Machine Learning based Network Planning in Drone Aided Emergency Communications.
VTC Spring(2020)
摘要
Rapid deployment is crucial for building up drone aided emergency communications to ensure the coverage and service support after the disaster. The chaos in the post-disaster area, such as the number of ground users and scattered locations, makes difficult on the decision of drone deployment. In this paper, an unsupervised machine learning method is conducted for drone deployment in drone aided emergency communications. Considering the importance of sustainable services for drones, the drone deployment problem is formulated with the aim of minimizing the total power of drones with all users’ coverage while maintaining their rate requirements, with constraints on drones’ coverage area, capacity and limited power. The problem is solved by two steps. A modified k-means clustering algorithm is proposed to obtain the number of drones and an optimal altitude and minimum transmit power algorithm is then derived. Simulation results show that although the number of drones obtained by the modified algorithm is more than that of the original k-means algorithm, all users are served and the minimum power of drones is guaranteed by proposed algorithms.
更多查看译文
关键词
drone aided emergency communications,service support,unsupervised machine learning,drone deployment problem,network planning,post-disaster area,drones coverage area,k-means clustering,optimal altitude,minimum transmit power algorithm
AI 理解论文
溯源树
样例
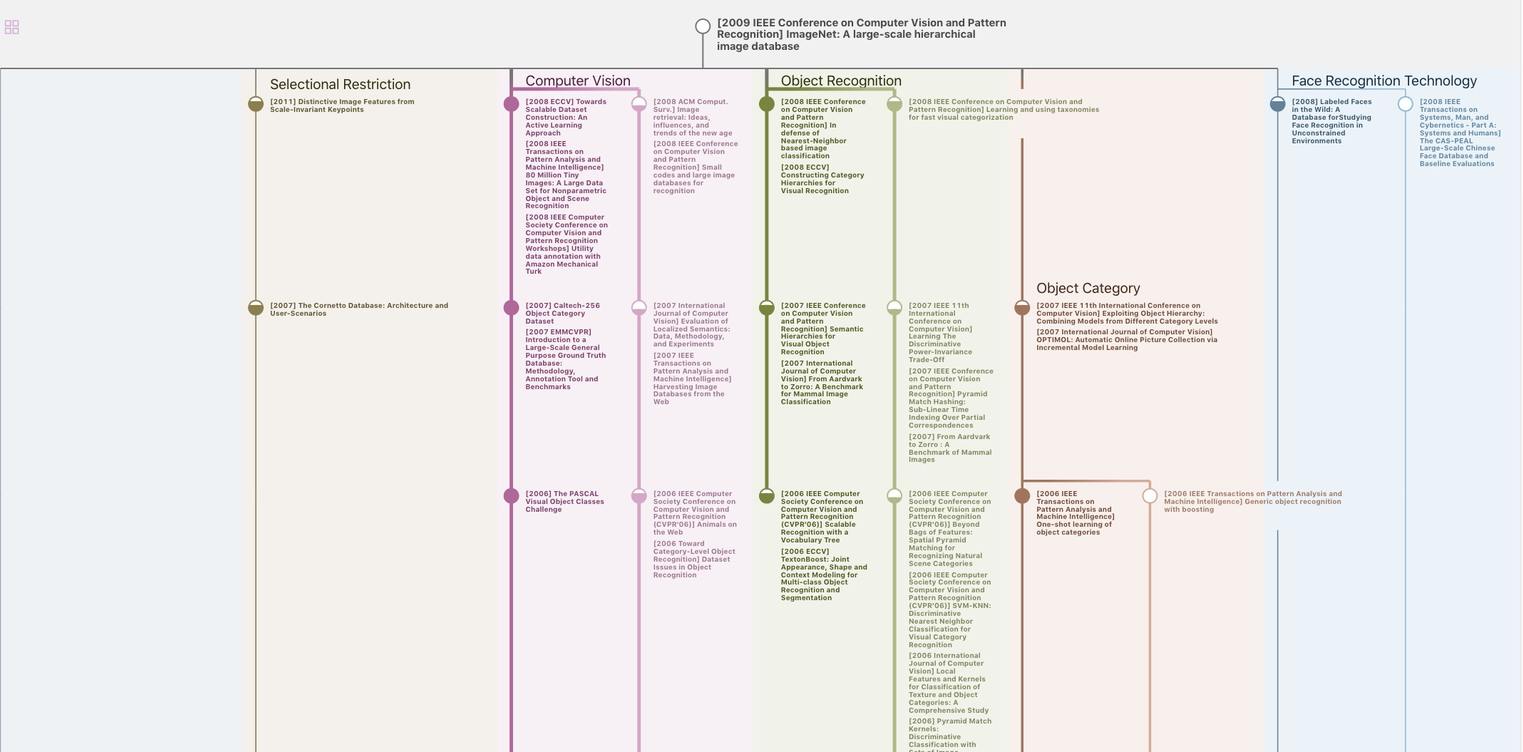
生成溯源树,研究论文发展脉络
Chat Paper
正在生成论文摘要