Outcome model free causal inference with ultra-high dimensional covariates
arxiv(2021)
摘要
Causal inference has been increasingly reliant on observational studies with rich covariate information. To build tractable causal models, including the propensity score models, it is imperative to first extract important features from high dimensional data. Unlike the familiar task of variable selection for prediction modeling, our feature selection procedure aims to control for confounding while maintaining efficiency in the resulting causal effect estimate. Previous empirical studies imply that one should aim to include all predictors of the outcome, rather than the treatment, in the propensity score model. In this paper, we formalize this intuition through rigorous proofs, and propose the causal ball screening for selecting these variables from modern ultra-high dimensional data sets. A distinctive feature of our proposal is that we do not require any modeling on the outcome regression, thus providing robustness against misspecification of the functional form or violation of smoothness conditions. Our theoretical analyses show that the proposed procedure enjoys a number of oracle properties including model selection consistency, normality and efficiency. Synthetic and real data analyses show that our proposal performs favorably with existing methods in a range of realistic settings.
更多查看译文
关键词
free causal inference,causal inference,model,ultra-high
AI 理解论文
溯源树
样例
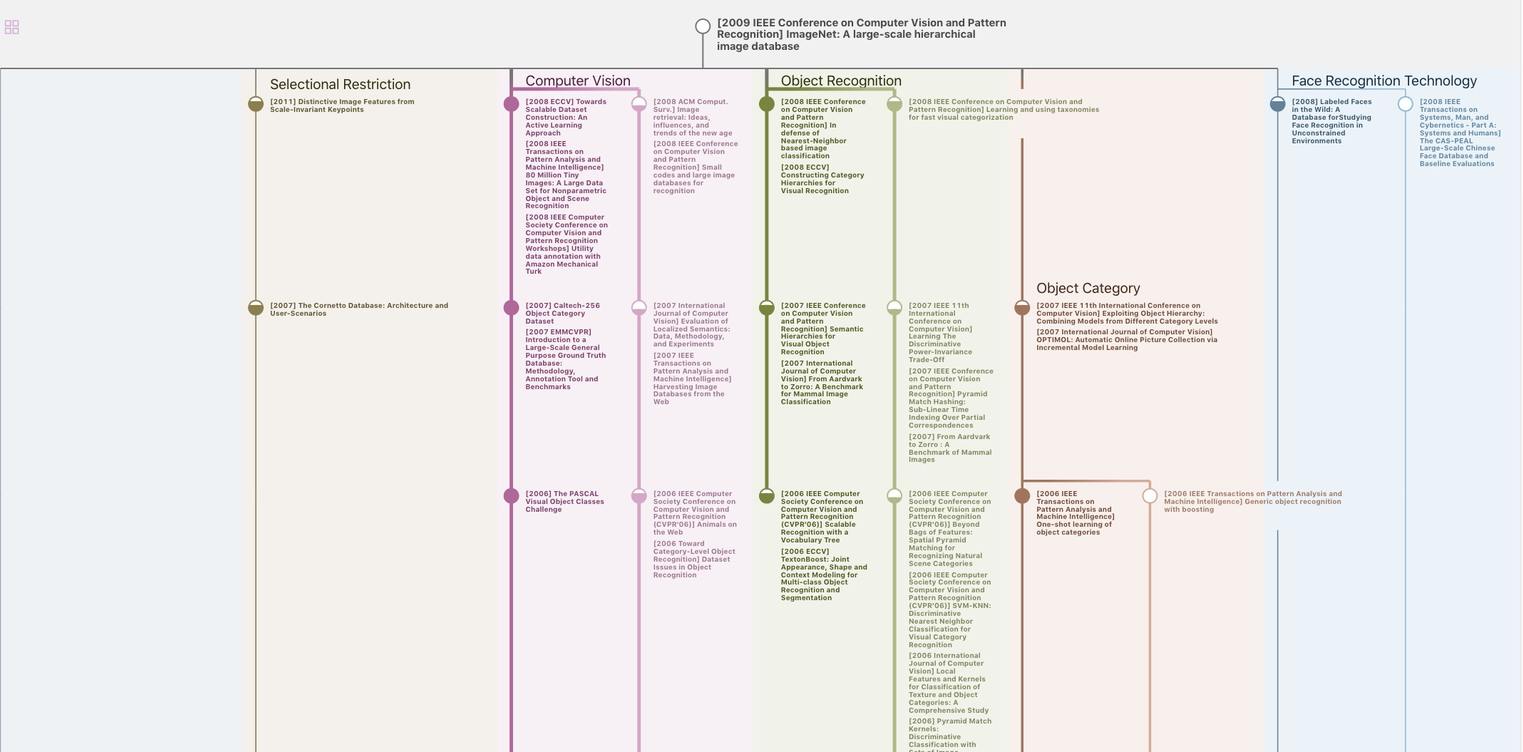
生成溯源树,研究论文发展脉络
Chat Paper
正在生成论文摘要