A parametrized nonlinear predictive control strategy for relaxing COVID-19 social distancing measures in Brazil
ISA Transactions(2022)
摘要
The SARS-CoV-2 virus was first registered in Brazil by the end of February 2020. Since then, the country counts over 150000 deaths due to COVID-19 and faces a profound social and economic crisis; there is also an ongoing health catastrophe, with the majority of hospital beds in many Brazilian cities currently occupied with COVID-19 patients. Thus, a Nonlinear Model Predictive Control (NMPC) scheme used to plan appropriate social distancing measures (and relaxations) in order to mitigate the effects of this pandemic is formulated in this paper. The strategy is designed upon an adapted data-driven Susceptible–Infected–Recovered–Deceased (SIRD) model, which includes time-varying auto-regressive immunological parameters. A novel identification procedure is proposed, composed of analytical regressions, Least-Squares optimization and auto-regressive model fits. The adapted SIRD model is validated with real data and able to adequately represent the contagion curves over large forecast horizons. The NMPC strategy is designed to generate piecewise constant quarantine guidelines, which can be reassessed (relaxed/strengthened) each week. Simulation results show that the proposed NMPC technique is able to mitigate the number of infections and progressively loosen social distancing measures. With respect to a “no-control” condition, the number of deaths could be reduced in up to 30% if the proposed NMPC coordinated health policy measures are enacted.
更多查看译文
关键词
Nonlinear Model Predictive Control,COVID-19,Social isolation,SIRD Model,System Identification
AI 理解论文
溯源树
样例
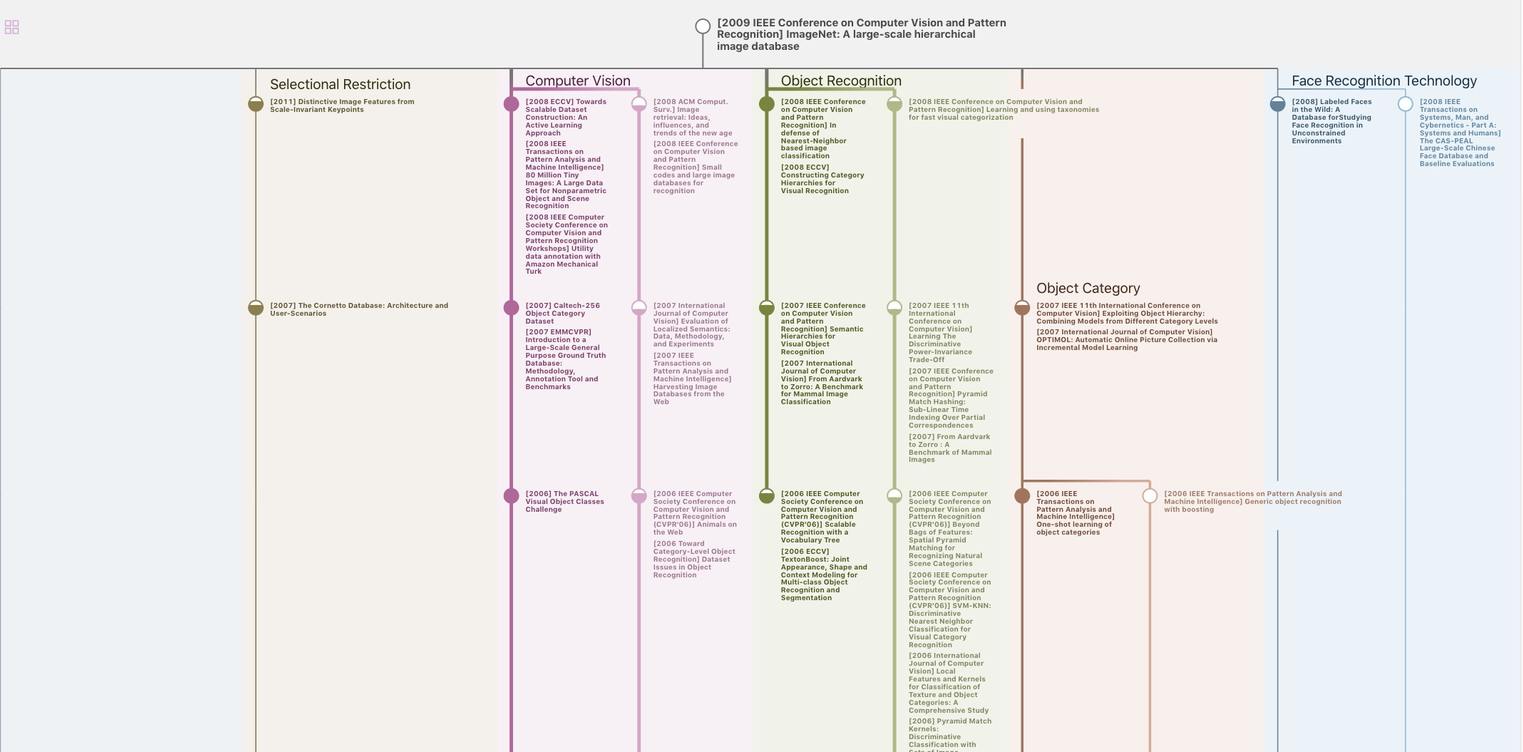
生成溯源树,研究论文发展脉络
Chat Paper
正在生成论文摘要