Recognition of Crop Diseases Based on Depthwise Separable Convolution in Edge Computing.
SENSORS(2020)
摘要
The original pattern recognition and classification of crop diseases needs to collect a large amount of data in the field and send them next to a computer server through the network for recognition and classification. This method usually takes a long time, is expensive, and is difficult to carry out for timely monitoring of crop diseases, causing delays to diagnosis and treatment. With the emergence of edge computing, one can attempt to deploy the pattern recognition algorithm to the farmland environment and monitor the growth of crops promptly. However, due to the limited resources of the edge device, the original deep recognition model is challenging to apply. Due to this, in this article, a recognition model based on a depthwise separable convolutional neural network (DSCNN) is proposed, which operation particularities include a significant reduction in the number of parameters and the amount of computation, making the proposed design well suited for the edge. To show its effectiveness, simulation results are compared with the main convolution neural network (CNN) models LeNet and Visual Geometry Group Network (VGGNet) and show that, based on high recognition accuracy, the recognition time of the proposed model is reduced by 80.9% and 94.4%, respectively. Given its fast recognition speed and high recognition accuracy, the model is suitable for the real-time monitoring and recognition of crop diseases by provisioning remote embedded equipment and deploying the proposed model using edge computing.
更多查看译文
关键词
depthwise separable convolution neural network,recognition of crop diseases,Visual Geometry Group (VGG) network model
AI 理解论文
溯源树
样例
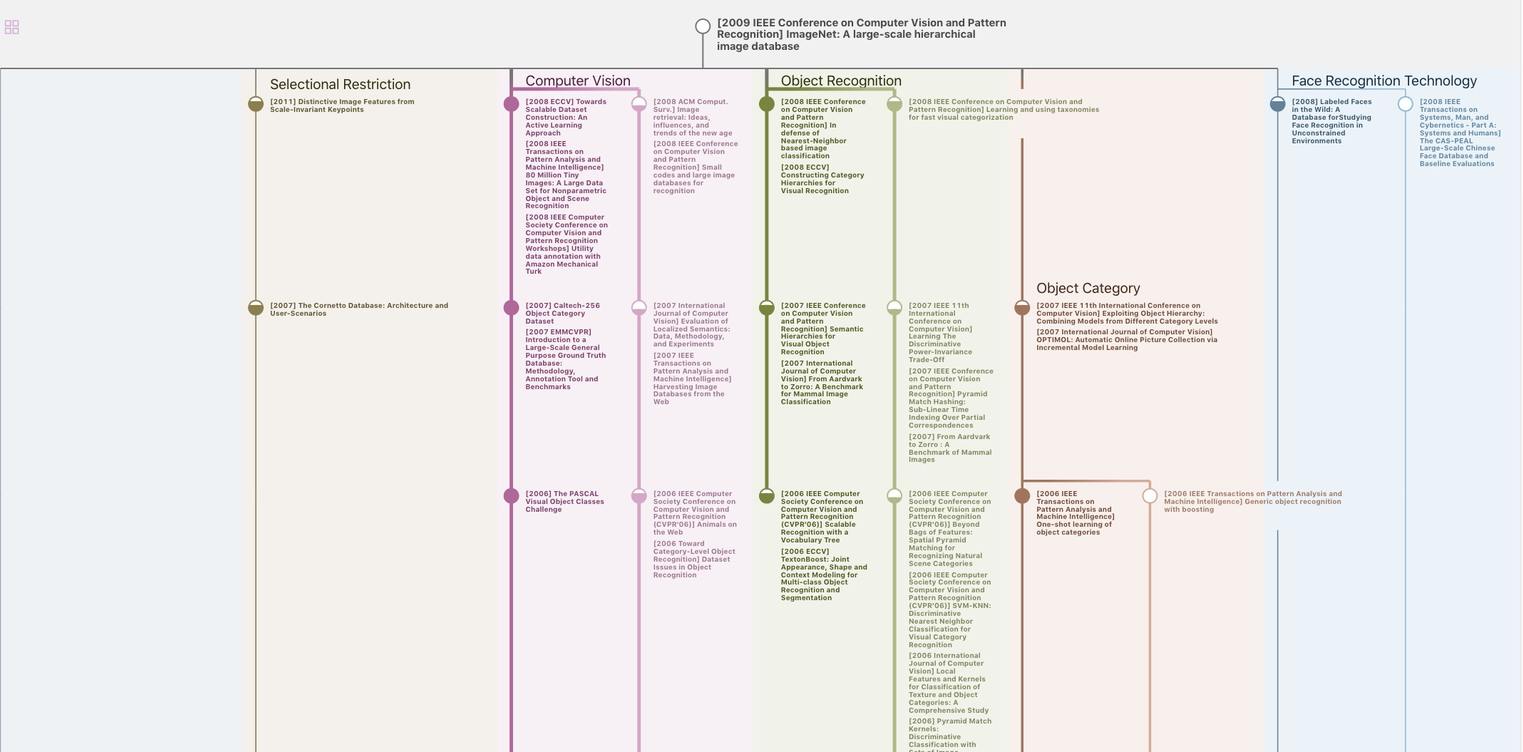
生成溯源树,研究论文发展脉络
Chat Paper
正在生成论文摘要