Clustering multivariate functional data in group-specific functional subspaces
Computational Statistics(2020)
摘要
With the emergence of numerical sensors in many aspects of everyday life, there is an increasing need in analyzing multivariate functional data. This work focuses on the clustering of such functional data, in order to ease their modeling and understanding. To this end, a novel clustering technique for multivariate functional data is presented. This method is based on a functional latent mixture model which fits the data into group-specific functional subspaces through a multivariate functional principal component analysis. A family of parsimonious models is obtained by constraining model parameters within and between groups. An Expectation Maximization algorithm is proposed for model inference and the choice of hyper-parameters is addressed through model selection. Numerical experiments on simulated datasets highlight the good performance of the proposed methodology compared to existing works. This algorithm is then applied to the analysis of the pollution in French cities for 1 year.
更多查看译文
关键词
Multivariate functional curves,Multivariate functional principal component analysis,Model-based clustering,EM algorithm
AI 理解论文
溯源树
样例
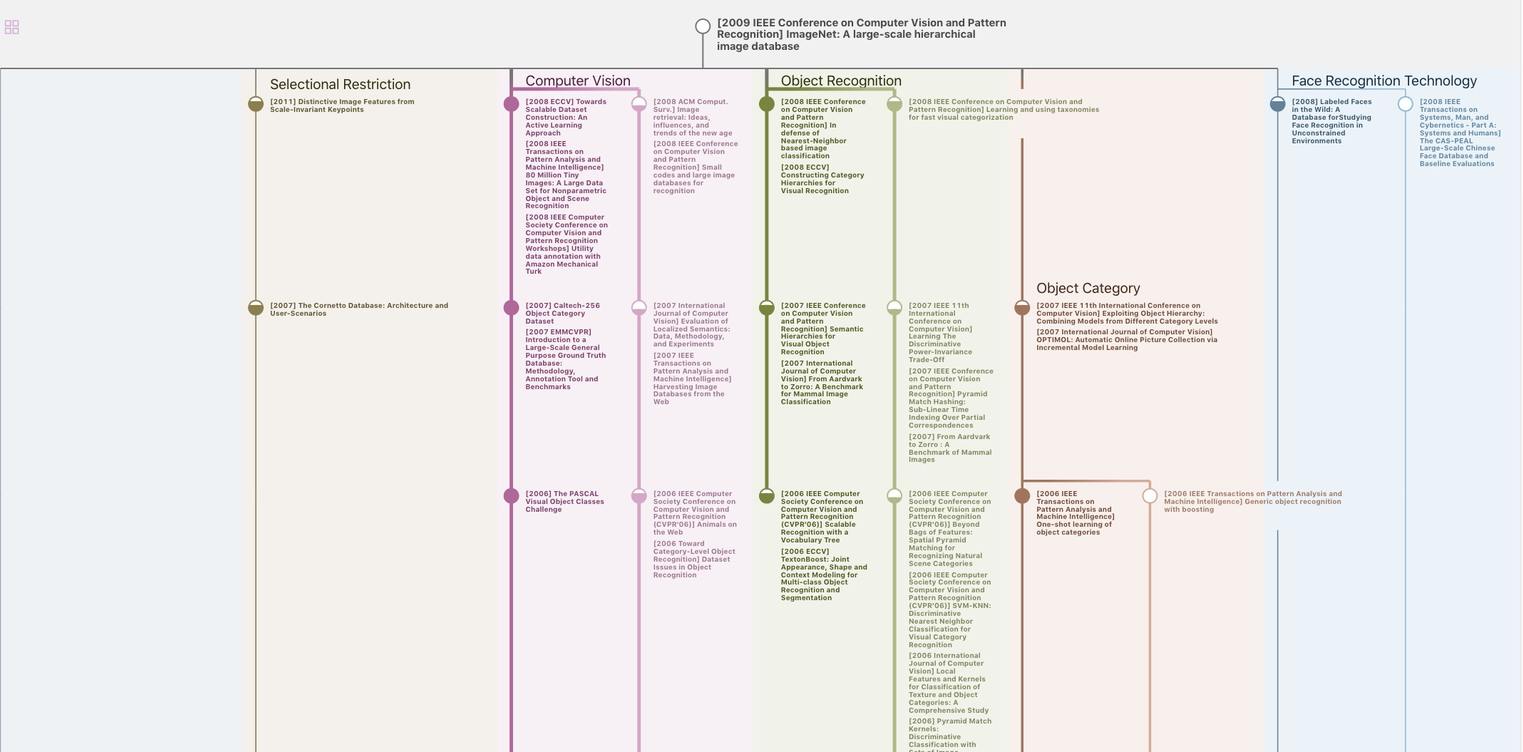
生成溯源树,研究论文发展脉络
Chat Paper
正在生成论文摘要