DeepSVG: A Hierarchical Generative Network for Vector Graphics Animation
NIPS 2020(2020)
摘要
Scalable Vector Graphics (SVG) are ubiquitous in modern 2D interfaces due to their ability to scale to different resolutions. However, despite the success of deep learning-based models applied to rasterized images, the problem of vector graphics representation learning and generation remains largely unexplored. In this work, we propose a novel hierarchical generative network, called DeepSVG, for complex SVG icons generation and interpolation. Our architecture effectively disentangles high-level shapes from the low-level commands that encode the shape itself. The network directly predicts a set of shapes in a non-autoregressive fashion. We introduce the task of complex SVG icons generation by releasing a new large-scale dataset along with an open-source library for SVG manipulation. We demonstrate that our network learns to accurately reconstruct diverse vector graphics, and can serve as a powerful animation tool by performing interpolations and other latent space operations. Our code is available at https://github.com/alexandre01/deepsvg.
更多查看译文
关键词
hierarchical generative network,animation
AI 理解论文
溯源树
样例
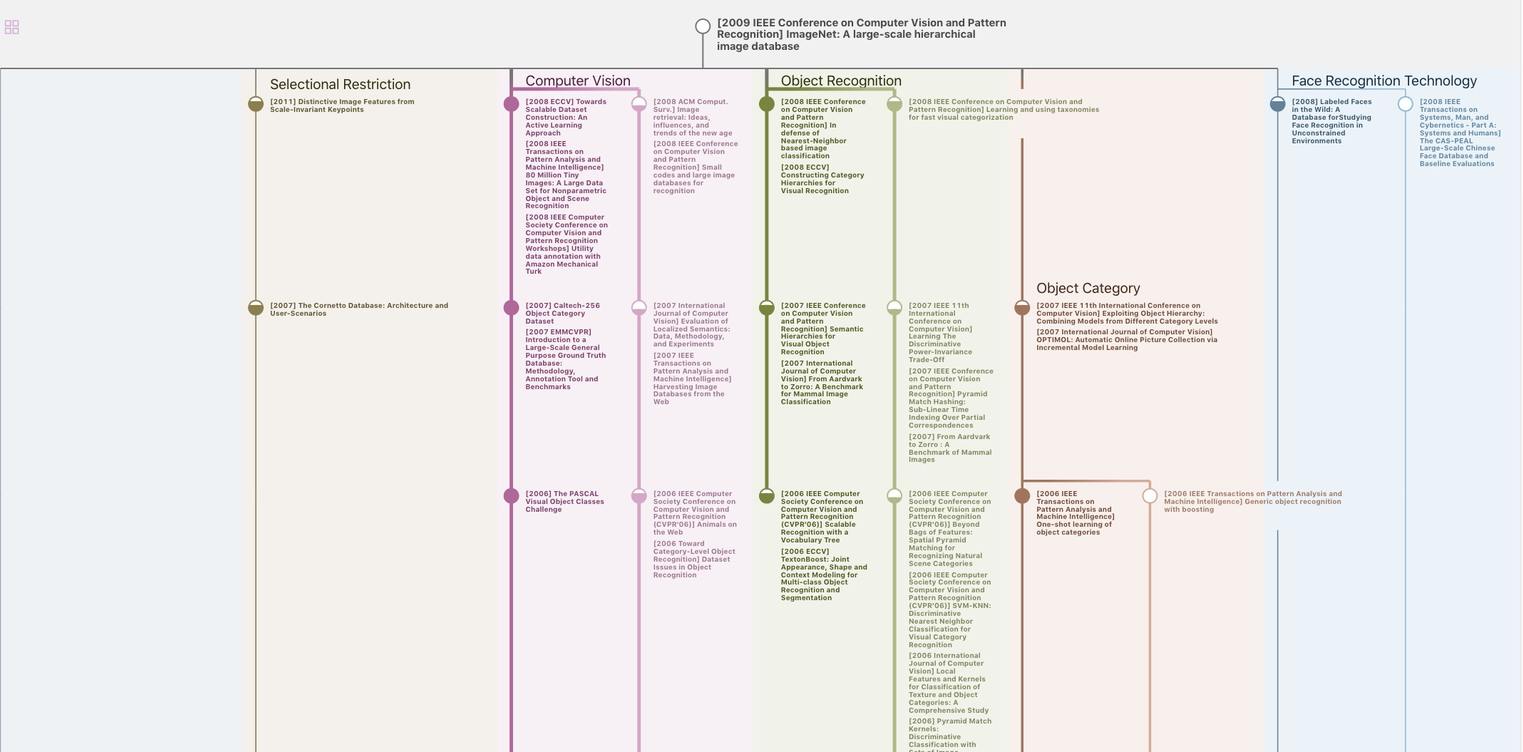
生成溯源树,研究论文发展脉络
Chat Paper
正在生成论文摘要