Computation Offloading With Reinforcement Learning in D2D-MEC Network
2020 International Wireless Communications and Mobile Computing (IWCMC)(2020)
摘要
With the deployment of compute-intensive applications on mobile devices, some work aims to support these applications by enhancing mobile edge computing (MEC) using device-to-device (D2D) communication technology. Different from previous work, we investigate the energy consumption optimization of MEC assisted by idle user equipment (UE) in a mobile environment when combining the two technologies, and propose an optimization method for continuous time. In this paper, we build a D2D-MEC model with user mobility. To optimize the processing decision to save the energy consumption in continuous time under this model, we define long-term costs and formulate the problem of minimizing long-term costs as a markov decision process (MDP) problem. The complex MDP problem is decomposed into two sub-problems, where the explicit cost is minimized first and then the long-term cost. Due to the uncertainty of the environment caused by the mobility of the UE and the high dimensionality of the environmental information, we use the reinforcement learning method based on the neural network approximation to minimize the long-term cost.
更多查看译文
关键词
Task analysis,Device-to-device communication,Energy consumption,Servers,Learning (artificial intelligence),Optimization,Computational modeling
AI 理解论文
溯源树
样例
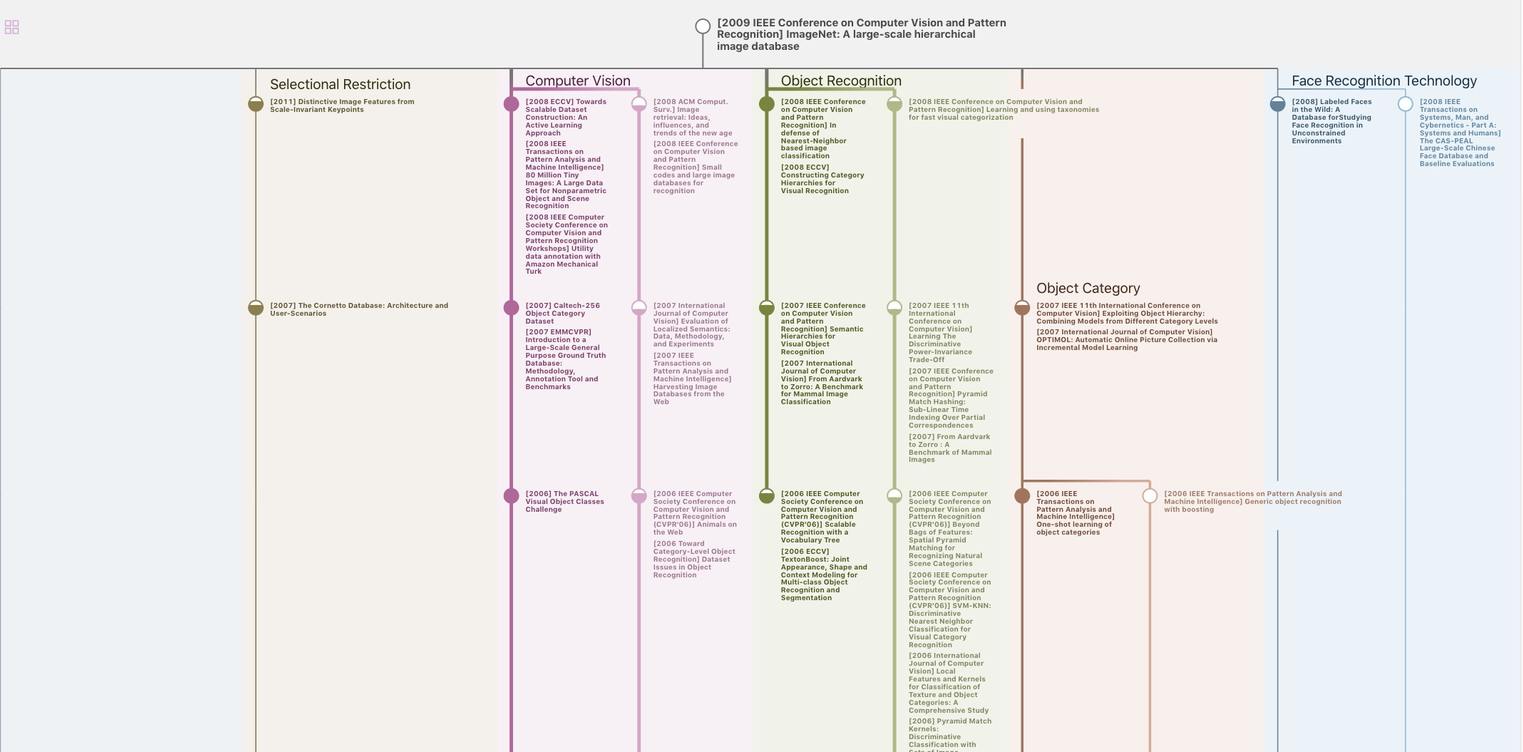
生成溯源树,研究论文发展脉络
Chat Paper
正在生成论文摘要