Automated Detection and Forecasting of COVID-19 using Deep Learning Techniques: A Review
arxiv(2024)
摘要
In March 2020, the World Health Organization (WHO) declared COVID-19 a global epidemic, caused by the SARS-CoV-2 virus. Initially, COVID-19 was diagnosed using real-time reverse transcription–polymerase chain reaction (RT-PCR) tests with a turnaround time of 2-3 days. To enhance diagnostic accuracy, medical professionals use medical imaging alongside RT-PCR. A positive result on both RT-PCR and medical imaging confirms a COVID-19 diagnosis. Imaging modalities like chest X-ray (CXR), computed tomography (CT) scans, and ultrasound are widely utilized for rapid and precise COVID-19 diagnoses. However, interpreting COVID-19 from these images is time-consuming and susceptible to human error. Therefore, leveraging artificial intelligence (AI) methods, particularly deep learning (DL) models, can deliver consistent, high-performance results. Unlike conventional machine learning (ML), DL models automate all stages of feature extraction, selection, and classification. This paper presents a comprehensive review of using DL techniques for diagnosing COVID-19 from medical imaging. The introduction provides an overview of diagnosing the coronavirus using medical imaging, highlighting associated challenges. Subsequently, the paper delves into key aspects of Computer-Aided Diagnosis Systems (CADS) based on DL methods for diagnosing COVID-19, covering segmentation, classification, explainable AI (XAI), and predictive research. Additionally, it reviews the rehabilitation systems such as the Internet of Medical Things (IoMT) in the context of COVID-19. In another section, uncertainty quantification (UQ) research is showcased, focusing on DL models for the diagnosis of Covid-19. Crucial challenges and future research directions are outlined in another section. Finally, discussion and conclusion sections are also provided at the end of the paper.
更多查看译文
关键词
COVID-19,diagnosis,Deep Learning,Explainable AI,Rehabilitation Systems,Uncertainty Quantification
AI 理解论文
溯源树
样例
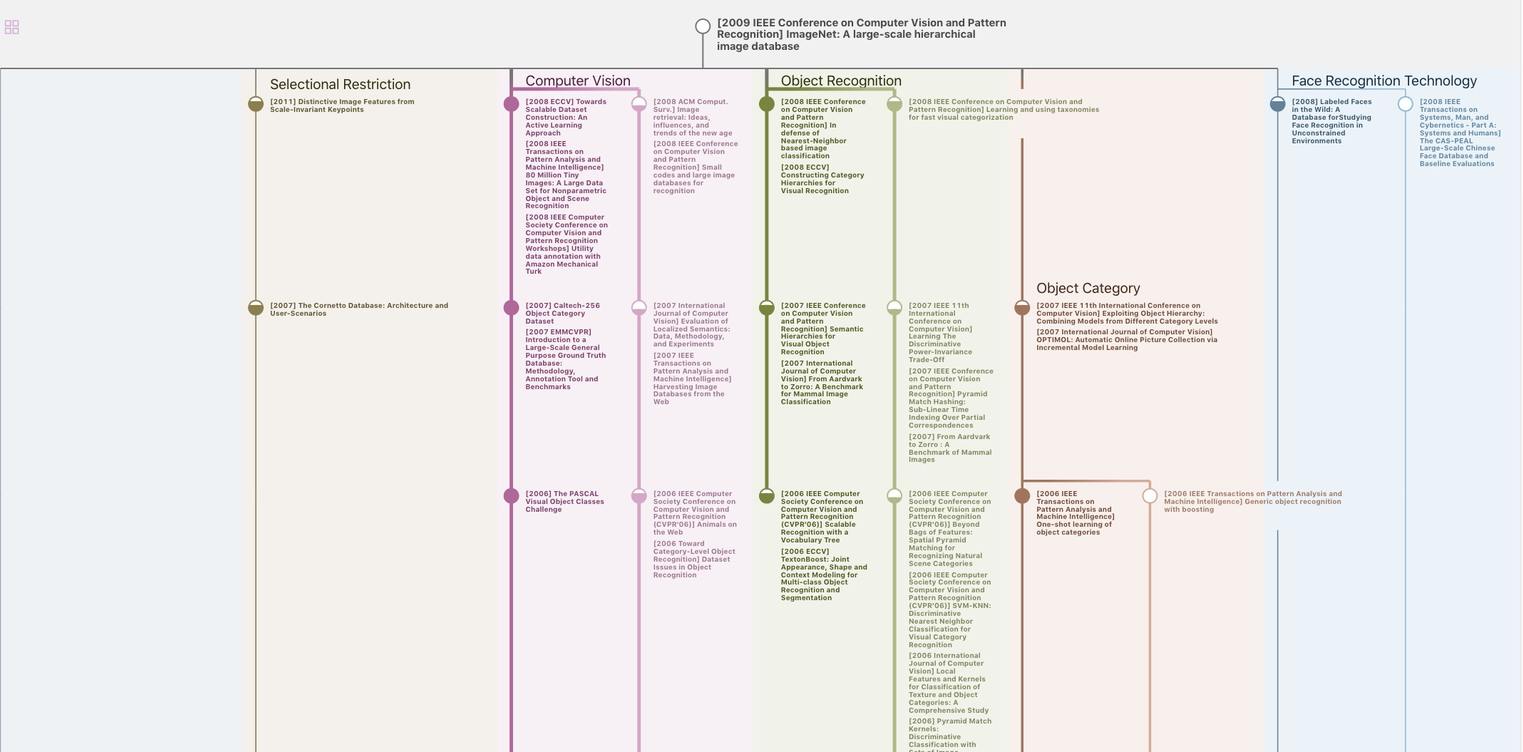
生成溯源树,研究论文发展脉络
Chat Paper
正在生成论文摘要