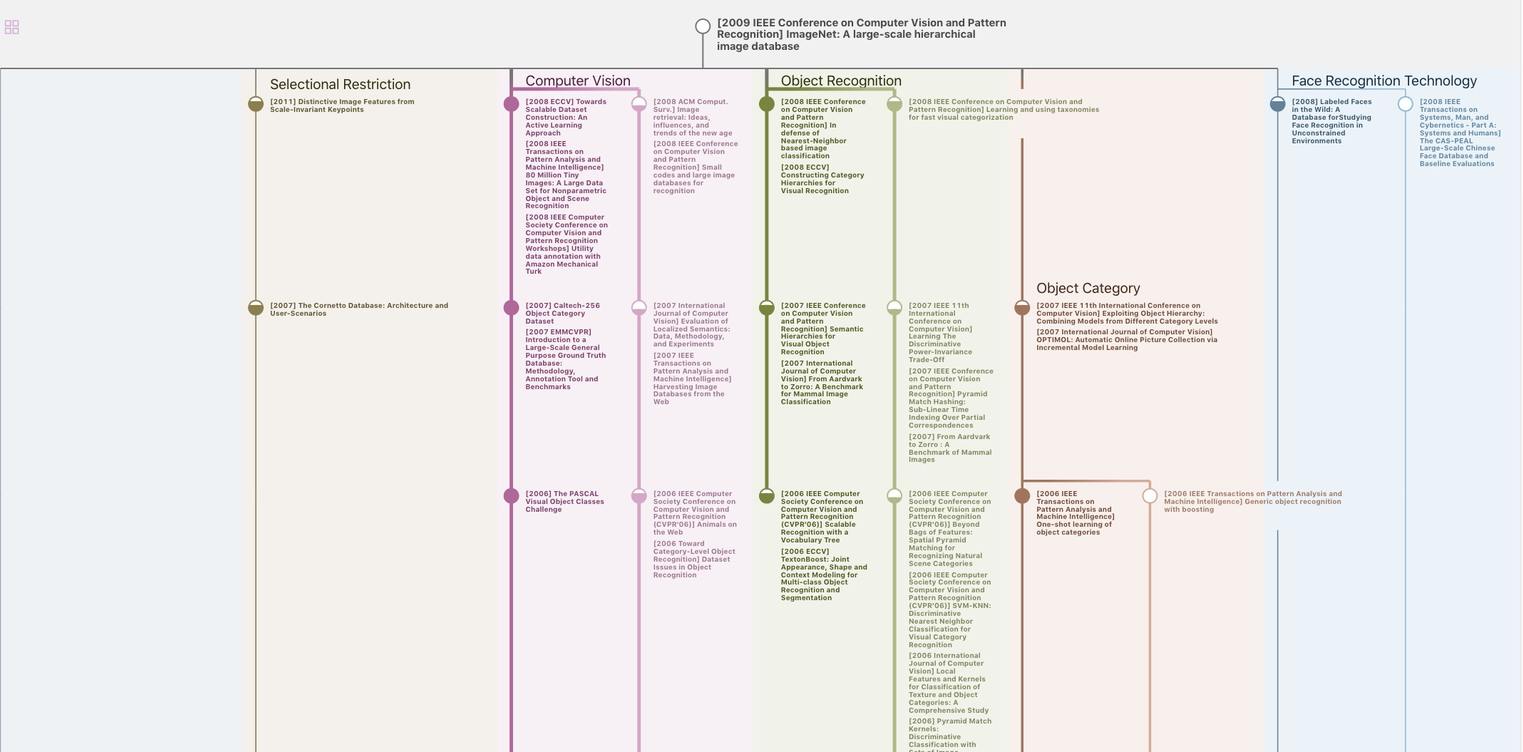
生成溯源树,研究论文发展脉络
Chat Paper
正在生成论文摘要