Discrete Point Flow Networks for Efficient Point Cloud Generation
European Conference on Computer Vision(2020)
摘要
Generative models have proven effective at modeling 3D shapes and their statistical variations. In this paper we investigate their application to point clouds, a 3D shape representation widely used in computer vision for which, however, only few generative models have yet been proposed. We introduce a latent variable model that builds on normalizing flows with affine coupling layers to generate 3D point clouds of an arbitrary size given a latent shape representation. To evaluate its benefits for shape modeling we apply this model for generation, autoencoding, and single-view shape reconstruction tasks. We improve over recent GAN-based models in terms of most metrics that assess generation and autoencoding. Compared to recent work based on continuous flows, our model offers a significant speedup in both training and inference times for similar or better performance. For single-view shape reconstruction we also obtain results on par with state-of-the-art voxel, point cloud, and mesh-based methods.
更多查看译文
关键词
Generative modeling,Normalizing flows,3D shape modeling,Point cloud generation,Single view reconstruction
AI 理解论文
溯源树
样例
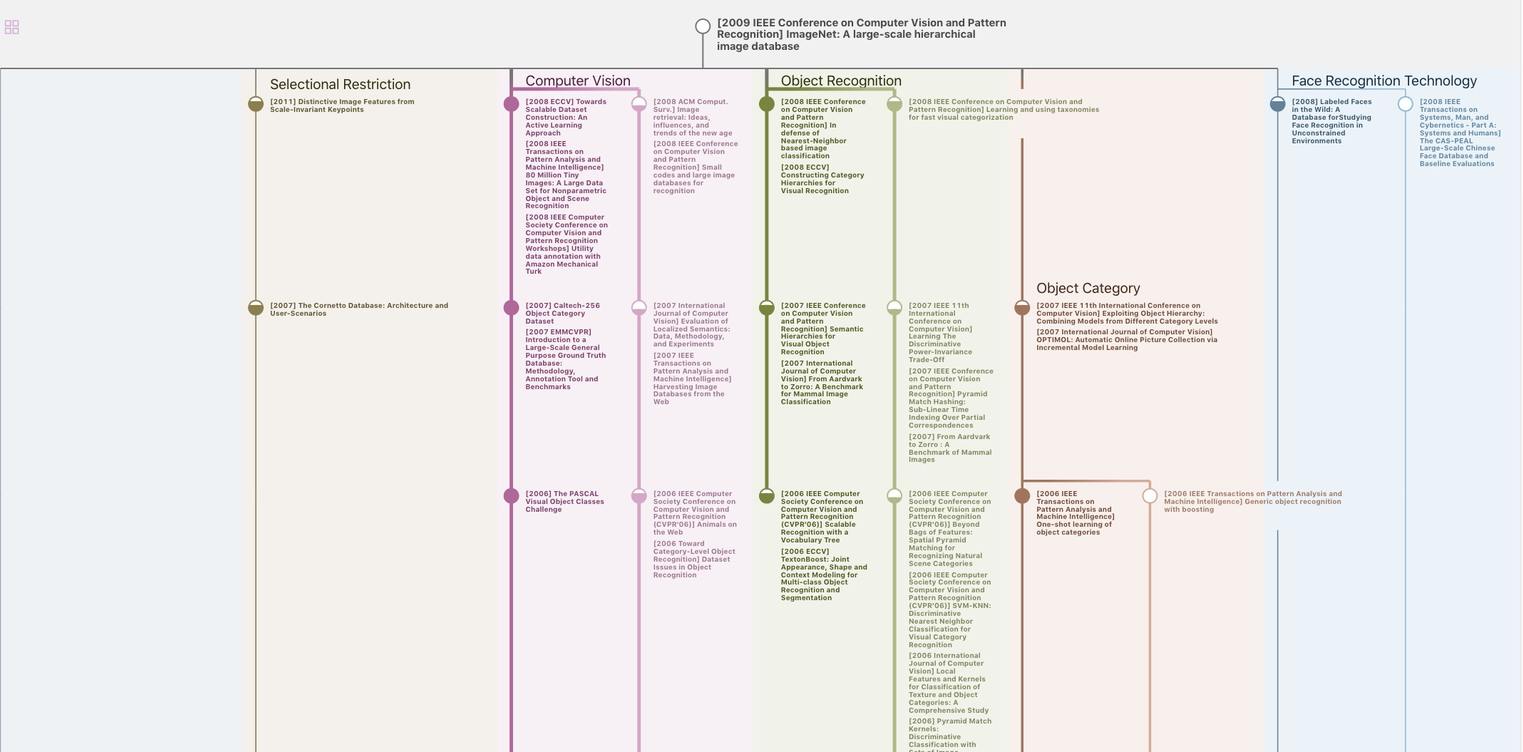
生成溯源树,研究论文发展脉络
Chat Paper
正在生成论文摘要