Transitive Sequencing Medical Records for Mining Predictive and Interpretable Temporal Representations
Patterns(2020)
摘要
Electronic health records (EHRs) contain important temporal information about the progression of disease and treatment outcomes. This paper proposes a transitive sequencing approach for constructing temporal representations from EHR observations for downstream machine learning. Using clinical data from a cohort of patients with congestive heart failure, we mined temporal representations by transitive sequencing of EHR medication and diagnosis records for classification and prediction tasks. We compared the classification and prediction performances of the transitive sequential representations (bag-of-sequences approach) with the conventional approach of using aggregated vectors of EHR data (aggregated vector representation) across different classifiers. We found that the transitive sequential representations are better phenotype “differentiators” and predictors than the “atemporal” EHR records. Our results also demonstrated that data representations obtained from transitive sequencing of EHR observations can present novel insights about the progression of the disease that are difficult to discern when clinical data are treated independently of the patient's history.
更多查看译文
关键词
DSML 2: Proof-of-Concept: Data science output has been formulated, implemented, and tested for one domain/problem
AI 理解论文
溯源树
样例
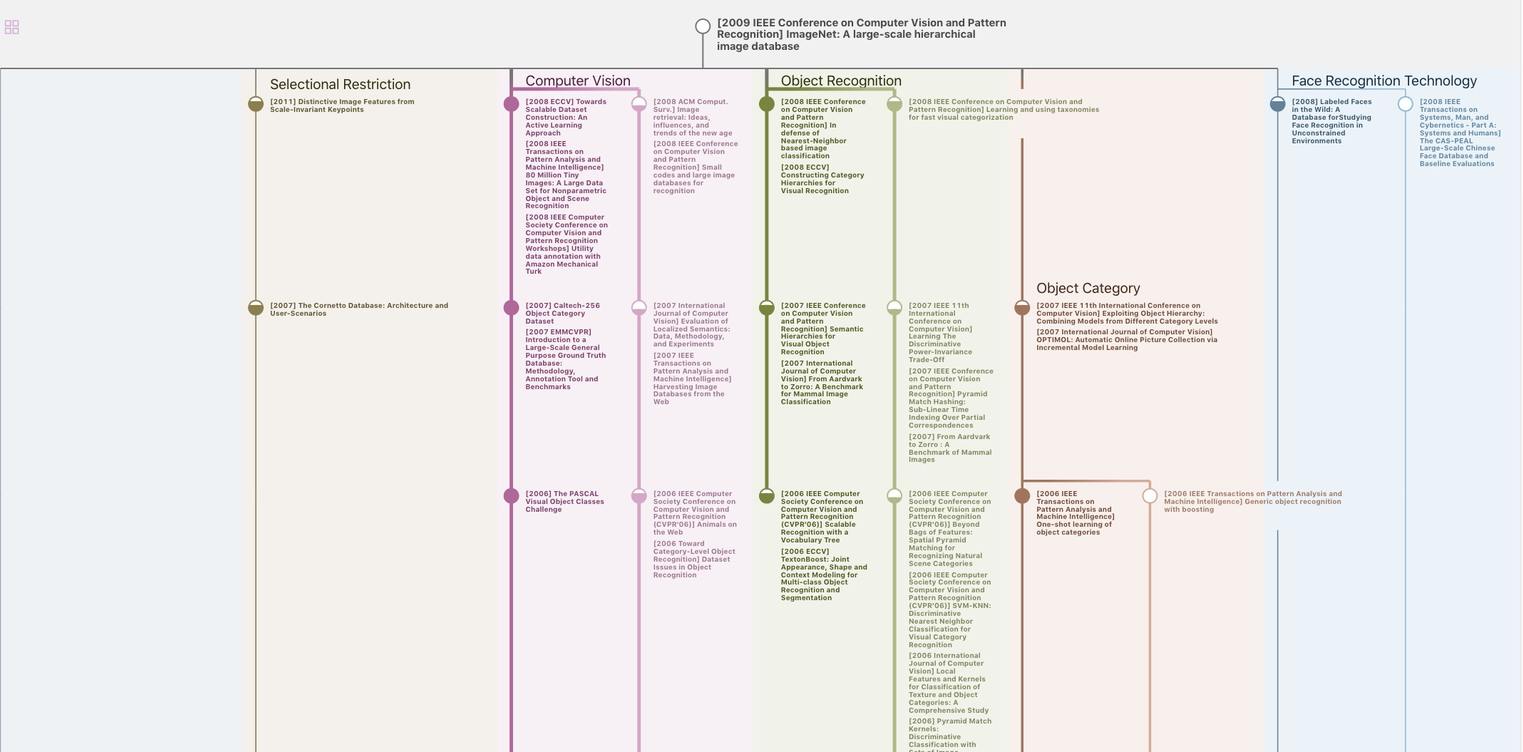
生成溯源树,研究论文发展脉络
Chat Paper
正在生成论文摘要