Data-Driven Load Data Cleaning and Its Impacts on Forecasting Performance
2019 IEEE Sustainable Power and Energy Conference (iSPEC)(2019)
摘要
Massive and various bad data may be introduced to load profiles in the process of data acquisition, transmission, and storage deliberately or accidentally such as cyber attacks and equipment failures. The bad data may result in bias for load forecasting and other data analytic applications. This paper proposes a novel bad data identification and repairing method for load profiles. In the first stage, Singular Value Thresholding (SVT) algorithm is applied to complete the missing data and detect the anomaly spikes roughly. In the second stage, quantile regression with lag value is performed to detect local fluctuation. How the data cleaning influences the forecasting performance is also investigated. Case studies on load of Fujian Province, China are conducted to verify the effectiveness of the proposed method.
更多查看译文
AI 理解论文
溯源树
样例
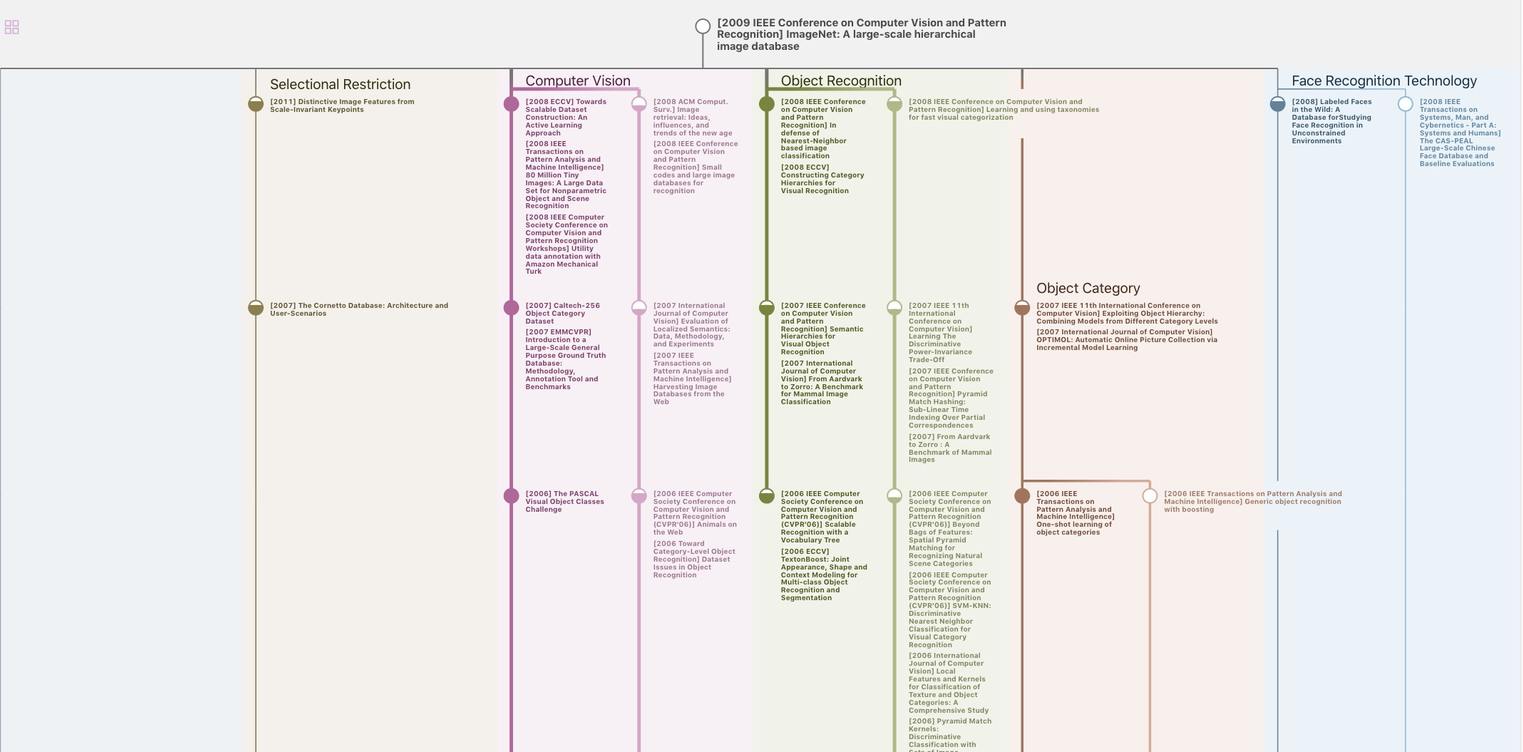
生成溯源树,研究论文发展脉络
Chat Paper
正在生成论文摘要