Neural Network Equalisation and Symbol Detection for 802.11p V2V Communication at 5.9GHz.
VTC Spring(2020)
摘要
Neural networks are shown to be a viable implementation for joint channel equalisation and symbol detection in a vehicular network. Experimental results using a hardware-in-the-loop approach at 5.9GHz validate the efficacy of the proposed implementation following the 802.11p parameters using orthogonal frequency division multiplexing (OFDM). Further results are obtained using a more spectrally efficient waveform, namely spectrally efficient frequency division multiplexing (SEFDM), to show a trade-off between loss of orthogonality, and therefore bit-error rate (BER) performance, versus increased spectral efficiency to enable higher data rates or the ability to service more users. SEFDM is tested with compression factors ranging from 20% up to 60% bandwidth compression. The results show the neural network is able to achieve an acceptable BER performance in a highway non-line-of-sight (NLOS) channel which is a well established harsh and dynamic vehicular channel. This is further validated via measurements of the error vector magnitude.
更多查看译文
关键词
Machine Learning,OFDM,SEFDM,V2V
AI 理解论文
溯源树
样例
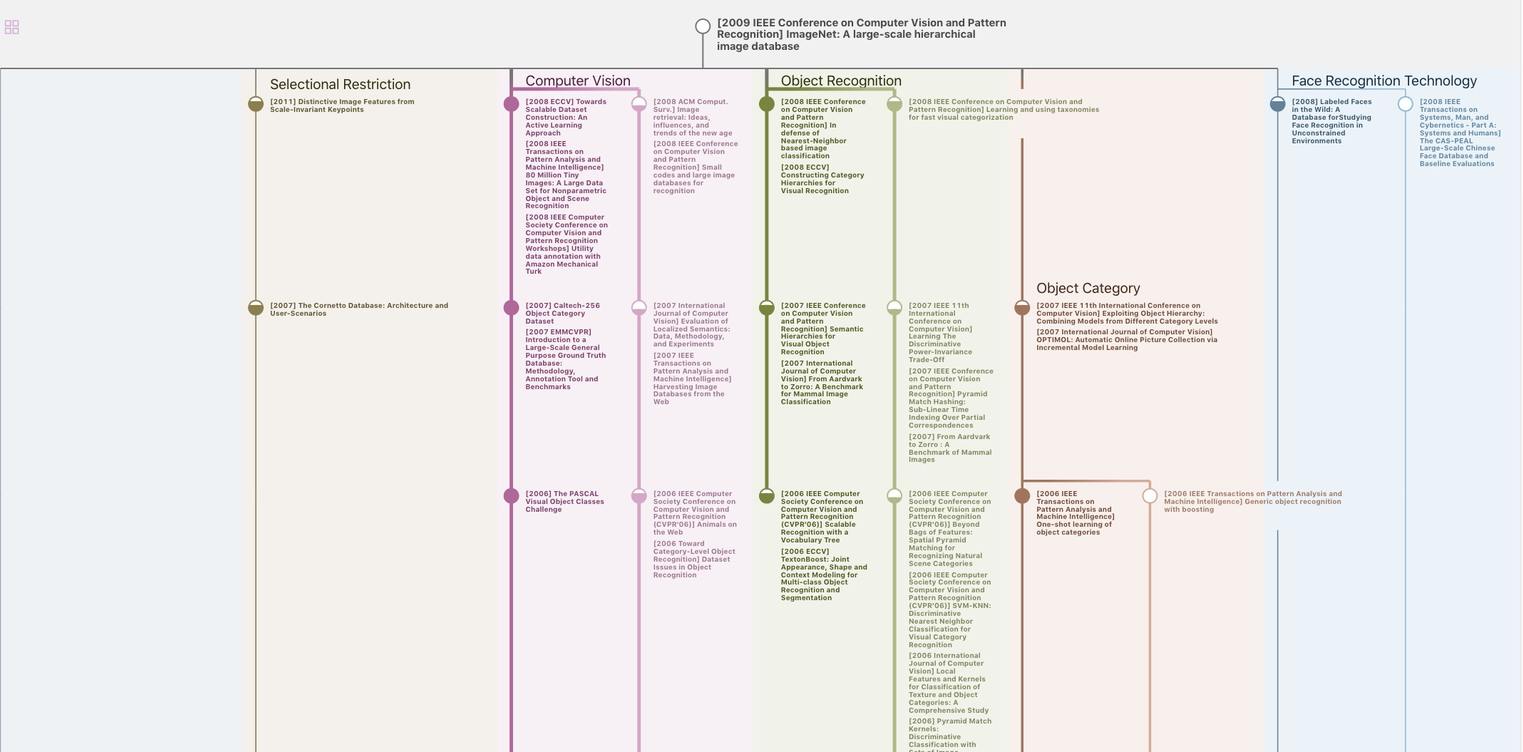
生成溯源树,研究论文发展脉络
Chat Paper
正在生成论文摘要