When deep learning meets causal inference: a computational framework for drug repurposing from real-world data
arxiv(2020)
摘要
Drug repurposing is an effective strategy to identify new uses for existing drugs, providing the quickest possible transition from bench to bedside. Existing methods for drug repurposing that mainly focus on pre-clinical information may exist translational issues when applied to human beings. Real world data (RWD), such as electronic health records and insurance claims, provide information on large cohorts of users for many drugs. Here we present an efficient and easily-customized framework for generating and testing multiple candidates for drug repurposing using a retrospective analysis of RWDs. Building upon well-established causal inference and deep learning methods, our framework emulates randomized clinical trials for drugs present in a large-scale medical claims database. We demonstrate our framework in a case study of coronary artery disease (CAD) by evaluating the effect of 55 repurposing drug candidates on various disease outcomes. We achieve 6 drug candidates that significantly improve the CAD outcomes but not have been indicated for treating CAD, paving the way for drug repurposing.
更多查看译文
关键词
causal inference,drug,deep learning,real-world
AI 理解论文
溯源树
样例
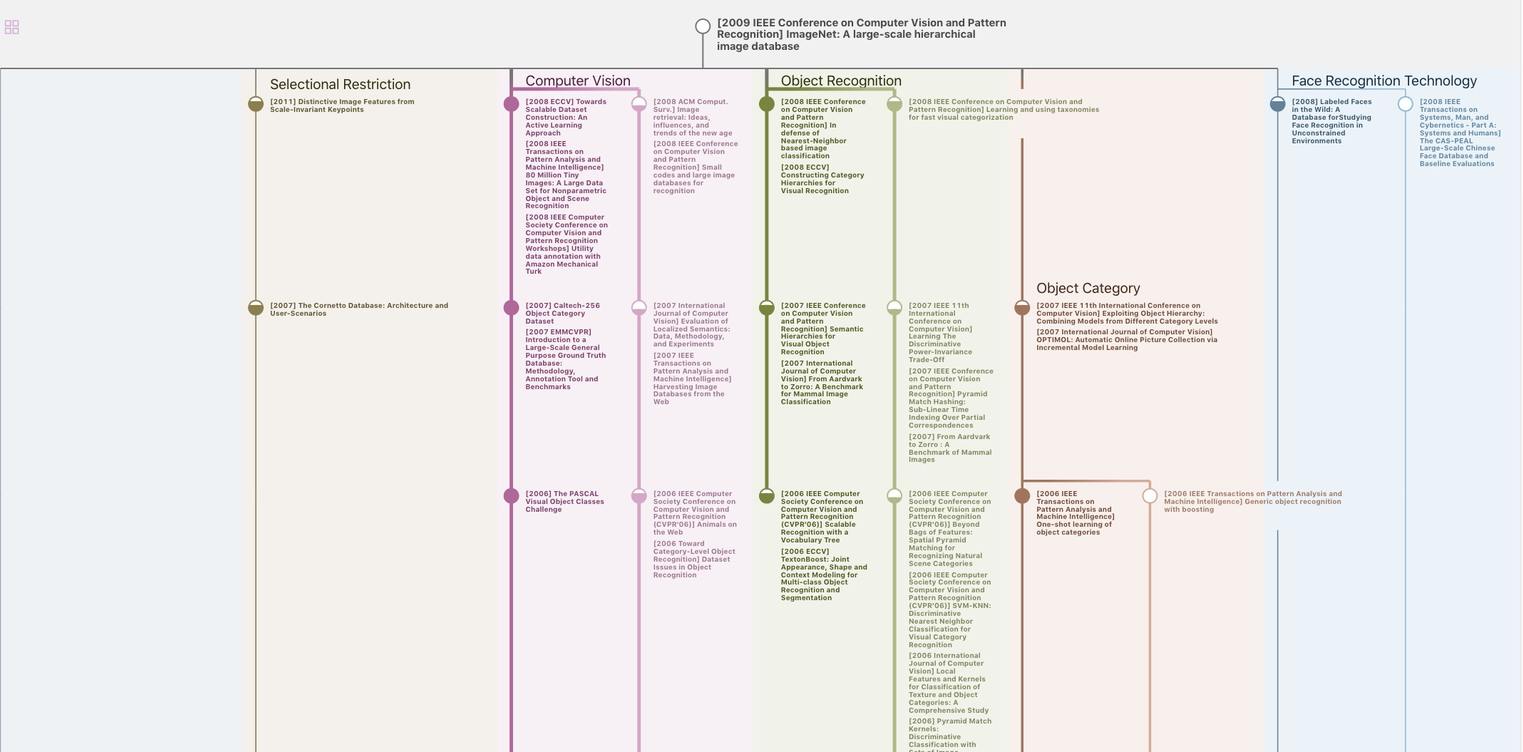
生成溯源树,研究论文发展脉络
Chat Paper
正在生成论文摘要