Analytic continuation of the self-energy via Machine Learning techniques
arxiv(2020)
摘要
We develop a novel analytic continuation method for self-energies on the Matsubara domain as computed by quantum Monte Carlo simulations within dynamical mean field theory (QMC+DMFT). Unlike a maximum entropy (maxEn) procedure employed for the last thirty years, our approach is based on a machine learning (ML) technique in combination with the iterative perturbative theory impurity solver of the dynamical mean field theory self-consistent process (IPT+DMFT). The input and output training datasets for ML are simultaneously obtained from IPT+DMFT calculations on Matsubara and real frequency domains, respectively. The QMC+DMFT self-energy on real frequencies is determined from the -- usually noisy -- input QMC+DMFT self-energy on the Matsubara domain and the trained ML kernel. Our approach is free from both, bias of ML training datasets and from fitting parameters present in the maxEn method. We demonstrate the efficiency of the method on the testbed frustrated Hubbard model on the square lattice.
更多查看译文
关键词
analytic continuation,machine learning,self-energy
AI 理解论文
溯源树
样例
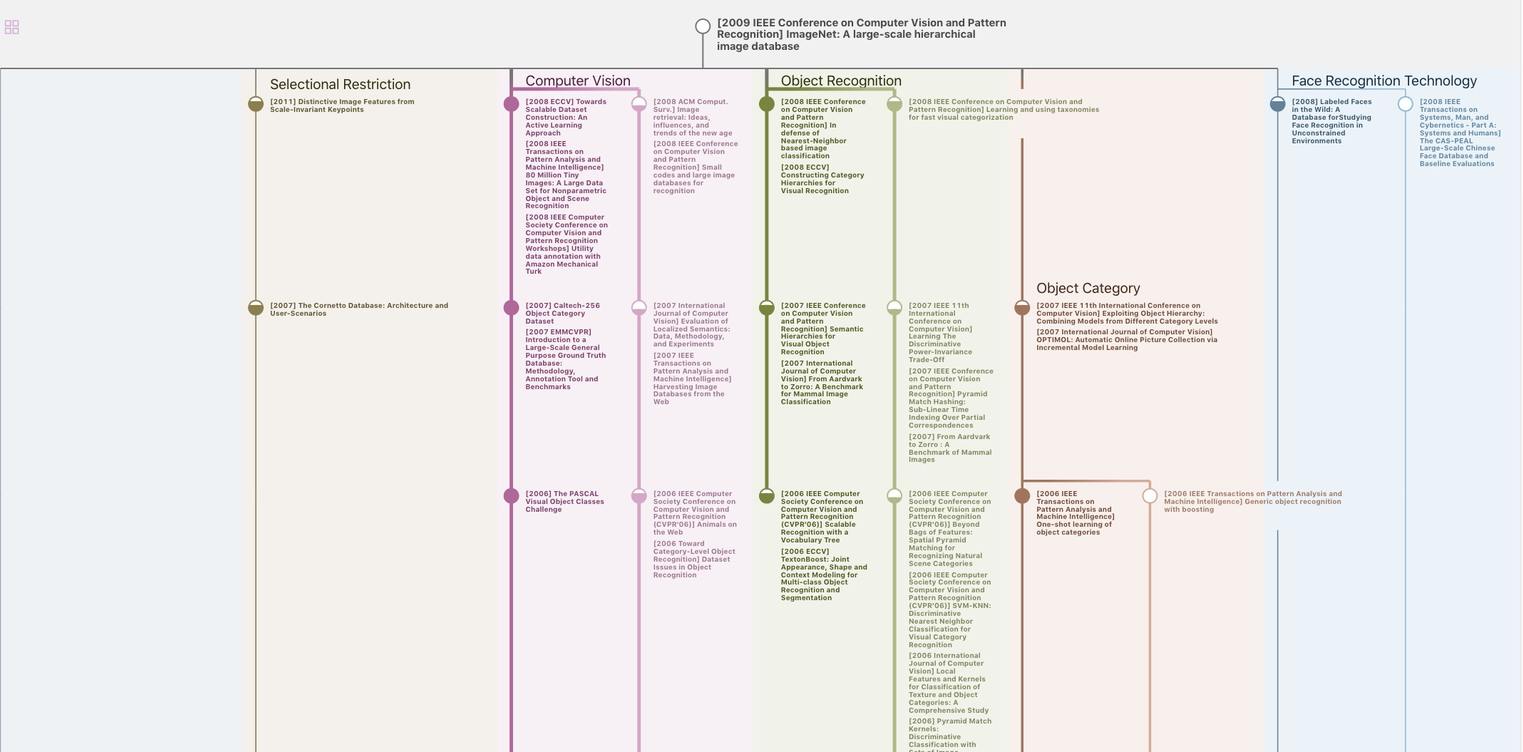
生成溯源树,研究论文发展脉络
Chat Paper
正在生成论文摘要