Balance Scene Learning Mechanism for Offshore and Inshore Ship Detection in SAR Images
IEEE GEOSCIENCE AND REMOTE SENSING LETTERS(2022)
摘要
Huge imbalance of different scenes' sample numbers seriously reduces synthetic aperture radar (SAR) ship detection accuracy. Thus, to solve this problem, this letter proposes a balance scene learning mechanism (BSLM) for offshore and inshore ship detection in SAR images. BSLM involves three steps: 1) based on unsupervised representation learning, a generative adversarial network (GAN) is used to extract the scene features of SAR images; 2) using these features, a scene binary cluster (offshore/inshore) is conducted by K-means; and 3) finally, the small cluster's samples (inshore) are augmented via replication, rotation transformation or noise addition to balance another big cluster (offshore), so as to eliminate scene learning bias and obtain balanced learning representation ability that can enhance learning benefits and improve detection accuracy. This letter applies BSLM to four widely used and open-sourced deep learning detectors, i.e., faster regions-convolutional neural network (Faster R-CNN), Cascade R-CNN, single shot multibox detector (SSD), and RetinaNet, to verify its effectiveness. Experimental results on the open SAR ship detection data set (SSDD) reveal that BSLM can greatly improve detection accuracy, especially for more complex inshore scenes.
更多查看译文
关键词
Balance scene learning mechanism (BSLM),inshore,offshore,ship detection,synthetic aperture radar (SAR)
AI 理解论文
溯源树
样例
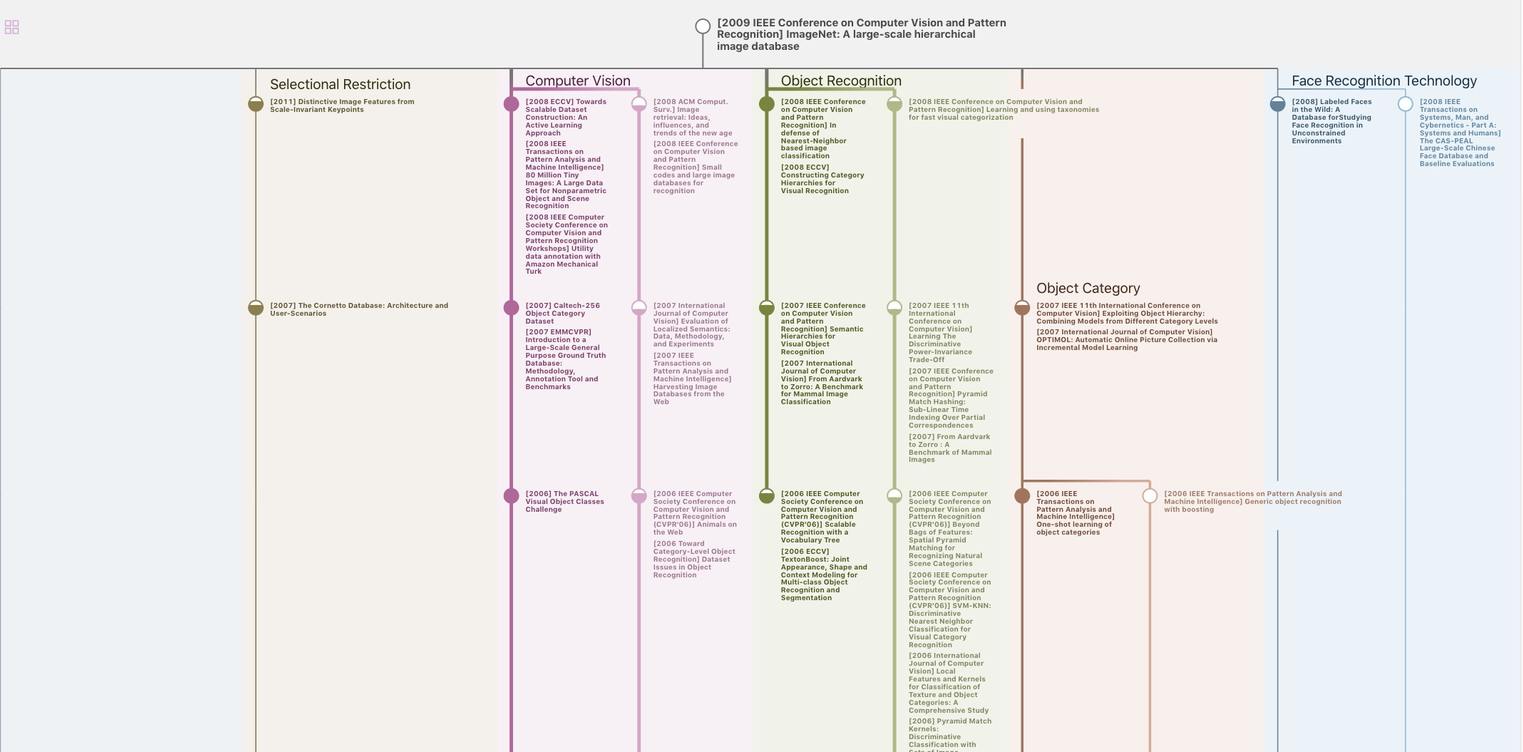
生成溯源树,研究论文发展脉络
Chat Paper
正在生成论文摘要