Bitrap: Bi-Directional Pedestrian Trajectory Prediction With Multi-Modal Goal Estimation
IEEE ROBOTICS AND AUTOMATION LETTERS(2021)
摘要
Pedestrian trajectory prediction is an essential task in robotic applications such as autonomous driving and robot navigation. State-of-the-art trajectory predictors use a conditional variational autoencoder (CVAE) with recurrent neural networks (RNNs) to encode observed trajectories and decode multi-modal future trajectories. This process can suffer from accumulated errors over long prediction horizons (>= 2 seconds). This letter presents BiTraP, a goal-conditioned hi-directional multi-modal trajectory prediction method based on the CVAE. BiTraP estimates the goal (end-point) of trajectories and introduces a novel bidirectional decoder to improve longer-term trajectory prediction accuracy. Extensive experiments show that BiTraP generalizes to both first-person view (FPV) and bird's-eye view (BEV) scenarios and outperforms state-of-the-art results by similar to 10-50%. We also show that different choices of non-parametric versus parametric target models in the CVAE directly influence the predicted multi-modal trajectory distributions. These results provide guidance on trajectory predictor design for robotic applications such as collision avoidance and navigation systems. Our code is available at: bups://github.com/untautobots/bidireaction-trajectory-prediction.
更多查看译文
关键词
Computer vision for automation, human and humanoid motion analysis and synthesis, deep learning methods, multi-modal trajectory prediction, goal-conditioned prediction
AI 理解论文
溯源树
样例
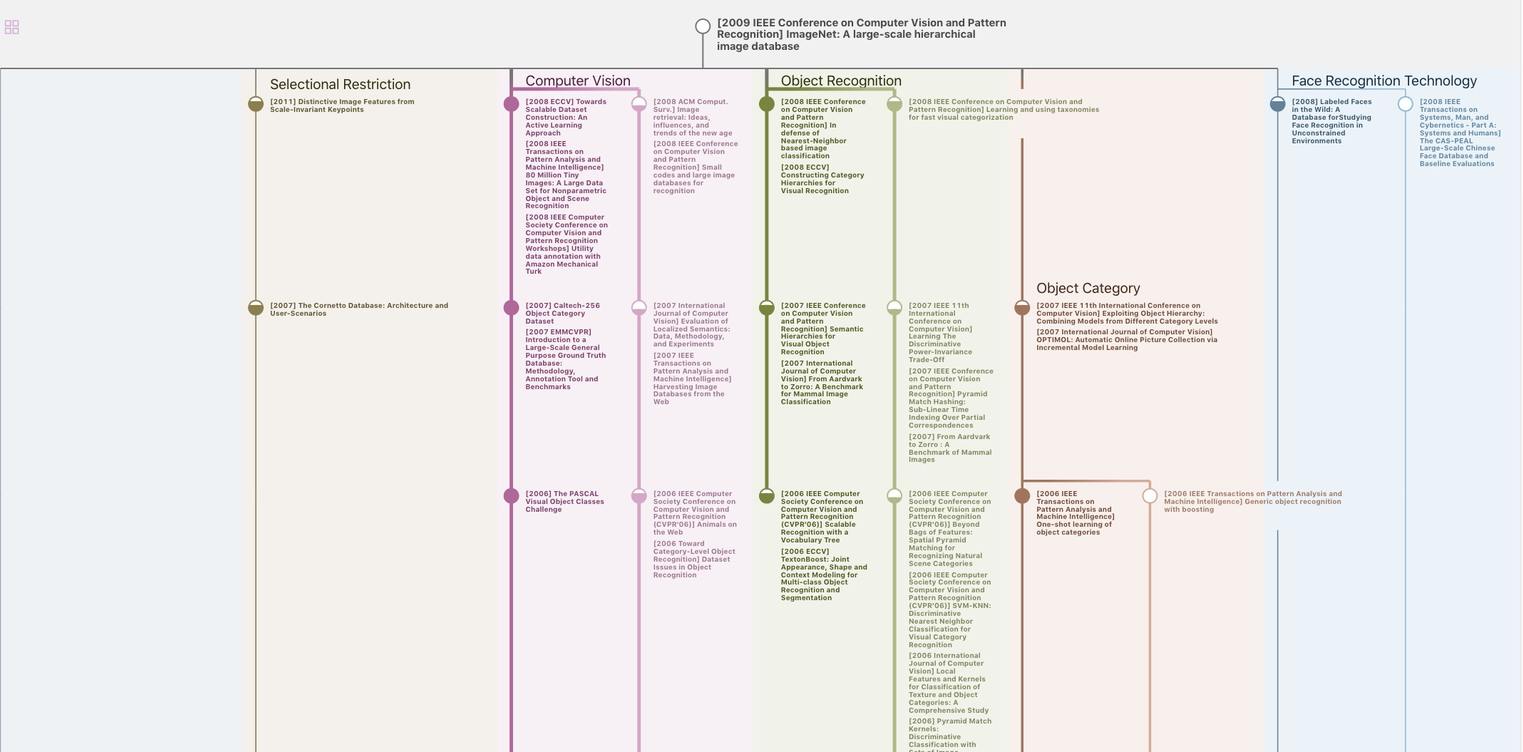
生成溯源树,研究论文发展脉络
Chat Paper
正在生成论文摘要