Learning Infinite-Horizon Average-Reward Mdps With Linear Function Approximation
24TH INTERNATIONAL CONFERENCE ON ARTIFICIAL INTELLIGENCE AND STATISTICS (AISTATS)(2021)
摘要
We develop several new algorithms for learning Markov Decision Processes in an infinite-horizon average-reward setting with linear function approximation. Using the optimism principle and assuming that the MDP has a linear structure, we first propose a computationally inefficient algorithm with optimal (O) over tilde(root T) regret and another computationally efficient variant with (O) over tilde (T-3/4) regret, where T is the number of interactions. Next, taking inspiration from adversarial linear bandits, we develop yet another efficient algorithm with (O) over tilde(root T) regret under a different set of assumptions, improving the best existing result by Hao et al. (2021) with (O) over tilde (T-2/3) regret. Moreover, we draw a connection between this algorithm and the Natural Policy Gradient algorithm proposed by Kakade (2002), and show that our analysis improves the sample complexity bound recently given by Agarwal et al. (2020).
更多查看译文
AI 理解论文
溯源树
样例
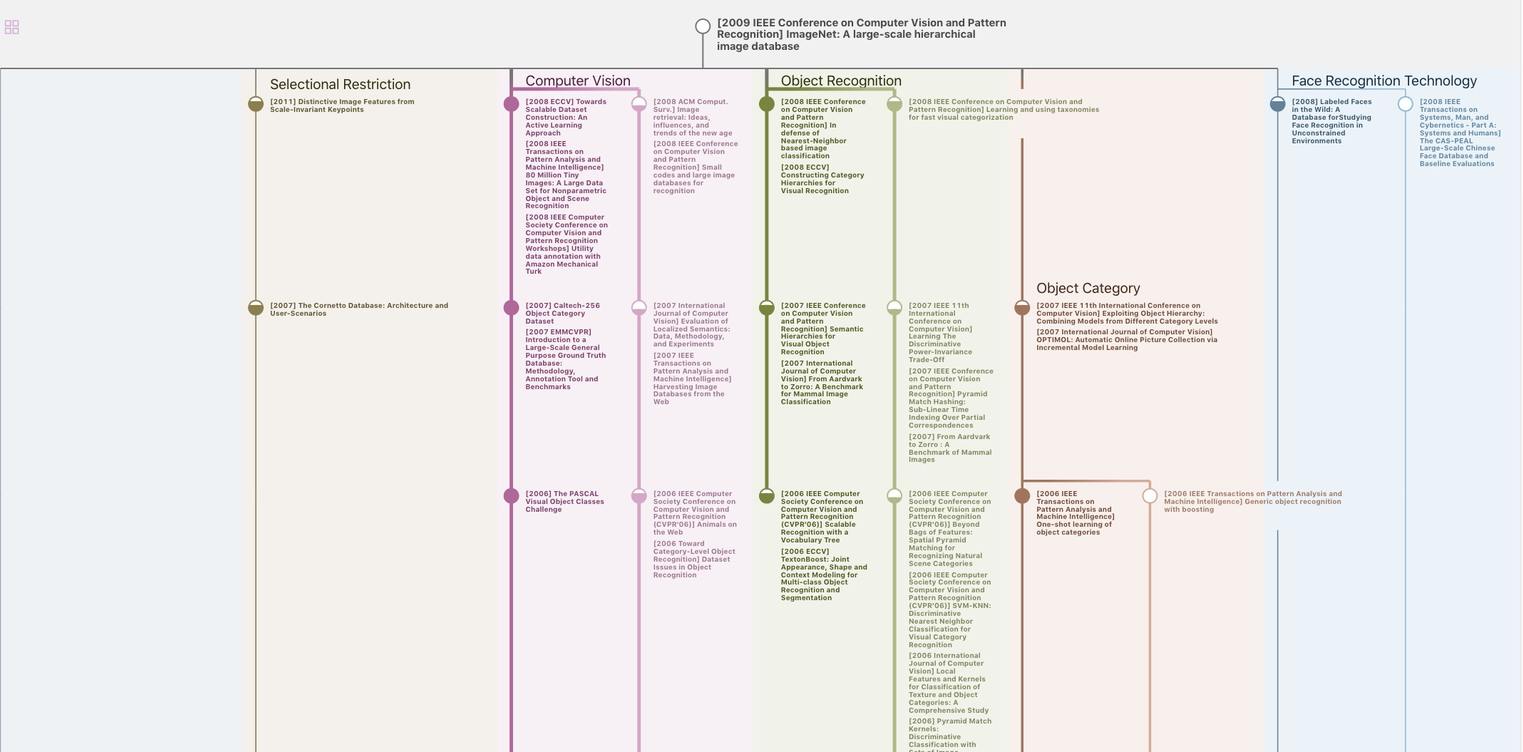
生成溯源树,研究论文发展脉络
Chat Paper
正在生成论文摘要