Tighter Generalization Bounds for Iterative Differentially Private Learning Algorithms.
UAI(2021)
摘要
This paper studies the relationship between generalization and privacy preservation in iterative learning algorithms by two sequential steps. We first establish the generalization-privacy relationship for any learning algorithm. We prove that $(\varepsilon, \delta)$-differential privacy implies an on-average generalization bound for multi-database learning algorithms which further leads to a high-probability generalization bound. The high-probability generalization bound implies a PAC-learnable guarantee for differentially private algorithms. We then investigate how the iterative nature would influence the generalizability and privacy. Three new composition theorems are proposed to approximate the $(\varepsilon', \delta')$-differential privacy of any iterative algorithm through the differential privacy of its every iteration. By integrating the above two steps, we deliver two generalization bounds for iterative learning algorithms, which characterize how privacy-preserving ability guarantees generalizability and how the iterative nature contributes to the generalization-privacy relationship. All the theoretical results are strictly tighter than the existing results in the literature and do not explicitly rely on the model size which can be prohibitively large in deep models. The theories directly apply to a wide spectrum of learning algorithms. In this paper, we take stochastic gradient Langevin dynamics and the agnostic federated learning from the client view for examples to show one can simultaneously enhance privacy preservation and generalizability through the proposed theories.
更多查看译文
关键词
iterative differentially private
AI 理解论文
溯源树
样例
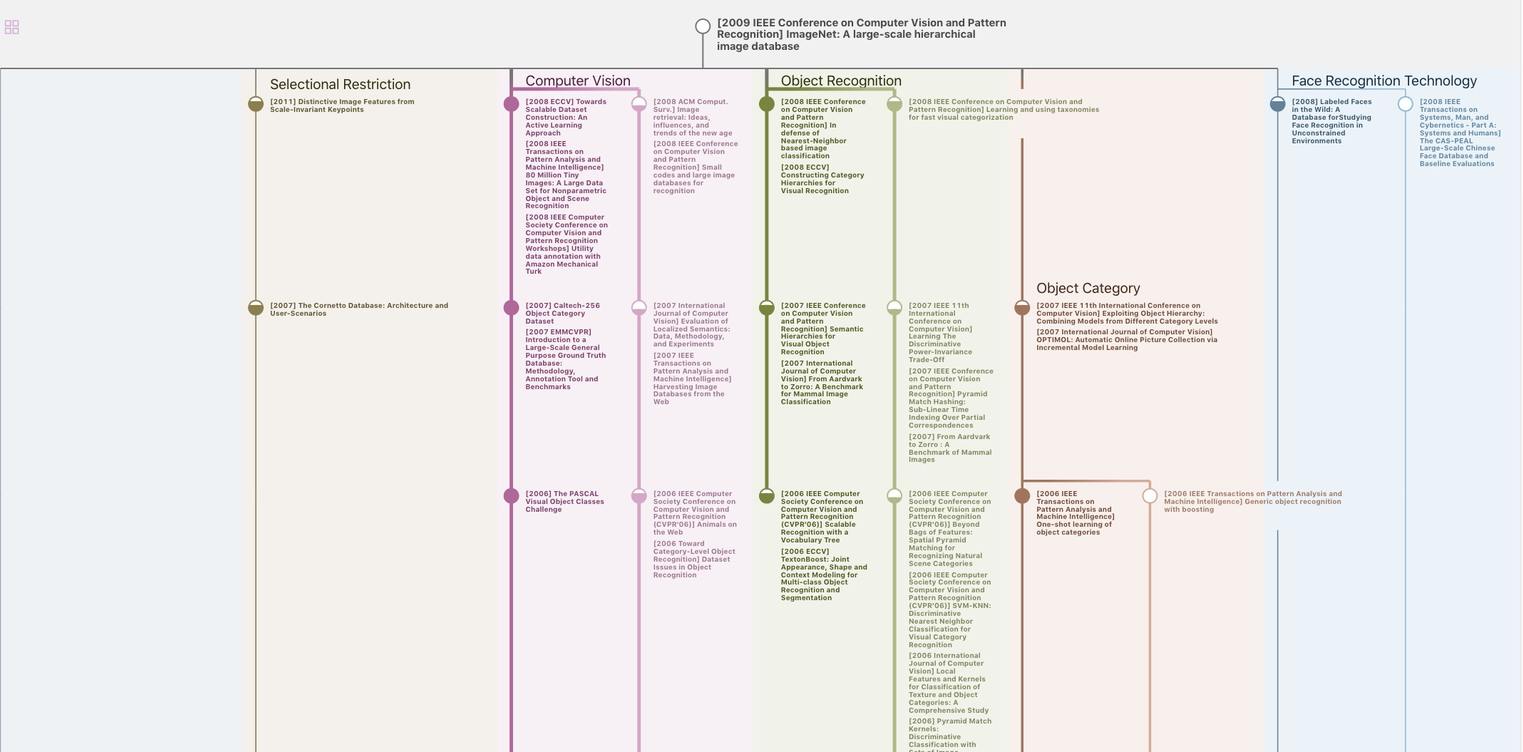
生成溯源树,研究论文发展脉络
Chat Paper
正在生成论文摘要