Efficient Miss Ratio Curve Computation for Heterogeneous Content Popularity.
USENIX Annual Technical Conference(2020)
Abstract
The Miss Ratio Curve (MRC) represents a fundamental tool for cache performance profiling. Approximate methods based on sampling provide a low-complexity solution for MRC construction. Nevertheless, in this paper we show that, in case of content with a large variance in popularity, the approximate MRC may be highly sensitive to the set of sampled content. We study in detail the impact of content popularity heterogeneity on the accuracy of the approximate MRC. We observe that few, highly popular, items may cause large error at the head of the reconstructed MRC. From these observations, we design a new approach for building an approximate MRC, where we combine an exact portion of the MRC with an approximate one built from samples. Results for different real-world traces show that our algorithm computes MRC with an error up to 10 times smaller than state-of-the-art methods based on sampling, with similar computational and space overhead.
MoreTranslated text
Key words
heterogeneous content popularity,ratio
AI Read Science
Must-Reading Tree
Example
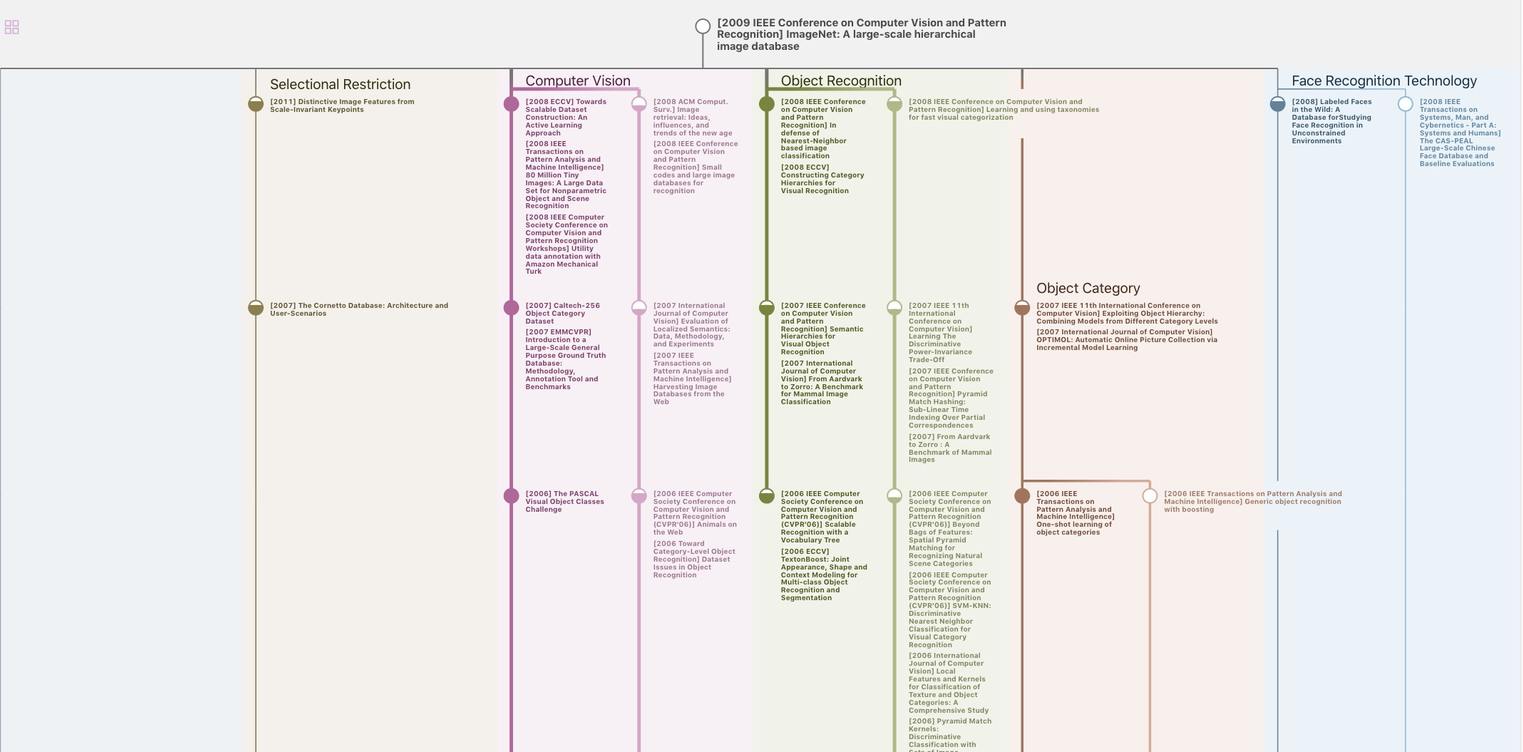
Generate MRT to find the research sequence of this paper
Chat Paper
Summary is being generated by the instructions you defined