Approximate Symbolic Explanation for Neural Network Enabled Water-Filling Power Allocation
2020 IEEE 91st Vehicular Technology Conference (VTC2020-Spring)(2020)
摘要
Water-filling (WF) is a well-established iterative solution to optimal power allocation in parallel fading channels. Slow iterative search can be impractical for allocating power to a large number of OFDM sub-channels. Neural networks (NN) can transform the iterative WF threshold search process into a direct high-dimensional mapping from channel gain to transmit power solution. Our results show that the NN can perform very well (error 0.05%) and can be shown to be indeed performing approximate WF power allocation. However, there is no guarantee on the NN is mapping between channel states and power output. Here, we attempt to explain the NN power allocation solution via the Meijer G-function as a general explainable symbolic mapping. Our early results indicate that whilst the Meijer G-function has universal representation potential, its large search space means finding the best symbolic representation is challenging.
更多查看译文
关键词
machine learning,deep learning,XAI,wireless
AI 理解论文
溯源树
样例
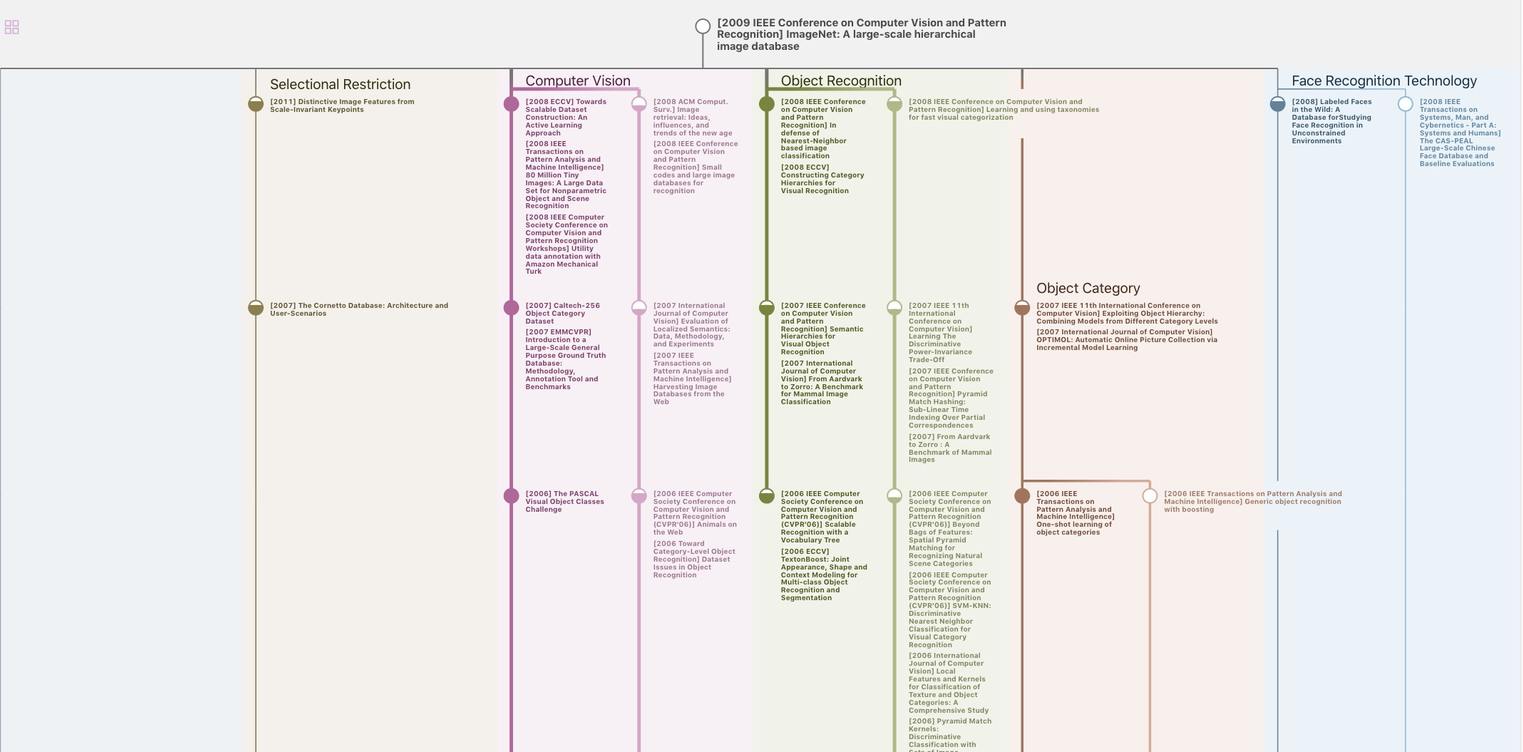
生成溯源树,研究论文发展脉络
Chat Paper
正在生成论文摘要