Computational Cognitive-Semantic Based Semantic Learning, Representation and Growth: A Perspective
2019 IEEE 18th International Conference on Cognitive Informatics & Cognitive Computing (ICCI*CC)(2019)
摘要
In this era of data-analytics, the unstructured text remains the main data format. The vector space model is commonly used in representing and modeling text semantics; however, it has some limitations. The main alternative for the vector space model is the graph model from graph theory. Then, the question is: On what basis should text semantics be modeled using graph modeling? Using semantic-graphs, cognitive-semantics tries to answer this question, as it models underlying mechanisms of our human cognition modules in learning, representing and expanding semantics. The fact that textual data is produced in the form of human natural language by human cognition skills means that a reverse-engineering methodology could be promising to extract back semantics from text. In this paper, we present a systematic perspective of the main computational graph-based cognitive-semantic models of human memory, that have been used for the semantic processing of unstructured text. The applications, strengths, and limitations of each model are described. Finally, open problems, future work and conclusions are presented.
更多查看译文
关键词
cognitive-semantics,semantic learning,semantic representation,cognitive computing,semantic memory
AI 理解论文
溯源树
样例
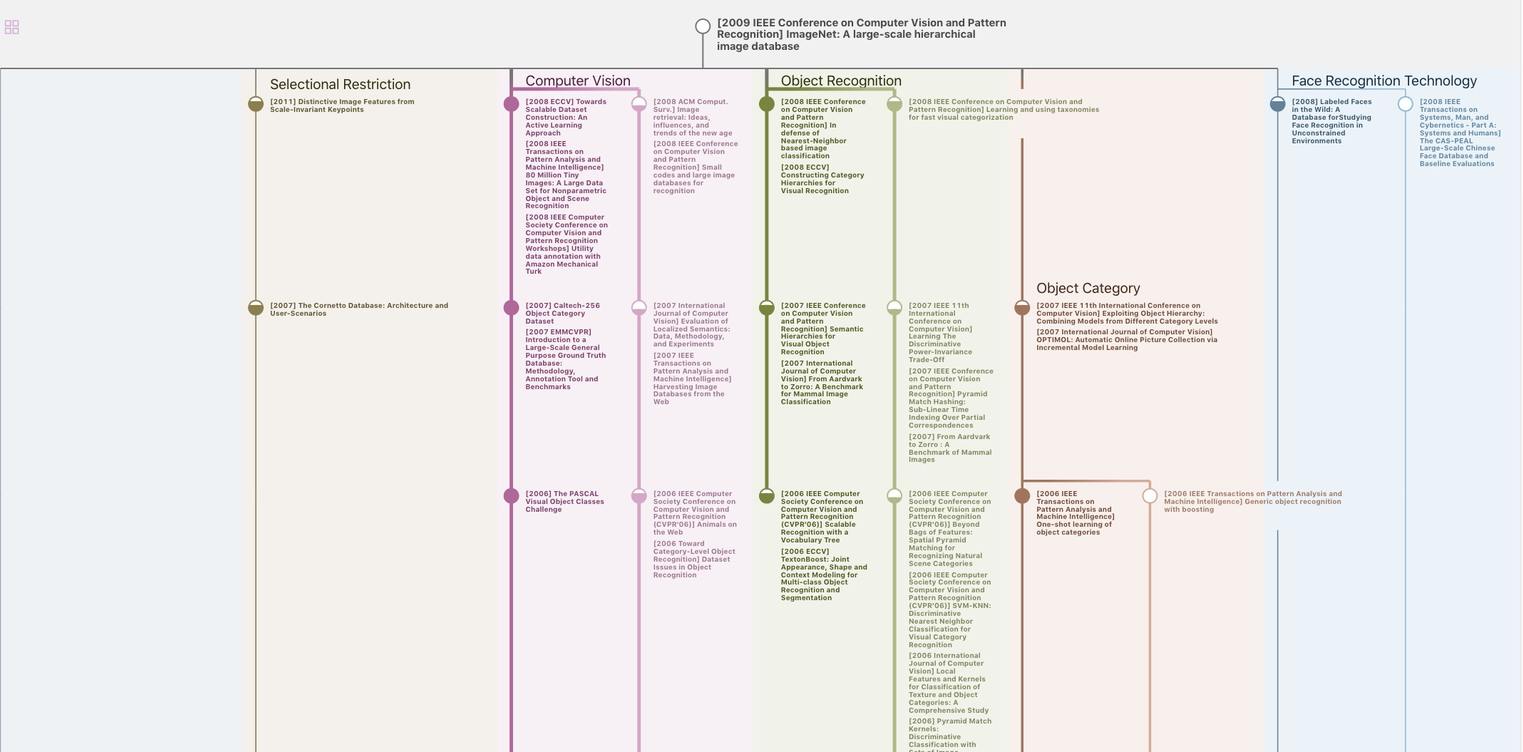
生成溯源树,研究论文发展脉络
Chat Paper
正在生成论文摘要