Activity recognition using wearable sensors for tracking the elderly
USER MODELING AND USER-ADAPTED INTERACTION(2020)
摘要
population group that is often overlooked in the recent revolution of self-tracking is the group of older people. This growing proportion of the general population is often faced with increasing health issues and discomfort. In order to come up with lifestyle advice towards the elderly, we need the ability to quantify their lifestyle, before and after an intervention. This research focuses on the task of activity recognition (AR) from accelerometer data. With that aim, we collect a substantial labelled dataset of older individuals wearing multiple devices simultaneously and performing a strict protocol of 16 activities (the GOTOV dataset, N=28 ). Using this dataset, we trained Random Forest AR models, under varying sensor set-ups and levels of activity description granularity. The model that combines ankle and wrist accelerometers (GENEActiv) produced the best results (accuracy >80% ) for 16-class classification. At the same time, when additional physiological information is used, the accuracy increased ( >85% ). To further investigate the role of granularity in our predictions, we developed the LARA algorithm, which uses a hierarchical ontology that captures prior biological knowledge to increase or decrease the level of activity granularity (merge classes). As a result, a 12-class model in which the different paces of walking were merged showed a performance above 93% . Testing this 12-class model in labelled free-living pilot data, the mean balanced accuracy appeared to be reasonably high, while using the LARA algorithm, we show that a 7-class model (lying down, sitting, standing, household, walking, cycling, jumping) was optimal for accuracy and granularity. Finally, we demonstrate the use of the latter model in unlabelled free-living data from a larger lifestyle intervention study. In this paper, we make the validation data as well as the derived prediction models available to the community.
更多查看译文
关键词
Activity recognition,Activity ontology,Ageing population,Public dataset,Random forest,Sensor selection,Wearables
AI 理解论文
溯源树
样例
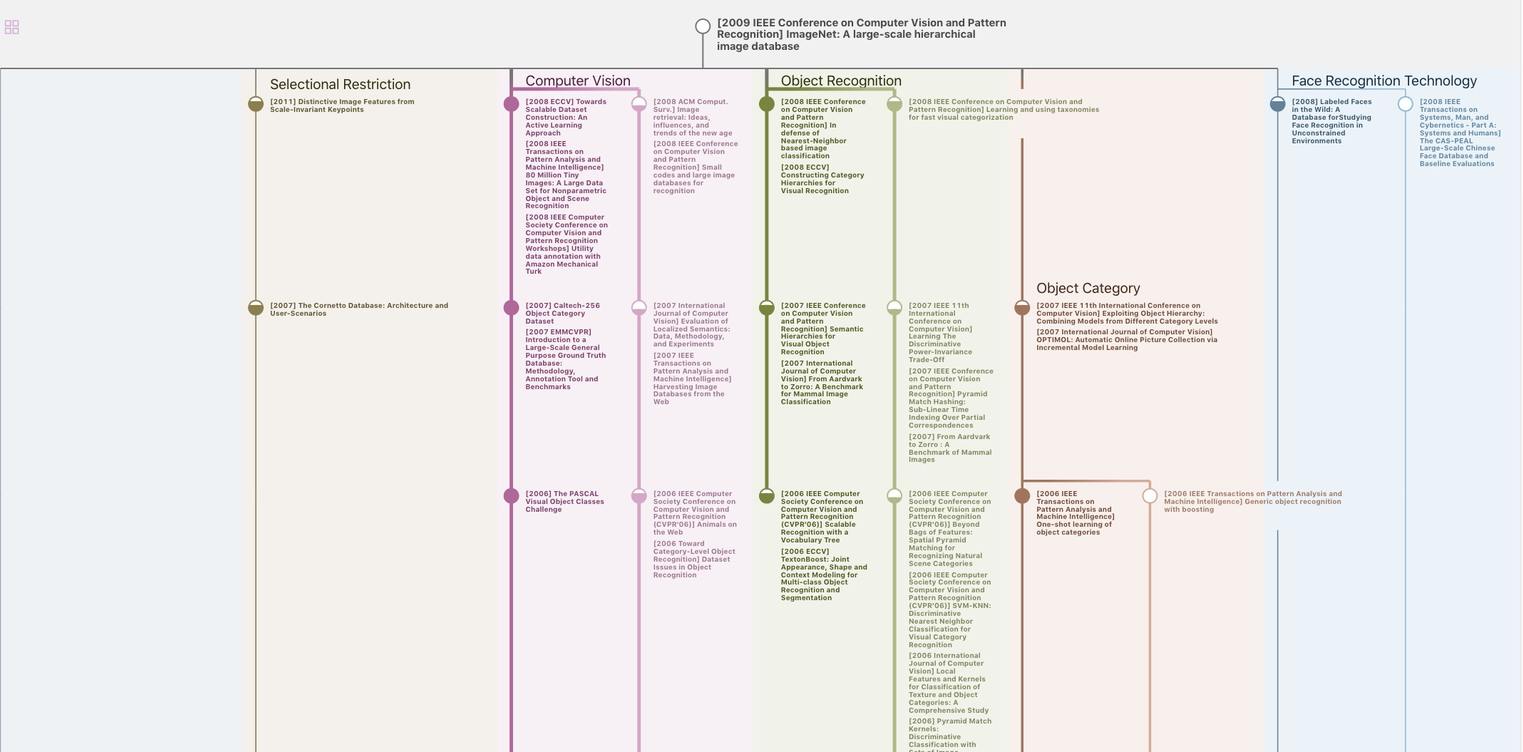
生成溯源树,研究论文发展脉络
Chat Paper
正在生成论文摘要