Transferable Calibration with Lower Bias and Variance in Domain Adaptation
NIPS 2020(2020)
摘要
Domain Adaptation (DA) enables transferring a learning machine from a labeled source domain to an unlabeled target domain. While remarkable advances have been made, most of the existing DA methods focus on improving the target accuracy at inference. How to estimate the predictive uncertainty of DA models is vital for decision-making in safety-critical scenarios but remains the boundary to explore. In this paper, we delve into the open problem of Calibration in DA, which is extremely challenging due to the coexistence of domain shift and the lack of target labels. We first reveal the dilemma that DA models learn higher accuracy at the expense of well-calibrated probabilities. Driven by this finding, we propose Transferable Calibration (TransCal) to tackle this dilemma, achieving accurate calibration with lower bias and variance in a unified hyperparameter-free optimization framework. As a general post-hoc calibration method, TransCal can be easily applied to recalibrate existing DA methods. Its efficacy has been justified both theoretically and empirically.
更多查看译文
关键词
transferable calibration,domain adaptation,lower bias
AI 理解论文
溯源树
样例
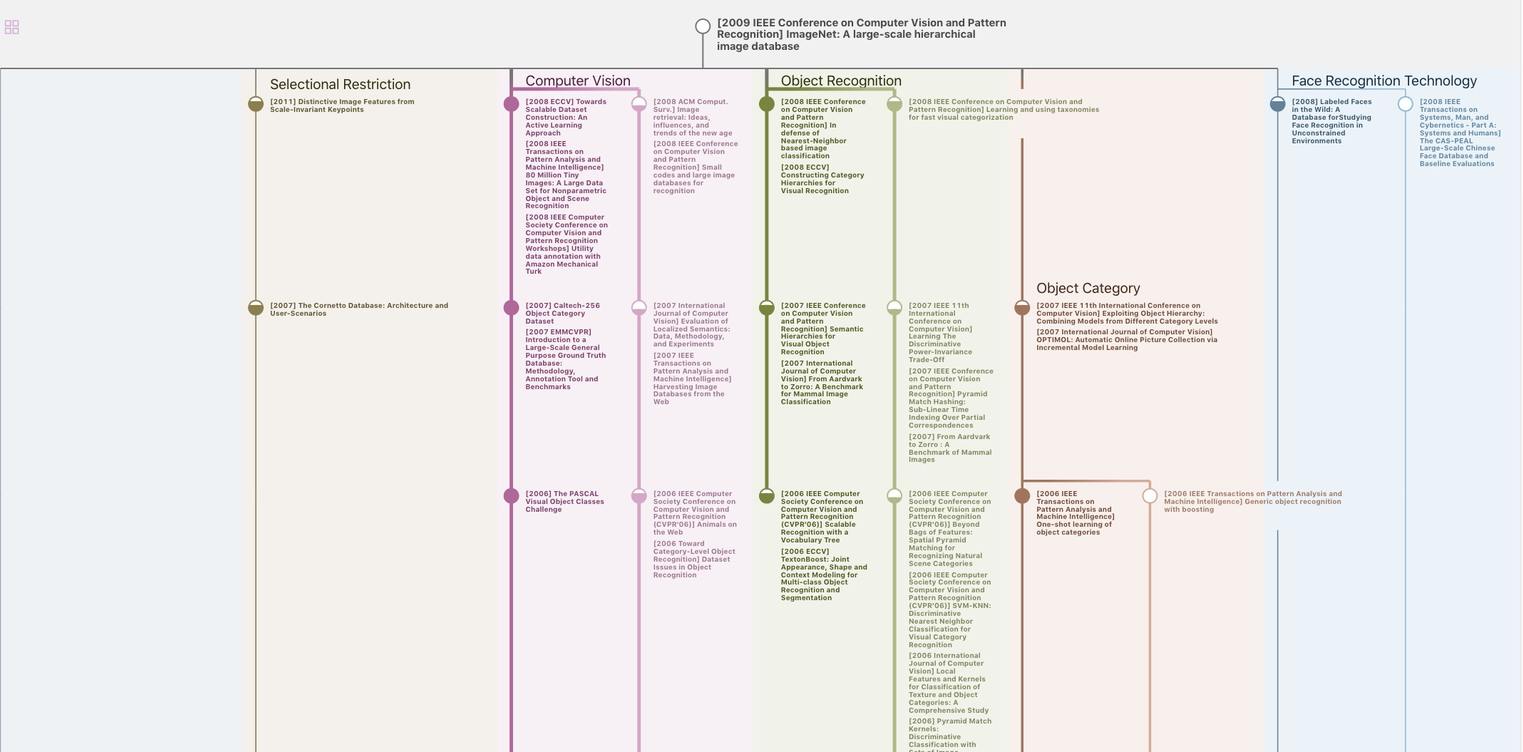
生成溯源树,研究论文发展脉络
Chat Paper
正在生成论文摘要