Multiobjective And Categorical Global Optimization Of Photonic Structures Based On Resnet Generative Neural Networks
NANOPHOTONICS(2021)
摘要
We show that deep generative neural networks, based on global optimization networks (GLOnets), can be configured to perform the multiobjective and categorical global optimization of photonic devices. A residual network scheme enables GLOnets to evolve from a deep architecture, which is required to properly search the full design space early in the optimization process, to a shallow network that generates a narrow distribution of globally optimal devices. As a proof-of-concept demonstration, we adapt our method to design thin-film stacks consisting of multiple material types. Benchmarks with known globally optimized antireflection structures indicate that GLOnets can find the global optimum with orders of magnitude faster speeds compared to conventional algorithms. We also demonstrate the utility of our method in complex design tasks with its application to incandescent light filters. These results indicate that advanced concepts in deep learning can push the capabilities of inverse design algorithms for photonics.
更多查看译文
关键词
categorical optimization, global optimization, multiobjective optimization, neural networks, thin-film stack
AI 理解论文
溯源树
样例
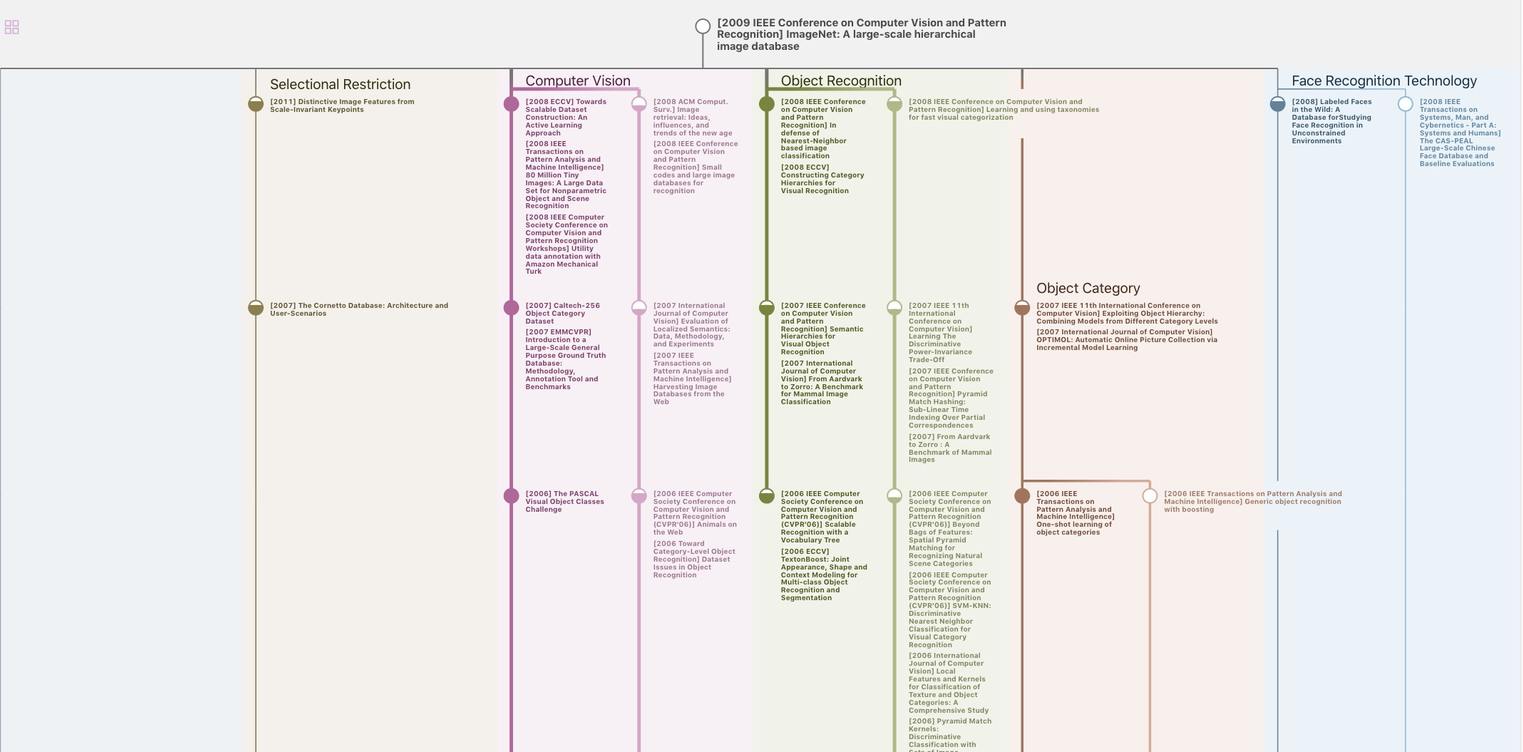
生成溯源树,研究论文发展脉络
Chat Paper
正在生成论文摘要