Differentiated Transmission based on Traffic Classification with Deep Learning in DataCenter
2020 IFIP Networking Conference (Networking)(2020)
摘要
Production datacenters generally collect diverse applications with differentiated requirements, e.g., low latency and high throughput. Therefore, datacenter transmission schemes must strive to meet these requirements. However, despite significant efforts, prior solutions are either ineffective to satisfy different requirements or costly to apply. Besides, no scheme can accommodate to all diverse data center scenarios or dynamic traffic patterns. In this paper, we propose SmartTrans, a deep learning based latency-aware differentiated transmission service, including three main components. First, SmartTrans utilizes deep learning methods for traffic classification and flow size rank prediction. Second, according to the classified results of flows, SmartTrans adopts multilevel priority queues to execute differentiated scheduling. Third, SmartTrans enlarges the switch butter to increase the capacity of datacenter networks (DCN), which effectively fights against the traffic burst and improves the throughput of latency-insensitive flows. We evaluate SmartTrans with several real workloads. Experiment results show that SmartTrans achieves both the low average flow completion time (FCT) for latency-sensitive flows and the high throughput for latency-insensitive flows.
更多查看译文
关键词
flow scheduling,data center,deep learning,latency,throughput
AI 理解论文
溯源树
样例
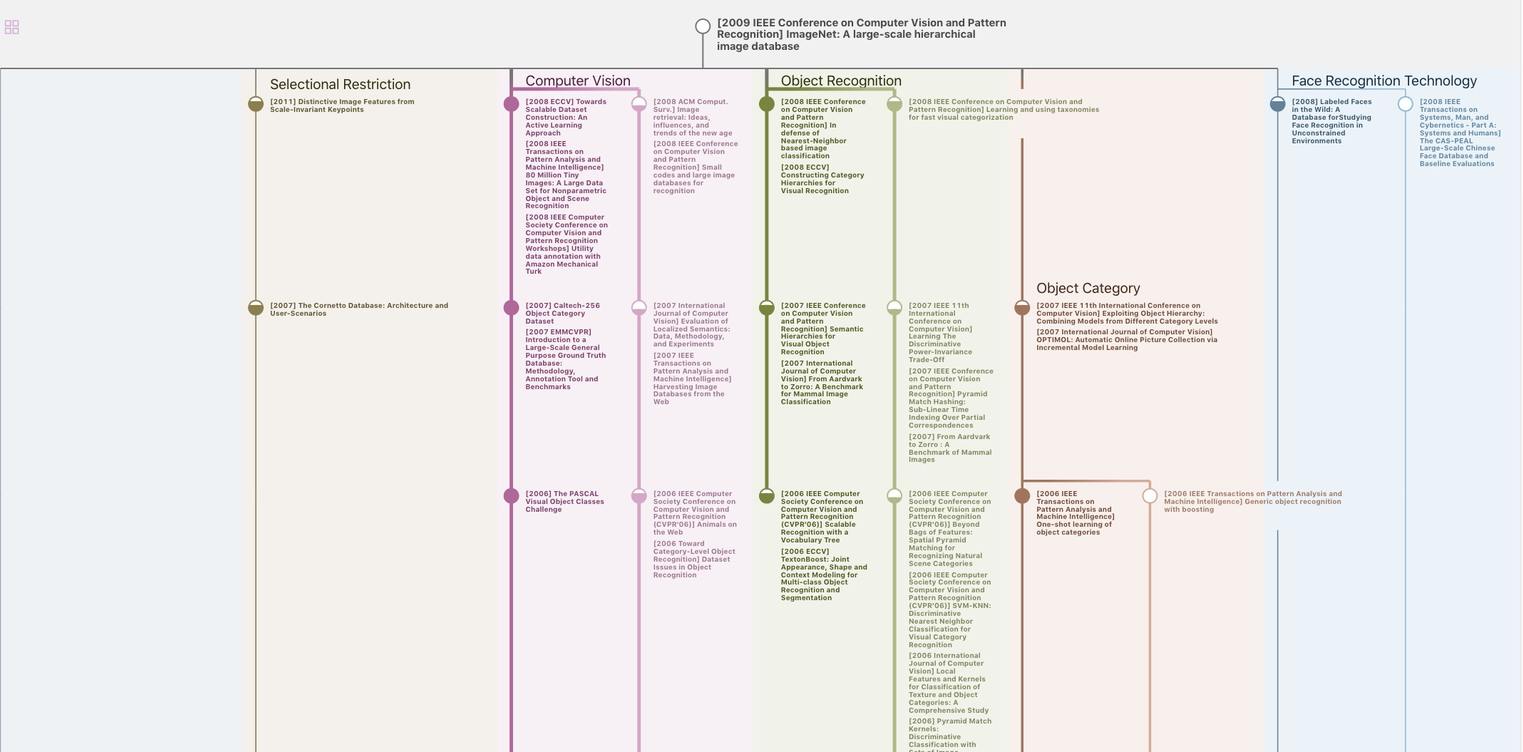
生成溯源树,研究论文发展脉络
Chat Paper
正在生成论文摘要