Parameterizing Variational Methods Through Data-Driven Inverse Problems for Image Processing Applications
2020 International Conference on Systems, Signals and Image Processing (IWSSIP)(2020)
摘要
The development of techniques to automatic set up parameters in image processing methods based on variational approaches is cumbersome because the model sensitivity to parameters values is not known in general. In this paper, we address this issue through a data-driven inverse problem. Specifically, our methodology receives pairs (input image(s), desired result(s)) and seeks for the near optimum parameter vector through an inverse problem based on minimization scheme. The methodology is not restricted to a particular functional and it does not require large annotated data sets as input. Besides, methods based on partial differential equations (PDEs) can be also accommodated in our approach. We validate the methodology for calibrating parameters using as test-bed a variation of Mumford-Shah method. The obtained solutions using the parameters found in this paper are compared with literature results in order to show the efficiency of our technique.
更多查看译文
关键词
Parameters calibration,Variational methods,Inverse problems,Partial differential equations,Image processing
AI 理解论文
溯源树
样例
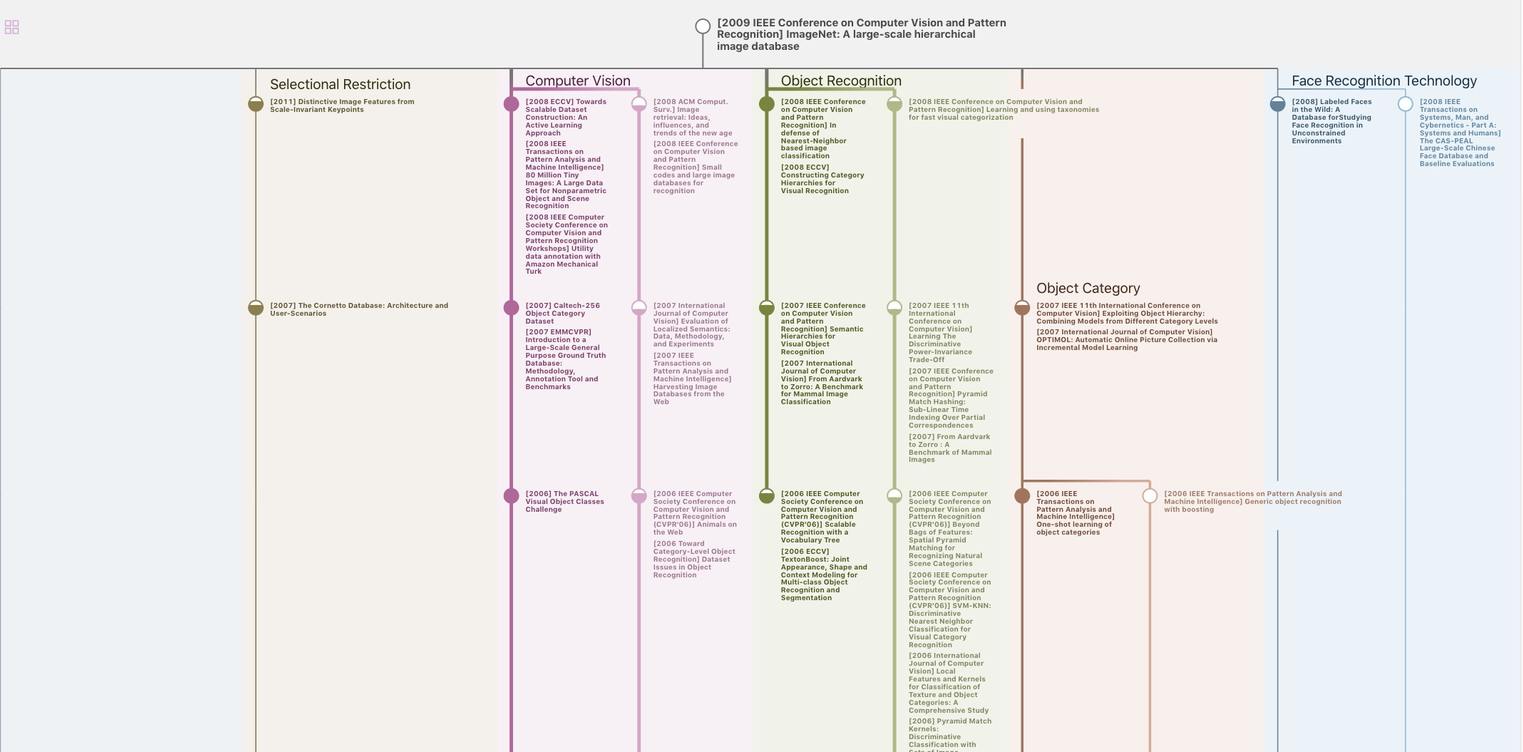
生成溯源树,研究论文发展脉络
Chat Paper
正在生成论文摘要