Explanation-Guided Training for Cross-Domain Few-Shot Classification
2020 25TH INTERNATIONAL CONFERENCE ON PATTERN RECOGNITION (ICPR)(2021)
摘要
Cross-domain few-shot classification task (CD-FSC) combines few-shot classification with the requirement to generalize across domains represented by datasets. This setup faces challenges originating from the limited labeled data in each class and, additionally, from the domain shift between training and test sets. In this paper, we introduce a novel training approach for existing FSC models. It leverages on the explanation scores, obtained from existing explanation methods when applied to the predictions of FSC models, computed for intermediate feature maps of the models. Firstly, we tailor the layer-wise relevance propagation (LRP) method to explain the predictions of FSC models. Secondly, we develop a model-agnostic explanation-guided training strategy that dynamically finds and emphasizes the features which are important for the predictions. Our contribution does not target a novel explanation method but lies in a novel application of explanations for the training phase. We show that explanation-guided training effectively improves the model generalization. We observe improved accuracy for three different FSC models: RelationNet, cross attention network, and a graph neural network-based formulation, on five few-shot learning datasets: miniImagenet, CUB, Cars, Places, and Plantae. The source code is available https://github.com/SunJiamei/few-shot-lrp-guided
更多查看译文
关键词
intermediate feature maps,layer-wise relevance propagation method,model-agnostic explanation-guided training strategy,training phase,model generalization,few-shot learning datasets,cross-domain few-shot classification task,CD-FSC,domain shift,explanation scores,FSC models,graph neural network,RelationNet cross attention network
AI 理解论文
溯源树
样例
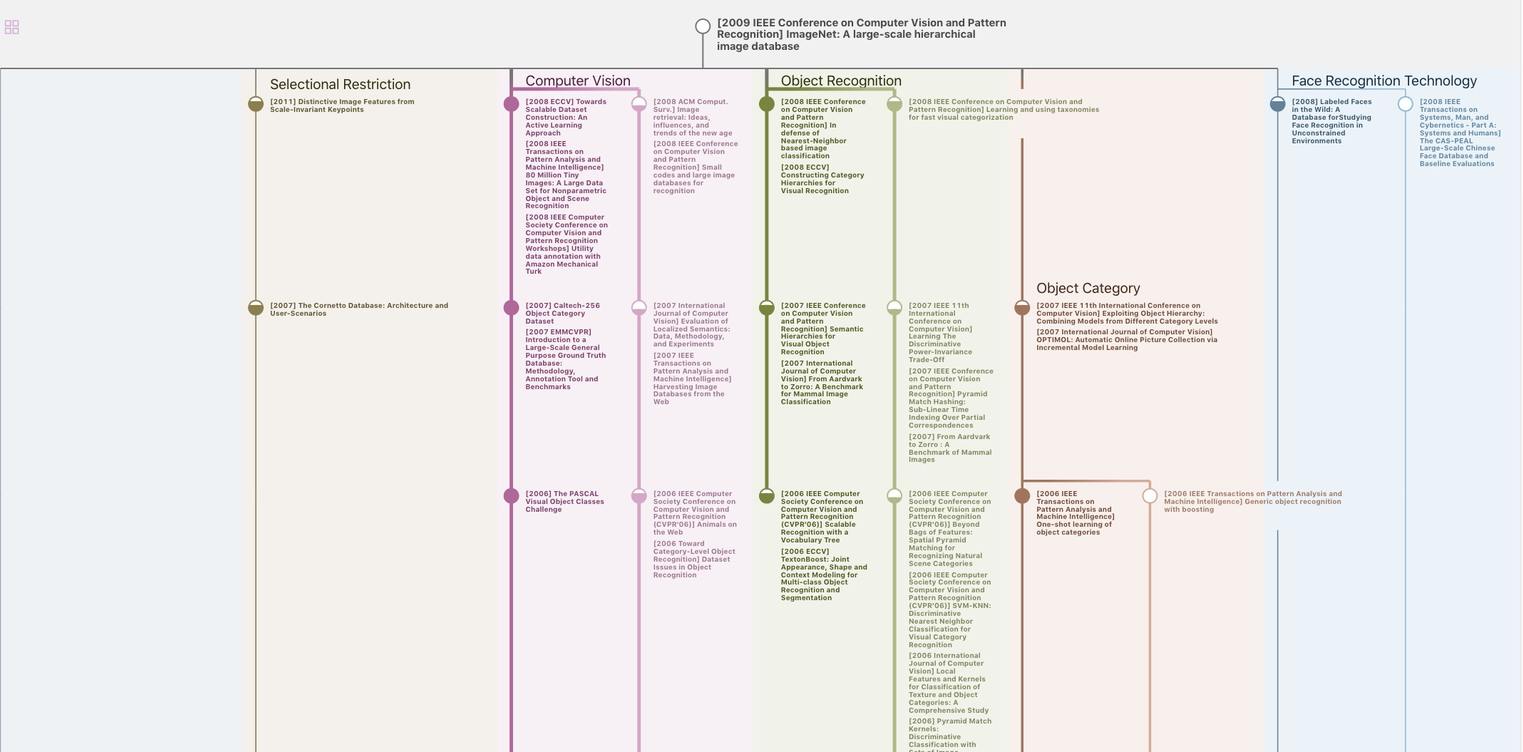
生成溯源树,研究论文发展脉络
Chat Paper
正在生成论文摘要